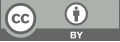
Big Data Analytics and Implementation Challenges of Machine Learning in Big data
- 1 Department of Computer Engineering, PSG Polytechnic College, Peelamedu, Coimbatore, Tamilnadu- 641004
- 2 Department of Industrial Engineering, Hanyang University, 222 Wangsimini-ro, Seongdong-gu, Seoul, Republic of Korea
- 3 Department of Information Technology, GMR Institute of Technology, Srikakulam, AP-532127
- 4 Department of Computer Engineering, PSG Polytechnic College, Peelamedu,, Coimbatore, Tamilnadu-641004.
- 5 School of Computing and Information Technology, REVA University, Bengaluru, Karnataka, India
* Author to whom correspondence should be addressed.
Abstract
The term "big data" refers to an information processing system that combines different conventional data techniques. Big data also includes a large amount of personally identifiable and authenticated data, making privacy a major concern. Various techniques have been developed to provide security and efficient data processing. Machine learning is a form of data technology that deals with one of the most important and least understood aspects of the data collected. Deep learning algorithms, similar to machine learning algorithms, learn programmers automatically from data and are thought to improve the efficiency and security of large data sets. The efficiency of machine learning and deep learning in a sensitive environment was evaluated in this paper, which reviewed security problems in big data. This paper begins by providing an overview of machine learning and deep learning algorithms. The research then moves on to machine learning problems and challenges, as well as potential solutions. The investigation into deep learning principles of big data continues after that. Finally, the report examines approaches used in recent research developments and concludes with recommendations for the future.
Keywords
Machine learning, big data, Deep learning, artificial intelligence, data mining, security issues.
[1]. Qiu, J., Wu, Q., Ding, G., Xu, Y., & Feng, S. (2016). A survey of machine learning for big data processing. EURASIP Journal on Advances in Signal Processing, 2016(1), 1-16
[2]. Al-Jarrah, O. Y., Yoo, P. D., Muhaidat, S., Karagiannidis, G. K., & Taha, K. (2015). Efficient machine learning for big data: A review. Big Data Research, 2(3), 87-93.
[3]. Sun, A. Y., & Scanlon, B. R. (2019). How can Big Data and machine learning benefit environment and water management: a survey of methods, applications, and future directions. Environmental Research Letters, 14(7), 073001.
[4]. López Belmonte, J., Segura-Robles, A., Moreno-Guerrero, A. J., & Parra-González, M. E. (2020). Machine learning and big data in the impact literature. A bibliometric review with scientific mapping in Web of science. Symmetry, 12(4), 495.
[5]. Zincir-Heywood, N., Casale, G., Carrera, D., Chen, L. Y., Dhamdhere, A., Inoue, T., ... & Samak, T. (2020). Guest Editorial: Special Section on Data Analytics and Machine Learning for Network and Service Management–Part I. IEEE Transactions on Network and Service Management, 17(4), 1971-1974.
Cite this article
P.,R.;E.,S.V.;Anilkumar,C.;Thilakaveni,P.;Moorthy,U. (2023). Big Data Analytics and Implementation Challenges of Machine Learning in Big data. Applied and Computational Engineering,2,484-489.
Data availability
The datasets used and/or analyzed during the current study will be available from the authors upon reasonable request.
Disclaimer/Publisher's Note
The statements, opinions and data contained in all publications are solely those of the individual author(s) and contributor(s) and not of EWA Publishing and/or the editor(s). EWA Publishing and/or the editor(s) disclaim responsibility for any injury to people or property resulting from any ideas, methods, instructions or products referred to in the content.
About volume
Volume title: Proceedings of the 4th International Conference on Computing and Data Science (CONF-CDS 2022)
© 2024 by the author(s). Licensee EWA Publishing, Oxford, UK. This article is an open access article distributed under the terms and
conditions of the Creative Commons Attribution (CC BY) license. Authors who
publish this series agree to the following terms:
1. Authors retain copyright and grant the series right of first publication with the work simultaneously licensed under a Creative Commons
Attribution License that allows others to share the work with an acknowledgment of the work's authorship and initial publication in this
series.
2. Authors are able to enter into separate, additional contractual arrangements for the non-exclusive distribution of the series's published
version of the work (e.g., post it to an institutional repository or publish it in a book), with an acknowledgment of its initial
publication in this series.
3. Authors are permitted and encouraged to post their work online (e.g., in institutional repositories or on their website) prior to and
during the submission process, as it can lead to productive exchanges, as well as earlier and greater citation of published work (See
Open access policy for details).