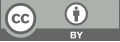
Research on Stock Price Rise and Fall Prediction Based on Optimization Random Forest
- 1 Hebei University of Economics and Business, No.47 Xuefu Road, Xinhua District, Shijiazhuang City, Hebei Province, 050061
* Author to whom correspondence should be addressed.
Abstract
Random forest algorithm is an effective machine learning algorithm in stock return classification prediction, with high accuracy, but it has problems such as parameter optimization defects and difficulty in feature selection. To this end, based on the traditional random forest algorithm, a new algorithm is proposed by combining the feature selection particle swarm algorithm with the parameter grid search algorithm - the particle swarm parameter grid search random forest algorithm. Using particle swarm optimization algorithm for feature selection of input data, reducing the dimensionality of input data by removing redundant features, and introducing grid search algorithm to optimize some parameters of random forest, not only reduces the computational complexity of random forest algorithm, but also improves the classification and prediction accuracy of random forest. The experimental results were compared with the original random forest, decision tree, and support vector machine classification models, confirming that the parameter optimized random forest stock prediction model has higher accuracy and AUC values in model evaluation than other models.
Keywords
random forest, Technical indicators, Parameter optimization, Grid search, Stock price prediction
[1]. Deivendran P , G. C , Suresh B P ,et al.Analysis of Stock Price Fluctuations Accuracy using a Cloud-Based Recurrent Neural Network's Long Short-Term Memory Model[J].International Journal of Electrical and Electronics Engineering, 2023.
[2]. Osu B O , Amadi I U .A Stochastic Analysis of Stock Market Price Fluctuations for Capital Market[J].Journal of Applied Mathematics and Computation, 2023, 6.
[3]. Dong Z , Jia Y , Wang Z ,et al.Research on the Quantitative Causal Transmission of Stock Price Fluctuations of Listed Companies in the Rare Earth Industry Chain[J].International Journal of Energy Research, 2023.
[4]. Kumari B , Patnaik S , Swarnkar T .Feature selection for stock price prediction: a critical review[J].International Journal of Intelligent Enterprise, 2023.
[5]. Wang R , Shao Z , Tian W L .A Dual-View Model for Stock Price Prediction of Internet-of-Thing Enterprises[J].Journal of circuits, systems and computers, 2024, 33(1):1.1-1.17.
Cite this article
Wang,J. (2025). Research on Stock Price Rise and Fall Prediction Based on Optimization Random Forest. Applied and Computational Engineering,133,60-66.
Data availability
The datasets used and/or analyzed during the current study will be available from the authors upon reasonable request.
Disclaimer/Publisher's Note
The statements, opinions and data contained in all publications are solely those of the individual author(s) and contributor(s) and not of EWA Publishing and/or the editor(s). EWA Publishing and/or the editor(s) disclaim responsibility for any injury to people or property resulting from any ideas, methods, instructions or products referred to in the content.
About volume
Volume title: Proceedings of the 5th International Conference on Signal Processing and Machine Learning
© 2024 by the author(s). Licensee EWA Publishing, Oxford, UK. This article is an open access article distributed under the terms and
conditions of the Creative Commons Attribution (CC BY) license. Authors who
publish this series agree to the following terms:
1. Authors retain copyright and grant the series right of first publication with the work simultaneously licensed under a Creative Commons
Attribution License that allows others to share the work with an acknowledgment of the work's authorship and initial publication in this
series.
2. Authors are able to enter into separate, additional contractual arrangements for the non-exclusive distribution of the series's published
version of the work (e.g., post it to an institutional repository or publish it in a book), with an acknowledgment of its initial
publication in this series.
3. Authors are permitted and encouraged to post their work online (e.g., in institutional repositories or on their website) prior to and
during the submission process, as it can lead to productive exchanges, as well as earlier and greater citation of published work (See
Open access policy for details).