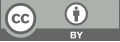
A Survey of Incentive Mechanisms for Federated Learning
- 1 Queen Mary School Hainan, Beijing University of Posts and Telecommunications, Beijing, China
* Author to whom correspondence should be addressed.
Abstract
Federated learning is a cutting-edge distributed machine learning technique that lowers the possibility of data leaking from centralized uploads by enabling users to cooperatively train models. It not only protects client privacy but also better utilizes decentralized data resources, demonstrating high learning performance. To employ their models in the training process, however, a significant number of clients must be involved, which inexorably uses up the device's resources—computational power, communication bandwidth, and energy. Therefore, incentivizing more clients to participate in federated learning is crucial. Typical methods can provide numerical or model-based compensation to ensure they contribute their valuable model resources. This paper provides a brief introduction to federated learning and incentive mechanisms, and surveys related research on federated learning incentives. Specifically, this paper categorizes existing federated learning incentive mechanisms into four technical approaches: Shapley values, Stackelberg games, auctions, and contracts. Finally, the paper discusses some future directions for incentivizing clients in federated learning.
Keywords
Federated learning, Incentive Mechanism, Survey.
[1]. McMahan, H. B. et al. (2017). Communication-efficient learning of deep networks from decentralized data. in Proc. Artif. Intell. Statist. Conf., pp. 1273–1282
[2]. Zheng, Z., Hong, Y., Li, K., Chen, Q. (2024). An Incentive Mechanism Based on AoU, Data Quality, and Data Quantity for Federated Learning. 2024 27th International Conference on Computer Supported Cooperative Work in Design (CSCWD), Tianjin, China, pp. 1832-1837.
[3]. Cao, D., Chang, S., Lin, Z., Liu, G. and Sun, D. (2019). Understanding Distributed Poisoning Attack in Federated Learning. 2019 IEEE 25th International Conference on Parallel and Distributed Systems (ICPADS), Tianjin, China, pp. 233-239.
[4]. Song, T., Tong, Y. and Wei, S. (2019). Profit Allocation for Federated Learning. 2019 IEEE International Conference on Big Data (Big Data), Los Angeles, CA, USA, pp. 2577-2586.
[5]. Wang, T., Rausch, J., Zhang, C., Jia, R., Song, D. (2020). A Principled Approach to Data Valuation for Federated Learning. In: Yang, Q., Fan, L., Yu, H. (eds) Federated Learning. Lecture Notes in Computer Science, vol 12500. Springer, Cham.
[6]. Yang, X., Xiang, S., Peng, C., Tan, W., Yue, W., Liu. H., and Ding, H. (2024). Federated Learning Incentive Mechanism with Supervised Fuzzy Shapley Value. Axioms, 13, no. 4: 254.
[7]. Wang, G., Dang, C. X. and Zhou, Z. (2019). Measure Contribution of Participants in Federated Learning. 2019 IEEE International Conference on Big Data (Big Data), Los Angeles, CA, USA, pp. 2597-2604.
[8]. Guo, W., Wang, Y., Jiang, P. (2023). Incentive mechanism design for Federated Learning with Stackelberg game perspective in the industrial scenario. Computers & Industrial Engineering, 184: 0360-8352.
[9]. Qin, S., Ye, J., Tang, X. and Li, X. (2023). Stackelberg Game-Base Incentive Scheme for Federated Learning in Artificial Intelligence of Things. 2023 IEEE International Conferences on Internet of Things (iThings) and IEEE Green Computing & Communications (GreenCom) and IEEE Cyber, Physical & Social Computing (CPSCom) and IEEE Smart Data (SmartData) and IEEE Congress on Cybermatics (Cybermatics), Danzhou, China, pp. 159-165.
[10]. Yi, Z., Jiao, Y., Dai, W., Li, G., Wang, H. and Xu, Y. (2022). A Stackelberg Incentive Mechanism for Wireless Federated Learning With Differential Privacy. in IEEE Wireless Communications Letters, vol. 11, no. 9, pp. 1805-1809.
[11]. Pang, J., Yu, J., Zhou, R. and Lui, J. C. S. (2023). An Incentive Auction for Heterogeneous Client Selection in Federated Learning. in IEEE Transactions on Mobile Computing, vol. 22, no. 10, pp. 5733-5750.
[12]. Xu, B., Zuo, L., Jin, J., et al. (2023). CAB: a combinatorial-auction-and-bargaining-based federated learning incentive mechanism. World Wide b, 26, 2351–2372.
[13]. Deng, Y. et al. (2021). FAIR: Quality-Aware Federated Learning with Precise User Incentive and Model Aggregation. IEEE INFOCOM 2021 - IEEE Conference on Computer Communications, Vancouver, BC, Canada, pp. 1-10.
[14]. Cao, M., Wang, Q., Wang, Q. (2024). Federated learning in smart home: A dynamic contract based approach with task preferences. Computer Networks, 249: 1389-1286.
[15]. Xuan, S., Wang, M., Zhang, J., Wang, W., Man, D., Yang, W. (2024). An incentive mechanism design for federated learning with multiple task publishers by contract theory approach. Information Sciences, 664: 0020-0255.
Cite this article
Zhou,Y. (2024). A Survey of Incentive Mechanisms for Federated Learning. Applied and Computational Engineering,99,121-127.
Data availability
The datasets used and/or analyzed during the current study will be available from the authors upon reasonable request.
Disclaimer/Publisher's Note
The statements, opinions and data contained in all publications are solely those of the individual author(s) and contributor(s) and not of EWA Publishing and/or the editor(s). EWA Publishing and/or the editor(s) disclaim responsibility for any injury to people or property resulting from any ideas, methods, instructions or products referred to in the content.
About volume
Volume title: Proceedings of the 5th International Conference on Signal Processing and Machine Learning
© 2024 by the author(s). Licensee EWA Publishing, Oxford, UK. This article is an open access article distributed under the terms and
conditions of the Creative Commons Attribution (CC BY) license. Authors who
publish this series agree to the following terms:
1. Authors retain copyright and grant the series right of first publication with the work simultaneously licensed under a Creative Commons
Attribution License that allows others to share the work with an acknowledgment of the work's authorship and initial publication in this
series.
2. Authors are able to enter into separate, additional contractual arrangements for the non-exclusive distribution of the series's published
version of the work (e.g., post it to an institutional repository or publish it in a book), with an acknowledgment of its initial
publication in this series.
3. Authors are permitted and encouraged to post their work online (e.g., in institutional repositories or on their website) prior to and
during the submission process, as it can lead to productive exchanges, as well as earlier and greater citation of published work (See
Open access policy for details).