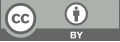
Applications of Denoising Diffusion Probability Model in Image Processing
- 1 School of Sciences, Tianjin University of Commerce, Tianjin, China
- 2 School of Mathematical Sciences, Nanjing Normal University, Jiangsu, China
- 3 School of Mathematics, Hunan University, Hunan, China
* Author to whom correspondence should be addressed.
Abstract
In 2020, Jonathan Ho et al. from the University of Berkeley proposed the Denoising Diffusion Probabilistic Models (DDPM), which improved on the shortcomings of the previous-generation Deformable Part Models (DPM). At the same time, they also surpassed previous generative models in image synthesis effects by using noise prediction, such as Generative Adversarial Networks (GAN), Variational Auto-Encoders (VAE), flow-based models and energy-based models, etc. After that, the denoising diffusion probabilistic models gradually received more discussions and research. In 2022, OpenAI launched the DALL-E 2, realizing Text-to-Image generation combined with the denoising diffusion probabilistic model. Two years later, with the release of Sora, the first Text-to-Video large models based on DALL-E, the denoising diffusion probabilistic models have received unprecedented attention in the multi-modal field. This article first provides the background and development process of the denoising diffusion probabilistic models. Secondly, it introduces the algorithmic principles of the diffusion models. Following that, it lists the applications of the models in the area of images. In the last section, it expounds on the advantages, disadvantages and future trends of the denoising diffusion probabilistic models.
Keywords
Generative Models, Diffusion Models, Noise Prediction, Text to Image, Multi-modality.
[1]. Ho, J., Jain, A., & Abbeel, P. (2020). Denoising diffusion probabilistic models. In Advances in Neural Information Processing Systems, Vol. 33. 6840–6851.
[2]. Sohl-Dickstein, J., Weiss, E. A., Maheswaranathan, N., & Ganguli, S. (2015). Deep unsupervised learning using nonequalibrium thermodynamics. In International Conference on Machine Learning. 2256–2265.
[3]. Song, J., Meng, C., & Ermon, S. (2020). Denoising Diffusion Implicit Models. In International Conference on Learning Representations.
[4]. Nichol, A., & Dhariwal, P. (2021). Improved denoising diffusion probabilistic models. In International Conference on Machine Learning. 8162–8171.
[5]. Cachay, S. R., Zhao, B., Joren, H., & Yu, R. (2022). DYffusion: A Dynamics-informed Diffusion Model for Spatiotemporal Forecasting. In Neural Information Processing Systems.
[6]. Lugmayr, A., Danelljan, M., Romero, A., Yu, F., Timofte, R., & Gool, L. V. (2022). RePaint: Inp-ainting using Denoising Diffusion Probabilistic Models. In Conference on Computer Vision and Pattern Recognition.
[7]. Khader, F., Mueller-Franzes, G., Tayebi Arasteh, S., Han, T., Haarburger, C., Schulze-Hagen, M., Schad, P., Engelhardt, S., Baessler, B., Foersch, S., Stegmaier, J., Kuhl, C., Nebelung, S., Kather, J. N., & Truhn, D. (2023). Denoising diffusion probabilistic models for 3D medical image generation. In Scientific Reports.
[8]. Lu, S., Guan, F., Zhang, H., & Lai, H. (2023). Underwater image enhancement method based on denoising diffusion probabilistic model. In Social Science Research Network.
[9]. Gong, K., Johnson, K. A., Fakhri, G. E., Li, Q., & Pan, T. (2022). PET image denoising based on denoising diffusion probabilistic models. In European Journal of Nuclear Medicine and Molecular Imaging.
[10]. Anand, N., & Achim, T. (2022). Protein structure and Sequence Generation with Equivariant Denoising Probabilistic Diffusion Models. In Neural Information Processing Systems.
Cite this article
He,R.;Jiang,R.;Miao,Z. (2024). Applications of Denoising Diffusion Probability Model in Image Processing. Applied and Computational Engineering,97,108-114.
Data availability
The datasets used and/or analyzed during the current study will be available from the authors upon reasonable request.
Disclaimer/Publisher's Note
The statements, opinions and data contained in all publications are solely those of the individual author(s) and contributor(s) and not of EWA Publishing and/or the editor(s). EWA Publishing and/or the editor(s) disclaim responsibility for any injury to people or property resulting from any ideas, methods, instructions or products referred to in the content.
About volume
Volume title: Proceedings of the 2nd International Conference on Machine Learning and Automation
© 2024 by the author(s). Licensee EWA Publishing, Oxford, UK. This article is an open access article distributed under the terms and
conditions of the Creative Commons Attribution (CC BY) license. Authors who
publish this series agree to the following terms:
1. Authors retain copyright and grant the series right of first publication with the work simultaneously licensed under a Creative Commons
Attribution License that allows others to share the work with an acknowledgment of the work's authorship and initial publication in this
series.
2. Authors are able to enter into separate, additional contractual arrangements for the non-exclusive distribution of the series's published
version of the work (e.g., post it to an institutional repository or publish it in a book), with an acknowledgment of its initial
publication in this series.
3. Authors are permitted and encouraged to post their work online (e.g., in institutional repositories or on their website) prior to and
during the submission process, as it can lead to productive exchanges, as well as earlier and greater citation of published work (See
Open access policy for details).