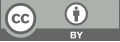
A Novel Filter Bank and Fourier Transform Convolutional Neural Network for SSVEP Classification
- 1 School of Engineering Science, Huazhong University of Science and Technology, Wuhan, Hubei, 430074, China
* Author to whom correspondence should be addressed.
Abstract
Classifying Steady-State Visual Evoked Potentials (SSVEPs) is crucial for enhancing the performance of Brain-Computer Interface (BCI) systems. This study introduces a new method for SSVEP classification within BCI frameworks, called the Filter Bank and Fourier Transform Convolutional Neural Network (FBFCNN). The FBFCNN model effectively extracts key harmonic features by first segmenting SSVEP signals into multiple sub-bands through a filter bank. Subsequently, the Fast Fourier Transform (FFT) is applied to convert these signals from the time domain into the frequency domain. The generated spectrum's real and imaginary components are then fed into a convolutional neural network (CNN). This method improves SSVEP feature extraction and increases classification accuracy by fusing the advantages of CNNs with filter banks. Experimental results, derived from publicly available SSVEP datasets, show that the FBFCNN model surpasses traditional techniques in both accuracy and Information Transfer Rate (ITR). Offering a reliable and efficient solution for real-time brain signal decoding, the FBFCNN model represents a significant advancement in SSVEP-based BCI systems.
Keywords
Convolutional neural networks, Steady-state visual evoked potentials, Brain-computer interface.
[1]. Wolpaw, J. R., Birbaumer, N., Heetderks, W. J., McFarland, D. J., Peckham, P. H., Schalk, G., ... & Vaughan, T. M. (2000). Brain-computer interface technology: a review of the first international meeting. IEEE transactions on rehabilitation engineering, 8(2), 164-173.
[2]. Wang, Y., Wang, R., Gao, X., Hong, B., & Gao, S. (2006). A practical VEP-based brain-computer interface. IEEE Transactions on neural systems and rehabilitation engineering, 14(2), 234-240.
[3]. Vialatte, F. B., Maurice, M., Dauwels, J., & Cichocki, A. (2010). Steady-state visually evoked potentials: focus on essential paradigms and future perspectives. Progress in neurobiology, 90(4), 418-438.
[4]. Guney, O. B., Oblokulov, M., & Ozkan, H. (2021). A deep neural network for ssvep-based brain-computer interfaces. IEEE transactions on biomedical engineering, 69(2), 932-944.
[5]. Bin, G., Gao, X., Yan, Z., Hong, B., & Gao, S. (2009). An online multi-channel SSVEP-based brain–computer interface using a canonical correlation analysis method. Journal of neural engineering, 6(4), 046002.
[6]. Lee, T., Nam, S., & Hyun, D. J. (2022). Adaptive window method based on FBCCA for optimal SSVEP recognition. IEEE Transactions on Neural Systems and Rehabilitation Engineering, 31, 78-86.
[7]. Penaloza, C. I., & Nishio, S. (2018). BMI control of a third arm for multitasking. Science Robotics, 3(20), 1-6.
[8]. Li, Y., Xiang, J., & Kesavadas, T. (2020). Convolutional correlation analysis for enhancing the performance of SSVEP-based brain-computer interface. IEEE Transactions on Neural Systems and Rehabilitation Engineering, 28(12), 2681-2690.
[9]. Zhao, D., Wang, T., Tian, Y., & Jiang, X. (2021). Filter bank convolutional neural network for SSVEP classification. IEEE Access, 9, 147129-147141.
[10]. Chen, J., Zhang, Y., Pan, Y., Xu, P., & Guan, C. (2023). A transformer-based deep neural network model for SSVEP classification. Neural Networks, 164, 521-534.
[11]. Lin, Z., Zhang, C., Wu, W., & Gao, X. (2006). Frequency recognition based on canonical correlation analysis for SSVEP-based BCIs. IEEE transactions on biomedical engineering, 53(12), 2610-2614.
[12]. Ravi, A., Beni, N. H., Manuel, J., & Jiang, N. (2020). Comparing user-dependent and user-independent training of CNN for SSVEP BCI. Journal of neural engineering, 17(2), 026028.
[13]. Yang, J., Zhao, S., Zhang, W., & Liu, X. (2024). High-Order Temporal Convolutional Network for Improving Classification Performance of SSVEP-EEG. IRBM, 45(2), 100830.
[14]. Chen, X., Wang, Y., Gao, S., Jung, T. P., & Gao, X. (2015). Filter bank canonical correlation analysis for implementing a high-speed SSVEP-based brain–computer interface. Journal of neural engineering, 12(4), 046008.
[15]. Nakanishi, M., Wang, Y., Wang, Y. T., & Jung, T. P. (2015). A comparison study of canonical correlation analysis based methods for detecting steady-state visual evoked potentials. PloS one, 10(10), e0140703.
[16]. Zhao, D., Wang, T., Tian, Y., & Jiang, X. (2021). Filter bank convolutional neural network for SSVEP classification. IEEE Access, 9, 147129-147141.
[17]. Kinga, D., & Adam, J. B. (2015). A method for stochastic optimization. In International conference on learning representations, 5, 1-9.
[18]. Wolpaw, J. R., Birbaumer, N., McFarland, D. J., Pfurtscheller, G., & Vaughan, T. M. (2002). Brain–computer interfaces for communication and control. Clinical neurophysiology, 113(6), 767-791.
Cite this article
Xie,J. (2024). A Novel Filter Bank and Fourier Transform Convolutional Neural Network for SSVEP Classification. Applied and Computational Engineering,103,9-16.
Data availability
The datasets used and/or analyzed during the current study will be available from the authors upon reasonable request.
Disclaimer/Publisher's Note
The statements, opinions and data contained in all publications are solely those of the individual author(s) and contributor(s) and not of EWA Publishing and/or the editor(s). EWA Publishing and/or the editor(s) disclaim responsibility for any injury to people or property resulting from any ideas, methods, instructions or products referred to in the content.
About volume
Volume title: Proceedings of the 2nd International Conference on Machine Learning and Automation
© 2024 by the author(s). Licensee EWA Publishing, Oxford, UK. This article is an open access article distributed under the terms and
conditions of the Creative Commons Attribution (CC BY) license. Authors who
publish this series agree to the following terms:
1. Authors retain copyright and grant the series right of first publication with the work simultaneously licensed under a Creative Commons
Attribution License that allows others to share the work with an acknowledgment of the work's authorship and initial publication in this
series.
2. Authors are able to enter into separate, additional contractual arrangements for the non-exclusive distribution of the series's published
version of the work (e.g., post it to an institutional repository or publish it in a book), with an acknowledgment of its initial
publication in this series.
3. Authors are permitted and encouraged to post their work online (e.g., in institutional repositories or on their website) prior to and
during the submission process, as it can lead to productive exchanges, as well as earlier and greater citation of published work (See
Open access policy for details).