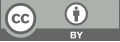
Review of the Development of Machine Learning Application in Tropical Cyclone Prediction
- 1 school of information science, Guangdong University of Finance & Economics, Guang Zhou, Guang Dong, China, 510320
* Author to whom correspondence should be addressed.
Abstract
Nowadays, along with the trend that more and more devastating tropical cyclones are happening all over the world, people’s are facing serious threat. Since traditional models have trouble giving more accurate prediction results, ML models are introduced to provide a more effective way. This overview briefly summarizes the history of ML and the causes of TCs, giving some algorithms of ML that were applied to TC prediction. It also included two actual examples of ML methods that had made a success on predicting TCs. Despite the fact that challenges exist in data quality and computational resources, machine learning models have proven to have huge potential in improving the accuracy and efficiency of tropical cyclone predictions. What’s more, this overview provides some possible prospects of the field, too, including model optimization, risk assessment, and interdisciplinary collaboration. These urgently-needed advancements are essential for improving the resilience of coastal rigions abilities to deal with TCs and the disaster caused by it.
Keywords
Machine learning, Tropical cyclone, Prediction, Algorithms
[1]. Lacheheb, Miloud, et al. “Impact of Tropical Cyclones on Fishing Activity in the Philippines.” Fisheries Research, vol. 281, Jan. 2025, p. 107221, https://doi.org/10.1016/j.fishres.2024.107221.
[2]. Wang, Zhen, et al. “A Review on the Application of Machine Learning Methods in Tropical Cyclone Forecasting.” Frontiers in Earth Science, vol. 10, 2022, https://doi.org/10.3389/feart.2022.902596.
[3]. Chen, Rui, et al. “Machine Learning in Tropical Cyclone Forecast Modeling: A Review.” Atmosphere, vol. 11, no. 7, 2020, https://doi.org/10.3390/atmos11070676.
[4]. Koyama, Hajime. “Machine Learning Application in Otology.” Auris Nasus Larynx, vol. 51, no. 4, Aug. 2024, pp. 666–73, https://doi.org/10.1016/j.anl.2024.04.003.
[5]. Chen, Guangqi. “Prospects, Challenges and Guidelines for Practical Deep Learning in Geoengineering.” Intelligent Geoengineering, vol. 1, no. 1, Dec. 2024, pp. 19–29, https://doi.org/10.1016/j.ige.2024.10.001.
[6]. Tang, Brian H., et al. “Recent Advances in Research on Tropical Cyclogenesis.” Tropical Cyclone Research and Review, vol. 9, no. 2, June 2020, pp. 87–105, https://doi.org/10.1016/j.tcrr.2020.04.004.
[7]. Pillay, Micheal T., and Jennifer M. Fitchett. “On the Conditions of Formation of Southern Hemisphere Tropical Cyclones.” Weather and Climate Extremes, vol. 34, Dec. 2021, p. 100376, https://doi.org/10.1016/j.wace.2021.100376.
[8]. Li, Guoyou, et al. “Trends and Periodicities of Tropical Cyclone Frequencies and the Correlations with Ocean Drivers.” Journal of Marine Science and Engineering, vol. 12, no. 10, 2024, https://doi.org/10.3390/jmse12101707.
[9]. Lindemann, Benjamin, et al. “A Survey on Long Short-Term Memory Networks for Time Series Prediction.” 14th CIRP Conference on Intelligent Computation in Manufacturing Engineering, 15-17 July 2020, vol. 99, Jan. 2021, pp. 650–55, https://doi.org/10.1016/j.procir.2021.03.088.
[10]. Abbasi, Mahmoud, et al. “Deep Learning for Network Traffic Monitoring and Analysis (NTMA): A Survey.” Computer Communications, vol. 170, Mar. 2021, pp. 19–41, https://doi.org/10.1016/j.comcom.2021.01.021.
[11]. Lian, Jie, et al. “A Novel Deep Learning Approach for Tropical Cyclone Track Prediction Based on Auto-Encoder and Gated Recurrent Unit Networks.” Applied Sciences, vol. 10, no. 11, 2020, https://doi.org/10.3390/app10113965.
[12]. Sahoo, Bishnupriya, and Prasad K. Bhaskaran. “Prediction of Storm Surge and Coastal Inundation Using Artificial Neural Network – A Case Study for 1999 Odisha Super Cyclone.” Weather and Climate Extremes, vol. 23, Mar. 2019, p. 100196, https://doi.org/10.1016/j.wace.2019.100196.
Cite this article
Liu,Y. (2025). Review of the Development of Machine Learning Application in Tropical Cyclone Prediction. Applied and Computational Engineering,121,72-77.
Data availability
The datasets used and/or analyzed during the current study will be available from the authors upon reasonable request.
Disclaimer/Publisher's Note
The statements, opinions and data contained in all publications are solely those of the individual author(s) and contributor(s) and not of EWA Publishing and/or the editor(s). EWA Publishing and/or the editor(s) disclaim responsibility for any injury to people or property resulting from any ideas, methods, instructions or products referred to in the content.
About volume
Volume title: Proceedings of the 5th International Conference on Signal Processing and Machine Learning
© 2024 by the author(s). Licensee EWA Publishing, Oxford, UK. This article is an open access article distributed under the terms and
conditions of the Creative Commons Attribution (CC BY) license. Authors who
publish this series agree to the following terms:
1. Authors retain copyright and grant the series right of first publication with the work simultaneously licensed under a Creative Commons
Attribution License that allows others to share the work with an acknowledgment of the work's authorship and initial publication in this
series.
2. Authors are able to enter into separate, additional contractual arrangements for the non-exclusive distribution of the series's published
version of the work (e.g., post it to an institutional repository or publish it in a book), with an acknowledgment of its initial
publication in this series.
3. Authors are permitted and encouraged to post their work online (e.g., in institutional repositories or on their website) prior to and
during the submission process, as it can lead to productive exchanges, as well as earlier and greater citation of published work (See
Open access policy for details).