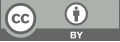
A Review of the State of the Art 3D Generative Models and Their Applications
- 1 Department of Computing Science, University of Alberta, Edmonton, Canada
* Author to whom correspondence should be addressed.
Abstract
Ever since 2022, there has been a large number of 3D generative models that have been devised and published, such as AvatarGen, CityDreamer, and HOLOFUSION. Generally speaking, these models can perform tasks such as generating a 3D human model, creating an unbounded city scene, and constructing a 3D object. And it is not a surprise that 3D generative models are very popular these years because there has been a witness of huge need for 3D models in the global market and the models themselves also serve as both convenient and productive tools for the relevant industries. For instance, 3D generative models can utilize a combination of Generative Adversarial Network (GAN) and Multi-Layer Perceptron (MLP) or Neural Radiance Field (NeRF) or Diffusion Model to produce 3D human model; Autoregressive Model or Feature Extraction + Volume Rendering to generate 3D scenes; Diffusion Model or GAN + MLP to produce 3D objects. This paper tries to present a taxonomy of the main 3D generative models from the angle of the kinds of outputs and strategies employed by different models.
Keywords
3D Generative Model, 3D Human Model, 3D Scene, 3D Object
[1]. Gao, K., Gao, Y., He, H., Lu, D., Xu, L., & Li, J. (2022). Nerf: Neural radiance field in 3d vision, a comprehensive review. arXiv preprint arXiv:2210.00379.
[2]. Jones, M. W., Baerentzen, J. A., & Sramek, M. (2006). 3D distance fields: A survey of techniques and applications. IEEE Transactions on visualization and Computer Graphics, 12(4), 581-599.
[3]. Loper, M., Mahmood, N., Romero, J., Pons-Moll, G., & Black, M. J. (2023). SMPL: A skinned multi-person linear model. In Seminal Graphics Papers: Pushing the Boundaries, Volume 2 (pp. 851-866).
[4]. Farsiu, S., Robinson, M. D., Elad, M., & Milanfar, P. (2004). Fast and robust multiframe super resolution. IEEE transactions on image processing, 13(10), 1327-1344.
[5]. Chen, Z., Wang, G., & Liu, Z. (2023). Scenedreamer: Unbounded 3d scene generation from 2d image collections. IEEE transactions on pattern analysis and machine intelligence.
[6]. Zhang, J., Jiang, Z., Yang, D., Xu, H., Shi, Y., Song, G., ... & Feng, J. (2022, October). Avatargen: a 3d generative model for animatable human avatars. In European Conference on Computer Vision (pp. 668-685). Cham: Springer Nature Switzerland.
[7]. Hong, F., Chen, Z., Lan, Y., Pan, L., & Liu, Z. (2022). Eva3d: Compositional 3d human generation from 2d image collections. arXiv preprint arXiv:2210.04888.
[8]. Huang, X., Shao, R., Zhang, Q., Zhang, H., Feng, Y., Liu, Y., & Wang, Q. (2024). Humannorm: Learning normal diffusion model for high-quality and realistic 3d human generation. In Proceedings of the IEEE/CVF Conference on Computer Vision and Pattern Recognition (pp. 4568-4577).
[9]. Shen, T., Gao, J., Yin, K., Liu, M. Y., & Fidler, S. (2021). Deep marching tetrahedra: a hybrid representation for high-resolution 3d shape synthesis. Advances in Neural Information Processing Systems, 34, 6087-6101.
[10]. Shen, Y., Ma, W. C., & Wang, S. (2022). SGAM: Building a virtual 3d world through simultaneous generation and mapping. Advances in Neural Information Processing Systems, 35, 22090-22102.
[11]. Karnewar, A., Vedaldi, A., Novotny, D., & Mitra, N. J. (2023). Holodiffusion: Training a 3d diffusion model using 2d images. In Proceedings of the IEEE/CVF conference on computer vision and pattern recognition (pp. 18423-18433).
[12]. Xu, J., Wang, X., Cheng, W., Cao, Y. P., Shan, Y., Qie, X., & Gao, S. (2023). Dream3d: Zero-shot text-to-3d synthesis using 3d shape prior and text-to-image diffusion models. In Proceedings of the IEEE/CVF Conference on Computer Vision and Pattern Recognition (pp. 20908-20918).
Cite this article
Qiu,Z. (2024). A Review of the State of the Art 3D Generative Models and Their Applications. Applied and Computational Engineering,112,123-129.
Data availability
The datasets used and/or analyzed during the current study will be available from the authors upon reasonable request.
Disclaimer/Publisher's Note
The statements, opinions and data contained in all publications are solely those of the individual author(s) and contributor(s) and not of EWA Publishing and/or the editor(s). EWA Publishing and/or the editor(s) disclaim responsibility for any injury to people or property resulting from any ideas, methods, instructions or products referred to in the content.
About volume
Volume title: Proceedings of the 5th International Conference on Signal Processing and Machine Learning
© 2024 by the author(s). Licensee EWA Publishing, Oxford, UK. This article is an open access article distributed under the terms and
conditions of the Creative Commons Attribution (CC BY) license. Authors who
publish this series agree to the following terms:
1. Authors retain copyright and grant the series right of first publication with the work simultaneously licensed under a Creative Commons
Attribution License that allows others to share the work with an acknowledgment of the work's authorship and initial publication in this
series.
2. Authors are able to enter into separate, additional contractual arrangements for the non-exclusive distribution of the series's published
version of the work (e.g., post it to an institutional repository or publish it in a book), with an acknowledgment of its initial
publication in this series.
3. Authors are permitted and encouraged to post their work online (e.g., in institutional repositories or on their website) prior to and
during the submission process, as it can lead to productive exchanges, as well as earlier and greater citation of published work (See
Open access policy for details).