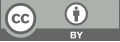
A study of advances in machine learning-based electronic design automation
- 1 Ocean University of China
* Author to whom correspondence should be addressed.
Abstract
The electronic design automation (EDA) is a convenient tool for designing integrated circuits (IC), which is employed extensively both in academic and engineering. The design of integrated circuits is conducted in accordance with a defined design flow, commonly referred to as the chip design flow, which can be divided into two distinct parts, the front-end design and the back-end design. Following a long period of evolution, the chip design flow of EDA has been gradually improved. Besides, it achieved some accomplishments during this period. However, with the growing demand of ICs, especially Very Large-Scale Integration Circuit (VLSI), the existing EDA is not adequate for the requirement. In addition, the EDA technology has been so developed that it is relatively less flexible. In this context, concerns about the future of EDA have recently emerged. In response to this challenge, research has mentioned that machine learning methods (ML methods) can improve the functionality of EDA. The ML method covers most of steps of EDA’s design flow, especially back-end design. The machine learning-based electronic design automation is still in its infancy, which is presented with a multitude of challenges. Therefore, the paper explores the development of EDA by reviewing and organizing the related literature, and summarizes the application of ML methods in EDA, thereby providing the future development trend of ML-based EDA.
Keywords
Electronic Design Automation, Machine Learning, Computer Aided Design, Trend, IC Design.
[1]. Curiac, C.D. and Doboli, A. (2022) Combining informetrics and trend analysis to understand past and current directions in electronic design automation. Scientometrics, 127: 5661–5689.
[2]. Huang, G.Y., Hu, J.B., et al. (2021) Machine Learning for Electronic Design Automation: A Survey. ACM Trans. Des. Autom. Electron. Syst. 26(5), 1-46.
[3]. Chai Z.M., Zhao Y.X., et al. (2022) CircuitNet: an open-source dataset for machine learning applications in electronic design automation (EDA). Sci China Inf Sci, 65(12): 227401.
[4]. Marques-Silva, J.P. and Sakallah, K.A. (2000) Boolean satisfiability in electronic design automation. Proceedings of the 37th Annual Design Automation Conference, 675-680.
[5]. MacMillen, D., et al. (2000) An industrial view of electronic design automation. IEEE transactions on computer-aided design of integrated circuits and systems, 19(12): 1428-1448.
[6]. Sangiovanni-Vincentelli, A.L. (2003) The tides of EDA. IEEE Design & Test of Computers, 20(6): 59-75.
[7]. Gubbi, K.I., et al. (2022) Survey of Machine Learning for Electronic Design Automation. GLSVLSI’22: Proceedings of the Great Lakes Symposium on VLSI 2022, 513-518.
[8]. Oetjens, J-H., et al. (2014) Safety evaluation of automotive electronics using virtual prototypes: State of the art and research challenges. Proceedings of the 51st annual design automation conference,1-6.
[9]. Lin, Y.B., Gao, X.H., et al. (2021) Machine learning assisted backend design method for digital integrated circuits. Micro/nano Electronics and Intelligent Manufacturing, 3(2): 11-20.
[10]. Marinova, G.I., et al. (2021) Challenges and opportunities for semiconductor and electronic design automation industry in post-Covid-19 years. IOP Conference Series: Materials Science and Engineering, 1208(1): 1-6.
[11]. Hamolia, V. and Melnyk, V. (2021) A Survey of Machine Learning Methods and Applications in Electronic Design Automation. 2021 11th International conference on advanced computer information technologies (ACIT), 757-760.
[12]. Chen, T.H., et al. (2022) Machine learning in advanced IC design: A methodological survey. IEEE Design & Test, 40(1): 17-33.
Cite this article
Li,J. (2024). A study of advances in machine learning-based electronic design automation. Applied and Computational Engineering,102,96-101.
Data availability
The datasets used and/or analyzed during the current study will be available from the authors upon reasonable request.
Disclaimer/Publisher's Note
The statements, opinions and data contained in all publications are solely those of the individual author(s) and contributor(s) and not of EWA Publishing and/or the editor(s). EWA Publishing and/or the editor(s) disclaim responsibility for any injury to people or property resulting from any ideas, methods, instructions or products referred to in the content.
About volume
Volume title: Proceedings of the 2nd International Conference on Machine Learning and Automation
© 2024 by the author(s). Licensee EWA Publishing, Oxford, UK. This article is an open access article distributed under the terms and
conditions of the Creative Commons Attribution (CC BY) license. Authors who
publish this series agree to the following terms:
1. Authors retain copyright and grant the series right of first publication with the work simultaneously licensed under a Creative Commons
Attribution License that allows others to share the work with an acknowledgment of the work's authorship and initial publication in this
series.
2. Authors are able to enter into separate, additional contractual arrangements for the non-exclusive distribution of the series's published
version of the work (e.g., post it to an institutional repository or publish it in a book), with an acknowledgment of its initial
publication in this series.
3. Authors are permitted and encouraged to post their work online (e.g., in institutional repositories or on their website) prior to and
during the submission process, as it can lead to productive exchanges, as well as earlier and greater citation of published work (See
Open access policy for details).