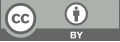
Enhancing Image Stitching Algorithms with SIFT Feature Detection
- 1 School of computing, Neusoft Institute Guangdong, Foshan, China
* Author to whom correspondence should be addressed.
Abstract
Image stitching technology plays a significant role in the fields of computer vision and image processing, with applications ranging from panoramic photography to virtual reality (VR), augmented reality (AR), medical diagnostics, and autonomous vehicle technology. As technology advances, the demand for high-quality, real-time panoramic images provided by image stitching technology continues to grow. This study aims to implement an image stitching method based on the Scale-Invariant Feature Transform (SIFT) feature point detection algorithm, combined with the Random Sampling Consensus (RANSAC) algorithm and the calculation of the homography matrix to automatically stitch two images. This paper elaborates on the entire process of image feature extraction, feature matching, homography matrix calculation, and image fusion, and compares different fusion modes. The experimental results show that the method can achieve seamless image stitching in some cases, its performance in complex scenes such as crowds or traffic flows is average. This study provides new perspectives and methods for the application of image stitching technology.
Keywords
Image stitching, SIFT feature detection, RANSAC algorithm, homography matrix.
[1]. Lowe, D.G. (2004). Distinctive image features from scale-invariant keypoints. International Journal of Computer Vision, 60(2), 91-110.
[2]. Mikolajczyk, K., & Schmid, C. (2005). A Performance Evaluation of Local Descriptors. IEEE Transactions on Pattern Analysis and Machine Intelligence, 27(10), 1615-1630. doi:10.1109/TPAMI.2005.188
[3]. Hartley, R. I., & Zisserman, A. (2003). Multiple View Geometry in Computer Vision. Cambridge University Press.
[4]. Fischler, M.A., & Bolles, R.J. (1981). Random sample consensus: A paradigm for model fitting with applications to image analysis and automated cartography. Communications of the ACM, 24(6), 381-395.
[5]. Bay, H., Tuytelaars, T., & Van Gool, L. (2006). SURF: Speeded Up Robust Features. Computer Vision and Image Understanding, 110(3), 346-359.
[6]. Kaiser, P., & Schmid, C. (2011). Efficient SIFT matching with a multi-layer approach. Image and Vision Computing, 29(9), 645-654.
[7]. Brown, M.S., & Lowe, D.G. (2007). Automatic panoramic image stitching using invariant features. International Journal of Computer Vision, 74(1), 59-73.
[8]. Zhang, Z. (1999). A flexible new technique for camera calibration. IEEE Transactions on Pattern Analysis and Machine Intelligence, 22(11), 1330-1334.
Cite this article
Wang,M. (2024). Enhancing Image Stitching Algorithms with SIFT Feature Detection. Applied and Computational Engineering,105,74-81.
Data availability
The datasets used and/or analyzed during the current study will be available from the authors upon reasonable request.
Disclaimer/Publisher's Note
The statements, opinions and data contained in all publications are solely those of the individual author(s) and contributor(s) and not of EWA Publishing and/or the editor(s). EWA Publishing and/or the editor(s) disclaim responsibility for any injury to people or property resulting from any ideas, methods, instructions or products referred to in the content.
About volume
Volume title: Proceedings of CONF-MLA 2024 Workshop: Neural Computing and Applications
© 2024 by the author(s). Licensee EWA Publishing, Oxford, UK. This article is an open access article distributed under the terms and
conditions of the Creative Commons Attribution (CC BY) license. Authors who
publish this series agree to the following terms:
1. Authors retain copyright and grant the series right of first publication with the work simultaneously licensed under a Creative Commons
Attribution License that allows others to share the work with an acknowledgment of the work's authorship and initial publication in this
series.
2. Authors are able to enter into separate, additional contractual arrangements for the non-exclusive distribution of the series's published
version of the work (e.g., post it to an institutional repository or publish it in a book), with an acknowledgment of its initial
publication in this series.
3. Authors are permitted and encouraged to post their work online (e.g., in institutional repositories or on their website) prior to and
during the submission process, as it can lead to productive exchanges, as well as earlier and greater citation of published work (See
Open access policy for details).