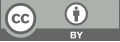
Comparison of the Robustness of Multimodal Models and Unimodal Models under Text-based Adversarial Attacks
- 1 School of Foreign Languages and Literature, Wuhan University, Wuhan, China
* Author to whom correspondence should be addressed.
Abstract
With the popularization of artificial intelligence technology, adversarial attacks have become a major challenge in the field of machine learning. This paper explores the robustness of multimodal and unimodal models under textual adversarial attacks, and probes to understand their differences and commonalities. By comparing and analyzing the performance of the CLIP multimodal model and BERT unimodal model under different textual datasets, it is pointed out that the multimodal model does not perform better than the unimodal model under unimodal adversarial attack when the multimodal fusion advantage cannot be reflected. On the contrary, the CLIP model, which is a multimodal model, exhibits larger robustness fluctuations similar to the BERT model under single-modal adversarial attacks. The advantages of multimodal models do not automatically translate into better robustness in all scenarios but need to be optimized for specific tasks and adversarial strategies, and the multimodal models do not have better accuracy than the unimodal models without task-specific pre-training. Both exhibit significant robustness fluctuations in the face of textual adversarial attacks. The research in this paper provides research value and further research directions for future studies
Keywords
Multimodal model, Unimodal model, Adversarial attack, Robustness.
[1]. Ian, J. G., Jonathon, S., and Christian, S. (2015). Explaining and Harnessing Adversarial Examples, 2015. arXiv:1412.6572v3.
[2]. Aleksander, M., Aleksandar, M., Ludwig, S., Dimitris, T., and Adrian, V. (2018). Towards Deep Learning Models Resistant to Adversarial Attacks, arXiv:1706.06083v4.
[3]. Yin, Z. Y., Ye, M. C. et al., (2023). VLATTACK: Multimodal Adversarial Attacks on Vision-Language Tasks via Pre-trained Models, 2023. In Advances in Neural Information Processing Systems,36 (pp. 52936-52956).
[4]. Emma, Z., Quan, Z. S., Ahoud, A., Chen, L. (2020). Adversarial Attacks on Deep-learning Models in Natural Language Processing: A Survey, 2020. In ACM Transactions on Intelligent Systems and Technology (TIST), Volume 11, Issue 3 (pp. 1-41)
[5]. Alec, R., Wook, K., Chris, H., Aditya, R., Gabriel, G., Sandhini, A., Girish, S., Amanda, A., Pamela, M., Jack, C., Gretchen, K., Ilya, S. (2021). Learning Transferable Visual Models From Natural Language Supervision, 2021. In Proceedings of the 38th International Conference on Machine Learning, PMLR 139:8748-8763.
[6]. Jacob, D., Ming, W. C., Kenton, L., Kristina, T. (2019). BERT: Pre-training of Deep Bidirectional Transformers for Language Understanding, 2019. arXiv:1810.04805.
[7]. Andrew, L. M., Raymond, E. D., Peter, T. P., Dan, H., Andrew, Y., and Christopher, P. (2011). Learning Word Vectors for Sentiment Analysis, 2011. In Proceedings of the 49th Annual Meeting of the Association for Computational Linguistics: Human Language Technologies (pp. 142-150).
[8]. Xiang, Z., Jun, Z., Yann, L. (2015). Character-level Convolutional Networks for Text Classification, 2015. In Advances in Neural Information Processing Systems 28 (NIPS 2015).
[9]. Jin, D., Jin, Z., Zhou, J. T., & Szolovits, P. (2020). Is BERT Really Robust? A Strong Baseline for Natural Language Attack on Text Classification and Entailment, 2020. In Proceedings of the AAAI Conference on Artificial Intelligence, 34(05), 8018-8025.
[10]. Wei, W., Du, T., Matt, F. (2020). What Makes Training Multi-Modal Classification Networks Hard, 2020. In Proceedings of the IEEE/CVF Conference on Computer Vision and Pattern Recognition (CVPR) (pp. 12695-12705).
Cite this article
Liu,Y. (2024). Comparison of the Robustness of Multimodal Models and Unimodal Models under Text-based Adversarial Attacks. Applied and Computational Engineering,103,117-122.
Data availability
The datasets used and/or analyzed during the current study will be available from the authors upon reasonable request.
Disclaimer/Publisher's Note
The statements, opinions and data contained in all publications are solely those of the individual author(s) and contributor(s) and not of EWA Publishing and/or the editor(s). EWA Publishing and/or the editor(s) disclaim responsibility for any injury to people or property resulting from any ideas, methods, instructions or products referred to in the content.
About volume
Volume title: Proceedings of the 2nd International Conference on Machine Learning and Automation
© 2024 by the author(s). Licensee EWA Publishing, Oxford, UK. This article is an open access article distributed under the terms and
conditions of the Creative Commons Attribution (CC BY) license. Authors who
publish this series agree to the following terms:
1. Authors retain copyright and grant the series right of first publication with the work simultaneously licensed under a Creative Commons
Attribution License that allows others to share the work with an acknowledgment of the work's authorship and initial publication in this
series.
2. Authors are able to enter into separate, additional contractual arrangements for the non-exclusive distribution of the series's published
version of the work (e.g., post it to an institutional repository or publish it in a book), with an acknowledgment of its initial
publication in this series.
3. Authors are permitted and encouraged to post their work online (e.g., in institutional repositories or on their website) prior to and
during the submission process, as it can lead to productive exchanges, as well as earlier and greater citation of published work (See
Open access policy for details).