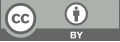
Federated Learning for Privacy-Preserving Medical Data Sharing in Drug Development
- 1 Innovation Management and Entrepreneurship, Brown University, RI, USA
- 2 Chemical and Biomolecular Engineering, University of Pennsylvania, Philadelphia, PA, USA
- 3 Electronics & Communication Engineering, University of Electronic Science and Technology of China, Chengdu, China
- 4 Management Information Systems, New York University, NY, USA
* Author to whom correspondence should be addressed.
Abstract
This study explores the potential of Federated Learning (FL) to facilitate the sharing and collaboration of medical data in drug development under the premise of privacy protection. This paper systematically describes the core mechanism of federated learning, including the key technologies such as model parameter updating, differential privacy and homomorphic encryption, and their applications in drug development and medical data processing. Examples, such as NVIDIA Clara's Federated learning application and COVID-19 resource prediction, show that federated learning improves the efficiency of multi-party collaboration and model performance while ensuring data privacy, especially in areas such as finance and insurance, where data privacy is critical.
Keywords
Federated Learning, Data Privacy, Drug Development, Distributed AI
[1]. Bakas, S., et al.: Identifying the best machine learning algorithms for brain tumor segmentation, progression assessment, and overall survival prediction in the BRATS challenge. arXiv:1811.02629 (2018).
[2]. Hitaj, B., Ateniese, G., Perez-Cruz, F.: Deep models under the GAN: information leakage from collaborative deep learning. In: SIGSAC. pp. 603–618. ACM (2017)
[3]. Kingma, D.P., Ba, J.: Adam: A method for stochastic optimization.arXiv:1412.6980 (2014)
[4]. Li, L., Fan, Y., Tse, M., & Lin, K. Y. (2020). A review of applications in federated learning. Computers & Industrial Engineering, 149, 106854.
[5]. Geyer, R.C., Klein, T., Nabi, M.: Differentially private federated learning: A client level perspective. arXiv:1712.07557 (2017).
[6]. Truex, S., Baracaldo, N., Anwar, A., Steinke, T., Ludwig, H., Zhang, R., & Zhou, Y. (2019, November). A hybrid approach to privacy-preserving federated learning. In Proceedings of the 12th ACM workshop on artificial intelligence and security (pp. 1-11).
[7]. Xu, K., Zhou, H., Zheng, H., Zhu, M., & Xin, Q. (2024). Intelligent Classification and Personalized Recommendation of E-commerce Products Based on Machine Learning. arXiv preprint arXiv:2403.19345.
[8]. Xu, K., Zheng, H., Zhan, X., Zhou, S., & Niu, K. (2024). Evaluation and Optimization of Intelligent Recommendation System Performance with Cloud Resource Automation Compatibility.
[9]. Zheng, H., Xu, K., Zhou, H., Wang, Y., & Su, G. (2024). Medication Recommendation System Based on Natural Language Processing for Patient Emotion Analysis. Academic Journal of Science and Technology, 10(1), 62-68.
[10]. Zheng, H.; Wu, J.; Song, R.; Guo, L.; Xu, Z. Predicting Financial Enterprise Stocks and Economic Data Trends Using Machine Learning Time Series Analysis. Applied and Computational Engineering 2024, 87, 26–32.
Cite this article
Yang,M.;Huang,D.;Wan,W.;Jin,M. (2024). Federated Learning for Privacy-Preserving Medical Data Sharing in Drug Development. Applied and Computational Engineering,108,7-13.
Data availability
The datasets used and/or analyzed during the current study will be available from the authors upon reasonable request.
Disclaimer/Publisher's Note
The statements, opinions and data contained in all publications are solely those of the individual author(s) and contributor(s) and not of EWA Publishing and/or the editor(s). EWA Publishing and/or the editor(s) disclaim responsibility for any injury to people or property resulting from any ideas, methods, instructions or products referred to in the content.
About volume
Volume title: Proceedings of the 5th International Conference on Signal Processing and Machine Learning
© 2024 by the author(s). Licensee EWA Publishing, Oxford, UK. This article is an open access article distributed under the terms and
conditions of the Creative Commons Attribution (CC BY) license. Authors who
publish this series agree to the following terms:
1. Authors retain copyright and grant the series right of first publication with the work simultaneously licensed under a Creative Commons
Attribution License that allows others to share the work with an acknowledgment of the work's authorship and initial publication in this
series.
2. Authors are able to enter into separate, additional contractual arrangements for the non-exclusive distribution of the series's published
version of the work (e.g., post it to an institutional repository or publish it in a book), with an acknowledgment of its initial
publication in this series.
3. Authors are permitted and encouraged to post their work online (e.g., in institutional repositories or on their website) prior to and
during the submission process, as it can lead to productive exchanges, as well as earlier and greater citation of published work (See
Open access policy for details).