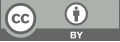
A research agenda of AI-based analog circuit fault diagnosis with bibliometric analysis
- 1 The University of Edinburgh, Old College, South Bridge, Edinburgh EH8 9YL
* Author to whom correspondence should be addressed.
Abstract
Analog circuit fault identification is crucial for preserving regular operation. Methods based on artificial intelligence (AI) provide excellent accuracy in fault identification. AI-based techniques have been used widely in recent years, displaying remarkable variety and complexity. Therefore, in order to have a clearer understanding of the issues in this area, it is necessary to summarise and classify the techniques. In this article, various AI-based fault detection techniques are displayed, and bibliometrics is used to show the trend and citations. The relationships, significant writers, and journals will first be discussed in this article, after which some key pieces of literature will be displayed. The key findings and conclusions, clarification of the current issues, and a summary of the present and foreseeable research trends are all included in the last section.
Keywords
analogy circuit, fault diagnosis, deep learning, machine learning, bibliometric analysis.
[1]. Xu X, Qiao X, Zhang N, Feng J, Wang X. Review of intelligent fault diagnosis for permanent magnet synchronous motors in electric vehicles. Vol. 12, Advances in Mechanical Engineering. SAGE Publications Inc.; 2020.
[2]. Afacan E, Lourenço N, Martins R, Dündar G. Review: Machine learning techniques in analog/RF integrated circuit design, synthesis, layout, and test. Integration. 2021 Mar 1;77:113–30.
[3]. Lang W, Hu Y, Gong C, Zhang X, Xu H, Deng J. Artificial Intelligence-Based Technique for Fault Detection and Diagnosis of EV Motors: A Review. IEEE Transactions on Transportation Electrification. 2022 Mar 1;8(1):384–406.
[4]. Peco Chacón AM, Segovia Ramírez I, García Márquez FP. State of the Art of Artificial Intelligence Applied for False Alarms in Wind Turbines. Archives of Computational Methods in Engineering. Springer Science and Business Media B.V.; 2021.
[5]. Dieste-Velasco MI. Application of a pattern-recognition neural network for detecting analog electronic circuit faults. Mathematics. 2021 Dec 1;9(24).
[6]. Ji L, Fu C, Sun W. Soft Fault Diagnosis of Analog Circuits Based on a ResNet with Circuit Spectrum Map. IEEE Transactions on Circuits and Systems I: Regular Papers. 2021 Jul 1;68(7):2841–9.
[7]. Zhang C, Zha D, Wang L, Mu N. A novel analog circuit soft fault diagnosis method based on convolutional neural network and backward difference. Symmetry (Basel). 2021 Jun 1;13(6).
[8]. Moezi A, Kargar SM. Simultaneous fault localization and detection of analog circuits using deep learning approach. Computers and Electrical Engineering. 2021 Jun 1;92.
[9]. Gao T, Yang J, Jiang S. A novel fault diagnosis method for analog circuits with noise immunity and generalization ability. Neural Comput Appl. 2021 Aug 1;33(16):10537–50.
[10]. Aizenberg I, Belardi R, Bindi M, Grasso F, Manetti S, Luchetta A, et al. A neural network classifier with multi-valued neurons for analog circuit fault diagnosis. Electronics (Switzerland). 2021 Feb 1;10(3):1–18.
[11]. Shokrolahi SM, Karimiziarani M. A deep network solution for intelligent fault detection in analog circuit. Analog Integr Circuits Signal Process. 2021 Jun 1;107(3):597–604.
[12]. Shi J, Deng Y, Wang Z. Analog circuit fault diagnosis based on density peaks clustering and dynamic weight probabilistic neural network. Neurocomputing. 2020 Sep 24;407:354–65.
[13]. Wang N. The analysis of electronic circuit fault diagnosis based on neural network data fusion algorithm. Symmetry (Basel). 2020 Mar 1;12(3).
[14]. Wang H. Fault diagnosis of analog circuit based on wavelet transform and neural network. Archives of Electrical Engineering. 2020;69(1):175–85.
[15]. Lin Q, Chen S, Lin CM. Parametric fault diagnosis based on fuzzy cerebellar model neural networks. IEEE Transactions on Industrial Electronics. 2019 Oct 1;66(10):8104–15.
[16]. Khanlari M, Ehsanian M. An Improved KFCM Clustering Method Used for Multiple Fault Diagnosis of Analog Circuits. Circuits Syst Signal Process. 2017 Sep 1;36(9):3491–513.
[17]. Xu-sheng G, Wen-ming G, Zhe D, Wei-dong L. Research on WNN soft fault diagnosis for analog circuit based on adaptive UKF algorithm. Applied Soft Computing Journal. 2017 Jan 1;50:252–9.
[18]. Liang H, Zhu Y, Zhang D, Chang L, Lu Y, Zhao X, et al. Analog circuit fault diagnosis based on support vector machine classifier and fuzzy feature selection. Electronics (Switzerland). 2021 Jun 2;10(12).
[19]. Wang L, Tian H, Zhang H. Soft fault diagnosis of analog circuits based on semi-supervised support vector machine. Analog Integr Circuits Signal Process. 2021
[20]. Su X, Cao C, Zeng X, Feng Z, Shen J, Yan X, et al. Application of DBN and GWO-SVM in analog circuit fault diagnosis. Sci Rep. 2021 Dec 1;11(1).
[21]. Guo S, Wu B, Zhou J, Li H, Su C, Yuan Y, et al. An analog circuit fault diagnosis method based on circle model and extreme learning machine. Applied Sciences (Switzerland). 2020 Apr 1;10(7).
[22]. Zhang T, Li T. A novel approach of analog circuit fault diagnosis utilizing RFT noise estimation. Analog Integr Circuits Signal Process. 2019 Mar 15;98(3):517–26.
[23]. Zhang C, He Y, Yuan L, Xiang S. A multiple heterogeneous kernel RVM approach for analog circuit fault prognostic. Cluster Comput. 2019 Mar 1;22:3849–61.
[24]. Yuan X, Liu Z, Miao Z, Zhao Z, Zhou F, Song Y. Fault diagnosis of analog circuits based on ihpso optimized support vector machine. IEEE Access. 2019;7:137945–58.
[25]. Khemani V, Azarian MH, Pecht MG. Learnable Wavelet Scattering Networks: Applications to Fault Diagnosis of Analog Circuits and Rotating Machinery. Electronics (Switzerland). 2022 Feb 1;11(3).
[26]. Liu Z, Liu X, Xie S, Wang J, Zhou X. A Novel Fault Diagnosis Method for Analog Circuits Based on Multi-Input Deep Residual Networks with an Improved Empirical Wavelet Transform. Applied Sciences (Switzerland). 2022 Feb 1;12(3).
[27]. Wang Y, Ma Y, Cui S, Yan Y. A novel approach of feature extraction for analog circuit fault diagnosis based on WPD-LLE-CSA. Journal of Electrical Engineering and Technology. 2018 Nov 1;13(6):2485–92.
[28]. He Y, Tan Y, Sun Y. Wavelet neural network approach for fault diagnosis of analogue circuits. IEE Proceedings: Circuits, Devices and Systems. 2004 Aug;151(4):379–84.
[29]. Cannas B, Fanni A, Manetti S, Montisci A, Piccirilli MC. Neural network-based analog fault diagnosis using testability analysis. Neural Comput Appl. 2004;13(4):288–98.
[30]. Yuan L, He Y, Huang J, Sun Y. A new neural-network-based fault diagnosis approach for analog circuits by using kurtosis and entropy as a preprocessor. IEEE Trans Instrum Meas. 2010 Mar;59(3):586–95.
[31]. Zhao G, Liu X, Zhang B, Liu Y, Niu G, Hu C. A novel approach for analog circuit fault diagnosis based on Deep Belief Network. Measurement (Lond). 2018 Jun 1;121:170–8.
[32]. Tan Y, Sun Y, Yin X. Analog fault diagnosis using S-transform preprocessor and a QNN classifier. Measurement (Lond). 2013;46(7):2174–83.
[33]. Xiao Y, He Y. A novel approach for analog fault diagnosis based on neural networks and improved kernel PCA. Neurocomputing. 2011 Mar;74(7):1102–15.
[34]. Alippi C, Catelani M, Fort A, Mugnaini M. SBT soft fault diagnosis in analog electronic circuits: A sensitivity-based approach by randomized algorithms. IEEE Trans Instrum Meas. 2002 Oct;51(5):1116–25.
[35]. Catelani M, Fort A. Fault diagnosis of electronic analog circuits using a radial basis function network classifier [Internet]. Vol. 28, Measurement. 2000. Available from: www.elsevier.com/locate/measurement
[36]. Aminian F, Member S, Aminian M, Collins HW. Analog Fault Diagnosis of Actual Circuits Using Neural Networks. Vol. 51, IEEE TRANSACTIONS ON INSTRUMENTATION AND MEASUREMENT. 2002.
Cite this article
Zhou,J. (2023). A research agenda of AI-based analog circuit fault diagnosis with bibliometric analysis. Applied and Computational Engineering,6,325-333.
Data availability
The datasets used and/or analyzed during the current study will be available from the authors upon reasonable request.
Disclaimer/Publisher's Note
The statements, opinions and data contained in all publications are solely those of the individual author(s) and contributor(s) and not of EWA Publishing and/or the editor(s). EWA Publishing and/or the editor(s) disclaim responsibility for any injury to people or property resulting from any ideas, methods, instructions or products referred to in the content.
About volume
Volume title: Proceedings of the 3rd International Conference on Signal Processing and Machine Learning
© 2024 by the author(s). Licensee EWA Publishing, Oxford, UK. This article is an open access article distributed under the terms and
conditions of the Creative Commons Attribution (CC BY) license. Authors who
publish this series agree to the following terms:
1. Authors retain copyright and grant the series right of first publication with the work simultaneously licensed under a Creative Commons
Attribution License that allows others to share the work with an acknowledgment of the work's authorship and initial publication in this
series.
2. Authors are able to enter into separate, additional contractual arrangements for the non-exclusive distribution of the series's published
version of the work (e.g., post it to an institutional repository or publish it in a book), with an acknowledgment of its initial
publication in this series.
3. Authors are permitted and encouraged to post their work online (e.g., in institutional repositories or on their website) prior to and
during the submission process, as it can lead to productive exchanges, as well as earlier and greater citation of published work (See
Open access policy for details).