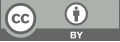
From sidelines to scoreboards: Regression modelling for predicting NBA game outcomes
- 1 Appleby College, Oakville, L6K 3P1, Canada
- 2 Shanghai Experimental School, Shanghai, 200011, China
- 3 Philips Exeter Academy, Exeter, 03833, United States
* Author to whom correspondence should be addressed.
Abstract
This research paper examines the diverse factors that affect score difference between teams in the NBA scene. With this intent, by utilizing data from the 2012-13 regular season, this research aims to develop a predictive model that can forecast the score difference between teams for the last 50 games of the season. Additionally, the same model can be expanded and used for many different seasons of NBA data. To accomplish this, the methodology implemented first involved a data collection process, where many years of injury data and NBA season data were gathered. Next, extensive cleaning was done so all the variable names matched, and only significant information remained. Then, by merging the injury data with data from the 2012-2013 NBA season, a larger, more comprehensive file was created. As last, through the use of regression modelling, a base model was created. In addition, factors impacting the score difference were considered and adjusted the model accordingly. To validate the final model’s prediction, actual score differences in the last 50 games will be compared to the differences given by the model, with statistical measurement methods to quantify the accuracy. By doing so, this research hopes to provide a more valuable system that produce insight towards basketball sports betting.
Keywords
Regression Modelling, Statistics, NBA, Sports Gambling, Point Spread
[1]. Matheson, V. (2021) 'An overview of the economics of sports gambling and an introduction to the symposium, ' Eastern Economic Journal, 47(1), pp. 1–8. https://doi.org/10.1057/s41302-020-00182-4.
[2]. Levitt, Steven. (2004). Why are Gambling Markets Organized so Differently from Financial Markets?. Economic Journal. 114. 223-246. 10.1111/j.1468-0297.2004.00207.x.
[3]. Feddersen, A., Humphreys, B. R. and Soebbing, B. P. (2013) 'Sentiment bias in National Basketball Association Betting, ' West Virginia University [Preprint]. http://busecon.wvu.edu/phd_economics/pdf/13-03.pdf.
[4]. Weinbach, Andrew & Paul, Rodney. (2008). Price Setting in the NBA Gambling Market: Tests of the Levitt Model of Sportsbook Behavior. International Journal of Sport Finance. 3. 137-145.
[5]. R Core Team (2024). _R: A Language and Environment for Statistical Computing_. R Foundation for Statistical Computing, Vienna, Austria. <https://www.R-project.org/>.
[6]. Fernando, J. (2024) R-Squared: Definition, Formula, Uses, and Limitations. https://www.investopedia.com/terms/r/r-squared.asp.
[7]. O’Brien, T.E. and Silcox, J.W. (2024) 'Nonlinear Regression Modelling: A Primer with Applications and Caveats, ' Bulletin of Mathematical Biology, 86(4). https://doi.org/10.1007/s11538-024-01274-4.
[8]. Schneider, A., Hommel, G. and Blettner, M. (2010) 'Linear Regression analysis, ' Deutsches Ärzteblatt International [Preprint]. https://doi.org/10.3238/arztebl.2010.0776.
[9]. Courneya, K. S., and Carron, A. V. (1992). The Home Advantage In Sport Competitions: A Literature Review. Journal of Sport and Exercise Psychology 14, 1, 13-27, available from: <https://doi.org/10.1123/jsep.14.1.13> [Accessed 23 August 2024]
[10]. NBA Injuries from 2010-2020 (2020). https://www.kaggle.com/datasets/ghopkins/nba-injuries-2010-2018.
Cite this article
Wang,Y.;Ji,H.;Dai,D. (2025). From sidelines to scoreboards: Regression modelling for predicting NBA game outcomes. Applied and Computational Engineering,131,60-71.
Data availability
The datasets used and/or analyzed during the current study will be available from the authors upon reasonable request.
Disclaimer/Publisher's Note
The statements, opinions and data contained in all publications are solely those of the individual author(s) and contributor(s) and not of EWA Publishing and/or the editor(s). EWA Publishing and/or the editor(s) disclaim responsibility for any injury to people or property resulting from any ideas, methods, instructions or products referred to in the content.
About volume
Volume title: Proceedings of the 2nd International Conference on Machine Learning and Automation
© 2024 by the author(s). Licensee EWA Publishing, Oxford, UK. This article is an open access article distributed under the terms and
conditions of the Creative Commons Attribution (CC BY) license. Authors who
publish this series agree to the following terms:
1. Authors retain copyright and grant the series right of first publication with the work simultaneously licensed under a Creative Commons
Attribution License that allows others to share the work with an acknowledgment of the work's authorship and initial publication in this
series.
2. Authors are able to enter into separate, additional contractual arrangements for the non-exclusive distribution of the series's published
version of the work (e.g., post it to an institutional repository or publish it in a book), with an acknowledgment of its initial
publication in this series.
3. Authors are permitted and encouraged to post their work online (e.g., in institutional repositories or on their website) prior to and
during the submission process, as it can lead to productive exchanges, as well as earlier and greater citation of published work (See
Open access policy for details).