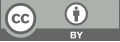
COVID-19 CT Image Segmentation Based on Modified U-Net
- 1 Shandong University of Science and Technology, 579 Qianwangang Road, Huangdao District, Qingdao, Shandong, China
* Author to whom correspondence should be addressed.
Abstract
This paper presents a COVID-19 CT image segmentation method based on a modified U-Net model. In the study and treatment of COVID-19, the segmentation of CT images is crucial for understanding the virus's impact on lung tissues. Traditional image segmentation methods have limitations when dealing with the complex lung lesions caused by COVID-19. Therefore, we optimized the U-Net model's structure and introduced an efficient loss function along with image enhancement techniques to improve segmentation accuracy and computational efficiency. Experiments were conducted on the Medseg and Radiopaedia datasets. The results demonstrate that the modified U-Net model outperforms traditional methods in terms of segmentation accuracy and computational efficiency. Additionally, we discuss the advantages and limitations of the model and propose directions for future research.
Keywords
COVID-19, CT Image Segmentation, U-Net Deep Learning, Medical Image Processing, Loss Function Optimization.
[1]. Y. Guo, Y. Wang, K. Meng, and Z. Zhu, “Otsu Multi-Threshold Image Segmentation Based on Adaptive Double-Mutation Differential Evolution,” Biomimetics, vol. 8, no. 5, Art. no. 5, Sep. 2023, doi: 10.3390/biomimetics8050418.
[2]. J. Zhang, W. Wang, and J. Wang, “Edge Detection in Colored Images Using Parallel CNNs and Social Spider Optimization,” Electronics, vol. 13, no. 17, Art. no. 17, Jan. 2024, doi: 10.3390/electronics13173540.
[3]. M. Leena Silvoster and R. M. S. Kumar, “Segmentation of Images Using Watershed and MSER: A State-of-the-Art Review,” in Recent Advances in Intelligent Systems and Smart Applications, M. Al-Emran, K. Shaalan, and A. E. Hassanien, Eds., Cham: Springer International Publishing, 2021, pp. 463–480. doi: 10.1007/978-3-030-47411-9_25.
[4]. B. Kayalibay, G. Jensen, and P. van der Smagt, “CNN-based Segmentation of Medical Imaging Data,” Jul. 25, 2017, arXiv: arXiv:1701.03056. doi: 10.48550/arXiv.1701.03056.
[5]. O. Ronneberger, P. Fischer, and T. Brox, “U-Net: Convolutional Networks for Biomedical Image Segmentation,” in Medical Image Computing and Computer-Assisted Intervention – MICCAI 2015, N. Navab, J. Hornegger, W. M. Wells, and A. F. Frangi, Eds., Cham: Springer International Publishing, 2015, pp. 234–241. doi: 10.1007/978-3-319-24574-4_28.
[6]. Igor.Slinko., COVID-19 CT Images Segmentation, 2020. [Online]. Available: https://kaggle.com/competitions/covid-segmentation
Cite this article
Zheng,W. (2024). COVID-19 CT Image Segmentation Based on Modified U-Net. Applied and Computational Engineering,104,176-182.
Data availability
The datasets used and/or analyzed during the current study will be available from the authors upon reasonable request.
Disclaimer/Publisher's Note
The statements, opinions and data contained in all publications are solely those of the individual author(s) and contributor(s) and not of EWA Publishing and/or the editor(s). EWA Publishing and/or the editor(s) disclaim responsibility for any injury to people or property resulting from any ideas, methods, instructions or products referred to in the content.
About volume
Volume title: Proceedings of the 2nd International Conference on Machine Learning and Automation
© 2024 by the author(s). Licensee EWA Publishing, Oxford, UK. This article is an open access article distributed under the terms and
conditions of the Creative Commons Attribution (CC BY) license. Authors who
publish this series agree to the following terms:
1. Authors retain copyright and grant the series right of first publication with the work simultaneously licensed under a Creative Commons
Attribution License that allows others to share the work with an acknowledgment of the work's authorship and initial publication in this
series.
2. Authors are able to enter into separate, additional contractual arrangements for the non-exclusive distribution of the series's published
version of the work (e.g., post it to an institutional repository or publish it in a book), with an acknowledgment of its initial
publication in this series.
3. Authors are permitted and encouraged to post their work online (e.g., in institutional repositories or on their website) prior to and
during the submission process, as it can lead to productive exchanges, as well as earlier and greater citation of published work (See
Open access policy for details).