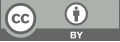
Design of a Gomoku AI Based on the Alpha-Beta Pruning Search Algorithm
- 1 School of Big Data and Software, Chongqing University, Chongqing, 401331, China
* Author to whom correspondence should be addressed.
Abstract
This paper presents the implementation of a Gomoku AI based on the alpha-beta pruning search algorithm and the Negamax algorithm. Gomoku, a traditional board game known for its strategic depth, poses significant challenges in AI development due to the exponential increase in possible moves. The AI leverages the computational efficiency of Alpha-Beta Pruning, which enhances the Negamax algorithm by reducing the number of nodes that need to be evaluated in the game tree. This combination allows for faster decision-making without compromising accuracy. Additionally, a value evaluation function is used to assess board states and guide the AI in selecting optimal moves. In the results the performance of the AI was tested through simulations, demonstrating great performance in move selection and computational efficiency compared to traditional methods. The paper also explores potential improvements, including the integration of reinforcement learning (RL) techniques to further enhance the AI's adaptability and strategic decision-making capabilities.
Keywords
Gomoku, Negamax algorithm, alpha-beta pruning.
[1]. Sravya M, Sanjan V and Tejashwini B 2018 Implementation of sequential and parallel alpha-beta pruning algorithm. International Journal of Innovations in Engineering and Technology, 7(8), 98-104.
[2]. Aashrit G and Anita S 2023 Chess Board: Performance of Alpha–Beta Pruning in Reducing Node Count of Minimax Tree. Intelligent Technologies and Robotics, 661-671.
[3]. Judea P 2022 The Solution for the Branching Factor of the Alpha-Beta Pruning Algorithm and its Optimality. Probabilistic and Causal Inference: The Works of Judea Pearl, 91-102.
[4]. Shevtekar S S, Malpe M and Bhaila M 2022 Analysis of Game Tree Search Algorithms Using Minimax Algorithm and Alpha-Beta Pruning. International Journal of Scientific Research in Computer Science Engineering and Information Technology, 328-333.
[5]. Singhal S P and Sridevi M 2019 Comparative study of performance of parallel alpha Beta Pruning for different architectures. 2019 IEEE 9th International Conference on Advanced Computing (IACC), 115-119.
[6]. Naoyuki S and Kokolo I 2016 Three types of forward pruning techniques to apply the alpha beta algorithm to turn-based strategy games. 2016 IEEE Conference on Computational Intelligence and Games (CIG), 1-6.
[7]. Che Z H and Lv F2024 Design and implementation of Pentoku game based on Alpha-Beta pruning algorithm. Computer programming skills and maintenance, 131-133.
[8]. Liang W, et al. 2023 Mastering Gomoku with AlphaZero: A Study in Advanced AI Game Strategy. Sage Science Review of Applied Machine Learning, 6(11), 32-43.
[9]. Li X, He S, Wu L, Chen D and Zhao Y 2020 A game model for Gomoku based on deep learning and monte carlo tree search. Lecture Notes in Electrical Engineering, 88-97.
[10]. Jim X 2016 The Evolution of Computing: AlphaGo. Computing in Science & Engineering, 18, 4-7.
Cite this article
Xu,Y. (2024). Design of a Gomoku AI Based on the Alpha-Beta Pruning Search Algorithm. Applied and Computational Engineering,94,49-56.
Data availability
The datasets used and/or analyzed during the current study will be available from the authors upon reasonable request.
Disclaimer/Publisher's Note
The statements, opinions and data contained in all publications are solely those of the individual author(s) and contributor(s) and not of EWA Publishing and/or the editor(s). EWA Publishing and/or the editor(s) disclaim responsibility for any injury to people or property resulting from any ideas, methods, instructions or products referred to in the content.
About volume
Volume title: Proceedings of CONF-MLA 2024 Workshop: Securing the Future: Empowering Cyber Defense with Machine Learning and Deep Learning
© 2024 by the author(s). Licensee EWA Publishing, Oxford, UK. This article is an open access article distributed under the terms and
conditions of the Creative Commons Attribution (CC BY) license. Authors who
publish this series agree to the following terms:
1. Authors retain copyright and grant the series right of first publication with the work simultaneously licensed under a Creative Commons
Attribution License that allows others to share the work with an acknowledgment of the work's authorship and initial publication in this
series.
2. Authors are able to enter into separate, additional contractual arrangements for the non-exclusive distribution of the series's published
version of the work (e.g., post it to an institutional repository or publish it in a book), with an acknowledgment of its initial
publication in this series.
3. Authors are permitted and encouraged to post their work online (e.g., in institutional repositories or on their website) prior to and
during the submission process, as it can lead to productive exchanges, as well as earlier and greater citation of published work (See
Open access policy for details).