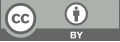
Analysis of Different Methods in Pedestrian Re-identification
- 1 Information Science and Technology, Xiamen University, Xiamen, China
* Author to whom correspondence should be addressed.
Abstract
This study focuses on the field of pedestrian re-identification (ReID), aiming to enhance the accuracy and efficiency of individual recognition through advanced deep learning models. The article first introduces two deep learning models: Omni-Scale Feature Learning for Person Re-Identification (OSNet) and Multi-Scale Interaction Network (MSINet). OSNet employs Depthwise Separable Convolutions (DSC) and Omni-Scale Residual Blocks to improve the ability to learn features across different scales. MSINet, on the other hand, utilizes neural architecture search technology to design a lightweight network architecture, enhancing feature discrimination and flexible utilization through Twins Contrastive Mechanism (TCM) and Multi-Scale Interaction (MSI). Additionally, a Spatial Alignment Module (SAM) is proposed to enhance the consistency of images under different viewpoints or conditions. The experimental section selects two widely used pedestrian re-identification datasets, Market1501 and MSMT17, for evaluation, and the results show that MSINet outperforms existing methods in terms of accuracy and stability. The article concludes by summarizing the advantages of OSNet and MSINet in multi-scale feature learning and points out their application limitations.
Keywords
Person re-ID,Comparison, MSINet, OSNet
[1]. Zheng, L., Wang, S., & Wang, J. (2016). Person Re-identification: A Benchmark. IEEE Transactions on Pattern Analysis and Machine Intelligence, 40(1), 1-20. doi:10.1109/TPAMI.2016.2593860.
[2]. Wu, Y., & Zheng, Y. (2019). A Comprehensive Review on Person Re-identification: From Feature Representation to Matching Strategy. ACM Computing Surveys, 54(3), 1-35. doi:10.1145/3299900.
[3]. Zhou, K., Yang, Y., Cavallaro, A., & Xiang, T. (2019). Omni-Scale Feature Learning for Person Re-Identification. arXiv preprint arXiv:1905.00953
[4]. Gu, J., Wang, K., Luo, H., Chen, C., Jiang, W., Fang, Y., Zhang, S., You, Y., & Zhao, J. (2023). MSINet: Twins Contrastive Search of Multi-Scale Interaction for Object ReID. arXiv preprint arXiv:2303.07065
[5]. Sun, Y., Zheng, L., & Wang, S. (2018). Beyondpart Models for Person Re-Identification. Proceedings of the 2018 IEEE/CVF Conference on Computer Vision and Pattern Recognition (CVPR), 1-15. doi:10.1109/CVPR.2018.00456.
[6]. Li, W., Zhang, X., & Wang, J. (2018). Hetero-Feature Fusion for Person Re-Identification. Proceedings of the 2018 IEEE/CVF Conference on Computer Vision and Pattern Recognition (CVPR), 1-10. doi:10.1109/CVPR.2018.00368.
[7]. Chen, Y., & Wang, G. (2019). Relation Network for Person Re-identification. Proceedings of the 2019 IEEE/CVF Conference on Computer Vision and Pattern Recognition (CVPR), 1-10. doi:10.1109/CVPR.2019.00289.
[8]. Zhao, R., Zhang, L., & Wang, H. (2017). Person Re-identification by Deep Learning: A Review. Journal of Multimedia, 12(6), 493-505. doi:10.18178/jmm.2017.12.6.410.
[9]. Huang, K., & Wang, Y. (2020). Person Re-identification: A Survey of the State of the art. IEEE Transactions on Image Processing, 29, 8846-8863. doi:10.1109/TIP.2020.2993405.
Cite this article
Li,K. (2024). Analysis of Different Methods in Pedestrian Re-identification. Applied and Computational Engineering,105,123-130.
Data availability
The datasets used and/or analyzed during the current study will be available from the authors upon reasonable request.
Disclaimer/Publisher's Note
The statements, opinions and data contained in all publications are solely those of the individual author(s) and contributor(s) and not of EWA Publishing and/or the editor(s). EWA Publishing and/or the editor(s) disclaim responsibility for any injury to people or property resulting from any ideas, methods, instructions or products referred to in the content.
About volume
Volume title: Proceedings of CONF-MLA 2024 Workshop: Neural Computing and Applications
© 2024 by the author(s). Licensee EWA Publishing, Oxford, UK. This article is an open access article distributed under the terms and
conditions of the Creative Commons Attribution (CC BY) license. Authors who
publish this series agree to the following terms:
1. Authors retain copyright and grant the series right of first publication with the work simultaneously licensed under a Creative Commons
Attribution License that allows others to share the work with an acknowledgment of the work's authorship and initial publication in this
series.
2. Authors are able to enter into separate, additional contractual arrangements for the non-exclusive distribution of the series's published
version of the work (e.g., post it to an institutional repository or publish it in a book), with an acknowledgment of its initial
publication in this series.
3. Authors are permitted and encouraged to post their work online (e.g., in institutional repositories or on their website) prior to and
during the submission process, as it can lead to productive exchanges, as well as earlier and greater citation of published work (See
Open access policy for details).