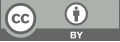
Leveraging Machine Learning for Food Waste Reduction: An Analysis of Predictive Models
- 1 College of Food Science, Sichuan Agricultural University, China
- 2 Information School, University of Washington, USA
- 3 University of South Florida, Tampa, Florida, USA
* Author to whom correspondence should be addressed.
Abstract
Food waste remains a significant global issue with severe environmental, economic, and social impacts. Recent advancements in machine learning (ML) have shown promise in tackling this problem across various sectors, including household, catering, and hospitality industries. This study explores the application of machine learning models to predict and minimize food waste, focusing on key predictors such as household consumption patterns, retail demand, and food service estimates. By analyzing the effectiveness of random forests, this research highlights the ability of these models to enhance forecasting accuracy and waste management efficiency. Findings underscore the potential of ML to support sustainable food consumption, reduce waste generation, and achieve environmental benefits. This research offers insights into the practical deployment of ML for food waste mitigation, providing a roadmap for stakeholders aiming to adopt technology-driven waste reduction strategies.
Keywords
Food waste reduction; Machine learning; Predictive modeling; Random forest
[1]. Panda S K, Dwivedi M. Minimizing food wastage using machine learning: A novel approach[C]//Smart Intelligent Computing and Applications: Proceedings of the Third International Conference on Smart Computing and Informatics, Volume 1. Springer Singapore, 2020: 465-473.
[2]. Dey S, Saha S, Singh A K, et al. SmartNoshWaste: Using blockchain, machine learning, cloud computing and QR code to reduce food waste in decentralized web 3.0 enabled smart cities[J]. Smart Cities, 2022, 5(1): 162-176.
[3]. Rodrigues M, Miguéis V, Freitas S, et al. Machine learning models for short-term demand forecasting in food catering services: A solution to reduce food waste[J]. Journal of Cleaner Production, 2024, 435: 140265.
[4]. Malefors C, Secondi L, Marchetti S, et al. Food waste reduction and economic savings in times of crisis: The potential of machine learning methods to plan guest attendance in Swedish public catering during the Covid-19 pandemic[J]. Socio-Economic Planning Sciences, 2022, 82: 101041.
[5]. Tang J, Tang Y, Liu Y, et al. Using machine learning method to predict food waste in catering industry under high resolution: a case in Dongguan[J]. Journal of Material Cycles and Waste Management, 2023, 25(4): 2442-2456.
[6]. Singh B, Kaunert C. Salvaging Responsible Consumption and Production of Food in the Hospitality Industry: Harnessing Machine Learning and Deep Learning for Zero Food Waste[M]//Sustainable Disposal Methods of Food Wastes in Hospitality Operations. IGI Global, 2024: 176-192.
[7]. Zhang X, Liu H, Yang G, et al. Comprehensive insights into the application strategy of kitchen waste derived hydrochar: Random forest-based modelling[J]. Chemical Engineering Journal, 2023, 469: 143840.
[8]. Grainger M J, Aramyan L, Piras S, et al. Model selection and averaging in the assessment of the drivers of household food waste to reduce the probability of false positives[J]. PloS one, 2018, 13(2): e0192075.
[9]. Biau G, Scornet E. A random forest guided tour[J]. Test, 2016, 25: 197-227.
[10]. Breiman L. Random forests[J]. Machine learning, 2001, 45: 5-32.
Cite this article
Yang,Y.;Zhao,C.;Lu,H. (2024). Leveraging Machine Learning for Food Waste Reduction: An Analysis of Predictive Models. Applied and Computational Engineering,112,154-160.
Data availability
The datasets used and/or analyzed during the current study will be available from the authors upon reasonable request.
Disclaimer/Publisher's Note
The statements, opinions and data contained in all publications are solely those of the individual author(s) and contributor(s) and not of EWA Publishing and/or the editor(s). EWA Publishing and/or the editor(s) disclaim responsibility for any injury to people or property resulting from any ideas, methods, instructions or products referred to in the content.
About volume
Volume title: Proceedings of the 5th International Conference on Signal Processing and Machine Learning
© 2024 by the author(s). Licensee EWA Publishing, Oxford, UK. This article is an open access article distributed under the terms and
conditions of the Creative Commons Attribution (CC BY) license. Authors who
publish this series agree to the following terms:
1. Authors retain copyright and grant the series right of first publication with the work simultaneously licensed under a Creative Commons
Attribution License that allows others to share the work with an acknowledgment of the work's authorship and initial publication in this
series.
2. Authors are able to enter into separate, additional contractual arrangements for the non-exclusive distribution of the series's published
version of the work (e.g., post it to an institutional repository or publish it in a book), with an acknowledgment of its initial
publication in this series.
3. Authors are permitted and encouraged to post their work online (e.g., in institutional repositories or on their website) prior to and
during the submission process, as it can lead to productive exchanges, as well as earlier and greater citation of published work (See
Open access policy for details).