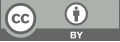
Hardware Implementation of Convolutional Neural Networks
- 1 Detroit Green Technology Institute, HuBei University of Technology, WuHan 430070, China
* Author to whom correspondence should be addressed.
Abstract
Convolutional Neural Networks (CNNs) have broad application prospects in computer vision, image processing, and other fields. However, their characteristics, such as high computational speed and large data volume, pose significant challenges for hardware implementation. This project aims to improve computational efficiency and reduce energy consumption by summarizing hardware-based convolutional neural network algorithms to meet real-time requirements. Additionally, it will summarize research methods for accelerating convolutional computation, pooling, and fully connected layers using different hardware platforms such as ASIC, FPGA, and GPU. This paper focuses on optimizing data flow, parallel processing, and memory architecture to reduce computation latency and energy consumption. To ensure network accuracy, methods such as quantization and pruning are employed to reduce model size and computational complexity. Literature indicates that custom hardware designs for convolutional neural networks significantly enhance performance and energy efficiency compared to traditional software implementations. This review aims to provide an efficient hardware acceleration method for practical deep learning algorithms and promote development in fields such as smart terminals and edge computing.
Keywords
Convolutional Neural Networks, Hardware Implementation, FPGA, GPU
[1]. Tan, Y. (2024). Image classification VGG16 hardware implementation and sensor-based side channel security research. Heilongjiang University.
[2]. Ji, M. (2024). Research on convolutional neural network accelerators based on configurable parallelism and automatic optimization of network architecture. Jilin University.
[3]. Shuen, Z., Gong, Z., & Zhao, D. (2024).Traffic signs and markings recognition based on lightweight convolutional neural network. The Visual Computer, 40(2), 559-570.
[4]. Yin, S., Ouyang, P., Tang, S., et al. (2018). Thinker: Reconfigurable hybrid neural network computing chip. Artificial Intelligence, (02), 34-45.
[5]. Chung, E., et al. (2018). Serving DNNs in real time at datacenter scale with Project Brainwave. IEEE Micro, 38(2), 8-20.
[6]. Chung, C.-C., Liang, Y.-P., Chang, Y.-C., & Chang, C.-M. (2023). A binary weight convolutional neural network hardware accelerator for analysis faults of the CNC machinery on FPGA. In 2023 International VLSI Symposium on Technology, Systems and Applications (VLSI-TSA/VLSI-DAT) (pp. 1-4).
[7]. Markov, N. G., Zoev, I. V., & Mytsko, E. A. (2022). FPGA hardware implementation of the YOLO subclass convolutional neural network model in computer vision systems. In 2022 International Siberian Conference on Control and Communications (SIBCON) (pp. 1-4).
[8]. Akimova, J. E., & Budanov, D. O. (2023). Hardware implementation of a convolutional neural network. In 2023 International Conference on Electrical Engineering and Photonics (EExPolytech) (pp. 72-75).
[9]. Olegovich, T. M., Maksimovich, A. D., & Evgenievna, L. E. (2021). Hardware implementation of classical and bipolar morphological models for convolutional neural network. In 2021 International Conference Engineering and Telecommunication (En&T) (pp. 1-5).
[10]. Elbtity, M. E., Son, H.-W., Lee, D.-Y., & Kim, H. (2020). High speed, approximate arithmetic based convolutional neural network accelerator. In 2020 International SoC Design Conference (ISOCC) (pp. 71-72).
[11]. Sowah, R. A., Apeadu, K., Gatsi, F., Ampadu, K. O., & Mensah, B. S. (2020). Hardware module design and software implementation of multisensor fire detection and notification system using fuzzy logic and convolutional neural networks (CNNs). Journal of Engineering, 2020, Article ID 3645729.
Cite this article
Guo,Y. (2025). Hardware Implementation of Convolutional Neural Networks. Applied and Computational Engineering,121,9-13.
Data availability
The datasets used and/or analyzed during the current study will be available from the authors upon reasonable request.
Disclaimer/Publisher's Note
The statements, opinions and data contained in all publications are solely those of the individual author(s) and contributor(s) and not of EWA Publishing and/or the editor(s). EWA Publishing and/or the editor(s) disclaim responsibility for any injury to people or property resulting from any ideas, methods, instructions or products referred to in the content.
About volume
Volume title: Proceedings of the 5th International Conference on Signal Processing and Machine Learning
© 2024 by the author(s). Licensee EWA Publishing, Oxford, UK. This article is an open access article distributed under the terms and
conditions of the Creative Commons Attribution (CC BY) license. Authors who
publish this series agree to the following terms:
1. Authors retain copyright and grant the series right of first publication with the work simultaneously licensed under a Creative Commons
Attribution License that allows others to share the work with an acknowledgment of the work's authorship and initial publication in this
series.
2. Authors are able to enter into separate, additional contractual arrangements for the non-exclusive distribution of the series's published
version of the work (e.g., post it to an institutional repository or publish it in a book), with an acknowledgment of its initial
publication in this series.
3. Authors are permitted and encouraged to post their work online (e.g., in institutional repositories or on their website) prior to and
during the submission process, as it can lead to productive exchanges, as well as earlier and greater citation of published work (See
Open access policy for details).