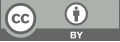
The Role of Artificial Intelligence in Modern Finance: Current Applications and Future Prospects
- 1 College of Professional Studies, Northeastern University, Boston, Massachusetts, United States of America
* Author to whom correspondence should be addressed.
Abstract
The finance industry has been radically re-invented by AI and is now providing novel solutions to a data-intensive and ever more sophisticated marketplace. In this article, AI applications in finance — portfolio management, risk management, and algorithmic trading are discussed in depth. The overview discusses some emerging techniques (including deep learning, synthetic data generation and deep reinforcement learning) and problems (such as interpretability, regulation compliance, algorithmic bias). The paper synthesizes existing research, outlining the limitations and prospects for AI technologies to enable financial decision-making, risk-taking and trading. The paper will address the promise and challenges of AI in finance, to contribute to the growing literature and inform scholars, practitioners and policymakers, leading to a more effective and resilient financial ecosystem.
Keywords
AI, Finance, portfolio management, risk management, algorithmic trading
[1]. Cao, L., & Longbing Cao, University of Technology Sydney, S. (2022). AI in finance: Challenges, techniques, and opportunities. ACM Computing Surveys.
[2]. John W. Goodell & Satish K & Weng Marc Lim & Debidutta Pattnaik. (2021). Artificial Intelligence and machine learning in finance: Identifying foundations, themes, and research clusters from Bibliometric analysis. Journal of Behavioral and Experimental Finance. https://doi.org/10.1016/j.jbef.2021.100577.
[3]. Ozkaya, I. (2020). What is really different in engineering AI-enabled systems?. IEEE software, 37(4), 3-6.
[4]. Ashta, A., & Herrmann, H. (2021). Artificial intelligence and fintech: An overview of opportunities and risks for banking, investments, and microfinance. Strategic Change, 30(3), 211-222.
[5]. Pallathadka, H., Ramirez-Asis, E. H., Loli-Poma, T. P., Kaliyaperumal, K., Ventayen, R. J. M., & Naved, M. (2023). Applications of artificial intelligence in business management, e-commerce and finance. Materials Today: Proceedings, 80, 2610-2613.
[6]. Bi, S., & Lian, Y. (2024). Advanced Portfolio Management in Finance using Deep Learning and Artificial Intelligence Techniques: Enhancing Investment Strategies through Machine Learning Models. Journal of Artificial Intelligence Research, 4(1), 233-298.
[7]. Kasaraneni, R. K. (2021). AI-Enhanced Portfolio Optimization: Balancing Risk and Return with Machine Learning Models. African Journal of Artificial Intelligence and Sustainable Development, 1(1), 219-265.
[8]. Sánchez-Fernández, Á., Díez-González, J., & Perez, H. (2024, October). Artificial Intelligence in Portfolio Selection Problem: A Review and Future Perspectives. In International Conference on Hybrid Artificial Intelligence Systems (pp. 252-264). Cham: Springer Nature Switzerland.
[9]. Arsic, V. B. (2021). Challenges of financial risk management: AI applications. Management: Journal of Sustainable Business and Management Solutions in Emerging Economies, 26(3), 27-34.
[10]. Schuett, J. (2024). Risk management in the artificial intelligence act. European Journal of Risk Regulation, 15(2), 367-385.
[11]. Habbal, A., Ali, M. K., & Abuzaraida, M. A. (2024). Artificial Intelligence Trust, risk and security management (AI trism): Frameworks, applications, challenges and future research directions. Expert Systems with Applications, 240, 122442.
[12]. Namperumal, G., Selvaraj, A., & Surampudi, Y. (2022). Synthetic Data Generation for Credit Scoring Models: Leveraging AI and Machine Learning to Improve Predictive Accuracy and Reduce Bias in Financial Services. Journal of Artificial Intelligence Research, 2(1), 168-204.
[13]. Gunnarsson, B. R., Vanden Broucke, S., Baesens, B., Óskarsdóttir, M., & Lemahieu, W. (2021). Deep learning for credit scoring: Do or don’t?. European Journal of Operational Research, 295(1), 292-305.
[14]. Lin, A. K. (2024). The AI Revolution in Financial Services: Emerging Methods for Fraud Detection and Prevention. Jurnal Galaksi, 1(1), 43-51.
[15]. Cohen, G. (2022). Algorithmic trading and financial forecasting using advanced artificial intelligence methodologies. Mathematics, 10(18), 3302.
[16]. Soundarapandiyan, R., Sivathapandi, P., & Surampudi, Y. (2022). Enhancing Algorithmic Trading Strategies with Synthetic Market Data: AI/ML Approaches for Simulating High-Frequency Trading Environments. Journal of Artificial Intelligence Research and Applications, 2(1), 333-373.
[17]. Théate, T., & Ernst, D. (2021). An application of deep reinforcement learning to algorithmic trading. Expert Systems with Applications, 173, 114632.
Cite this article
Wu,S. (2024). The Role of Artificial Intelligence in Modern Finance: Current Applications and Future Prospects. Applied and Computational Engineering,120,17-22.
Data availability
The datasets used and/or analyzed during the current study will be available from the authors upon reasonable request.
Disclaimer/Publisher's Note
The statements, opinions and data contained in all publications are solely those of the individual author(s) and contributor(s) and not of EWA Publishing and/or the editor(s). EWA Publishing and/or the editor(s) disclaim responsibility for any injury to people or property resulting from any ideas, methods, instructions or products referred to in the content.
About volume
Volume title: Proceedings of the 5th International Conference on Signal Processing and Machine Learning
© 2024 by the author(s). Licensee EWA Publishing, Oxford, UK. This article is an open access article distributed under the terms and
conditions of the Creative Commons Attribution (CC BY) license. Authors who
publish this series agree to the following terms:
1. Authors retain copyright and grant the series right of first publication with the work simultaneously licensed under a Creative Commons
Attribution License that allows others to share the work with an acknowledgment of the work's authorship and initial publication in this
series.
2. Authors are able to enter into separate, additional contractual arrangements for the non-exclusive distribution of the series's published
version of the work (e.g., post it to an institutional repository or publish it in a book), with an acknowledgment of its initial
publication in this series.
3. Authors are permitted and encouraged to post their work online (e.g., in institutional repositories or on their website) prior to and
during the submission process, as it can lead to productive exchanges, as well as earlier and greater citation of published work (See
Open access policy for details).