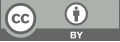
Comparative Analysis of Expected Goals Models: Evaluating Predictive Accuracy and Feature Importance in European Soccer
- 1 High School Affiliated to Renmin University of China, Beijing, China
* Author to whom correspondence should be addressed.
Abstract
Expected Goals (xG) is a widely used metric in soccer analytics that estimates the probability of a shot resulting in a goal based on various characteristics of the shot. This study compares the predictive accuracy and feature importance of two prominent xG models: Opta and Understat. Using data from the top five European leagues from the 2017-2018 to the 2023-2024 seasons, we evaluate the predictive accuracy of each model using L1 and L2 loss metrics. Our findings indicate that Understat outperforms Opta in terms of lower prediction errors in the Bundesliga, Premier League, and Serie A, while Opta yields more stable predictions in La Liga and Ligue 1. We further analyze the factors influencing xG predictions through feature importance techniques using Random Forest and XGBoost models, complemented by SHAP (SHapley Additive exPlanations) analysis. Results reveal that goal exposure angle, shooting angle, and shot distance are key features in predicting goal probability, with differences in how categorical variables are weighted between the models. The study concludes with a discussion of the strengths, limitations, and league-specific applications of both models, highlighting the need for standardized data collection practices and expanded contextual features to enhance xG model utility and accuracy.
Keywords
Expected Goals (xG), Soccer Analytics, Predictive Modeling, Feature Importance, SHAP Analysis
[1]. FBRef. (n.d.). xG explained. Retrieved from https://fbref.com/en/expected-goals-model-explained/
[2]. Hudl StatsBomb. (2024). What are expected goals (xG)?. Retrieved from https://statsbomb.com/soccer-metrics/expected-goals-xg-explained/
[3]. Harkness, C. (2020). What is expected goals (xG) in football and how is it calculated? Total Football Analysis. Retrieved from: https://statsbomb.com/soccer-metrics/expected-goals-xg-explained/.
[4]. Mead, J., O'Hare, A., & McMenemy, P. (2023). Expected goals in football: Improving model performance and demonstrating value. PloS one, 18(4), e0282295. Retrieved from https://doi.org/10.1371/journal.pone.0282295
[5]. Bornn, L., Fernández, J., Cervone, D., & Goldsberry, K. (2018). Soccer analytics: Unraveling the complexity of the beautiful game. INFORMS Journal on Applied Analytics, 48(4), 338-349. Retrieved from https://doi.org/10.1111/j.1740-9713.2018.01146.x
[6]. Roccetti, M., Berveglieri, F., & Cappiello, G. (2024). Football data analysis: The predictive power of expected goals (xG). https://doi.org/10.13140/RG.2.2.34493.27365/14
[7]. Spearman, W. (2018). Beyond expected goals. MIT Sloan Sports Analytics Conference. Retrieved from https://www.researchgate.net/publication/32713984_Beyond_Expected_Goals
[8]. Gieparda, M. (2023). Football Data Analytics — Let’s start. Medium. Retrieved from https://medium.com/@DataThinker/football-data-analytics-lets-start-4ad1a28ee357
[9]. Tiippana, T. (2020). How accurately does the expected goals model reflect goalscoring and success in football? Aaltodoc. Retrieved from https://aaltodoc.aalto.fi/server/api/core/bitstreams/43d75b97-553d-40eb-8aaf-79dadbe54514/content
[10]. Decroos, T., Bransen, L., Van Haaren, J., & Davis, J. (2019). Actions speak louder than goals: Valuing player actions in soccer. Proceedings of the 25th ACM SIGKDD International Conference on Knowledge Discovery & Data Mining, 1851-1861. Retrieved from https://doi.org/10.1145/3292500.3330758
[11]. Beatthebookie2017. (2024). Comparing the predictive power of different xG data providers. Retrieved from https://beatthebookie.blog/2024/01/06/comparing-the-predictive-power-of-different-xg-data-providers/
[12]. Orest. (2021, December 15). xG model comparison—Understat, StatsBomb & Opta. Retrieved from https://tacticsnotantics.org/statistical-models-and-analyses/xg-model-comparison/
Cite this article
Fu,S. (2024). Comparative Analysis of Expected Goals Models: Evaluating Predictive Accuracy and Feature Importance in European Soccer. Applied and Computational Engineering,113,36-45.
Data availability
The datasets used and/or analyzed during the current study will be available from the authors upon reasonable request.
Disclaimer/Publisher's Note
The statements, opinions and data contained in all publications are solely those of the individual author(s) and contributor(s) and not of EWA Publishing and/or the editor(s). EWA Publishing and/or the editor(s) disclaim responsibility for any injury to people or property resulting from any ideas, methods, instructions or products referred to in the content.
About volume
Volume title: Proceedings of the 2nd International Conference on Machine Learning and Automation
© 2024 by the author(s). Licensee EWA Publishing, Oxford, UK. This article is an open access article distributed under the terms and
conditions of the Creative Commons Attribution (CC BY) license. Authors who
publish this series agree to the following terms:
1. Authors retain copyright and grant the series right of first publication with the work simultaneously licensed under a Creative Commons
Attribution License that allows others to share the work with an acknowledgment of the work's authorship and initial publication in this
series.
2. Authors are able to enter into separate, additional contractual arrangements for the non-exclusive distribution of the series's published
version of the work (e.g., post it to an institutional repository or publish it in a book), with an acknowledgment of its initial
publication in this series.
3. Authors are permitted and encouraged to post their work online (e.g., in institutional repositories or on their website) prior to and
during the submission process, as it can lead to productive exchanges, as well as earlier and greater citation of published work (See
Open access policy for details).