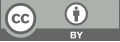
Optimizing Stock Price Prediction Based on Triangular Topology Aggregation Optimizer Using Long Short-term Memory Network
- 1 Department of Economics, University of California, Berkeley, State of California, Berkeley, 94704, USA
* Author to whom correspondence should be addressed.
Abstract
Stock price prediction has always been a complex and challenging task in the financial field. This article proposes a novel method to optimize long short-term memory networks (LSTM) through a triangular topology aggregation optimizer, in order to improve the accuracy of stock price prediction. This method combines deep learning and advanced optimization techniques, aiming to provide more effective support for investment decisions in the stock market. We first introduced an optimized LSTM model and used a dataset for predicting stock prices. By observing the changes in loss between the training and validation sets, we found that the loss value of the training set gradually decreased from 0.04 to below 0.005 and approached convergence. Meanwhile, the loss value of the validation set remained below 0.005. This indicates that the performance of this model in stock price prediction is quite outstanding during both the training and validation phases. After analyzing the test set, the results showed that the predicted stock prices of this model were very close to the actual values, and could accurately predict market trends both numerically and trendwise. In addition, the MSE (mean square error) results obtained through the evaluation indicators of the model show that the MSE of the training set is 1.938, the MSE of the testing set is 1.944, and the RMSE (root mean square error) of the training set is 1.392, and the RMSE of the validation set is 1.394. These results indicate that the evaluation metrics of the training set and the test set are not significantly different, further proving that the model has strong generalization ability and can continue to demonstrate good predictive performance on new datasets. In summary, this article proposes an effective stock price prediction method by combining a triangular topology aggregation optimizer with an LSTM model, and verifies its efficiency and practicality in the training, validation, and testing stages. This type of research not only provides new ideas for analyzing the stock market, but also provides strong support for investors to make more reasonable decisions in a dynamic market environment.
Keywords
Triangle topology aggregation optimizer, LSTM, Stock price
[1]. Jiyang C ,Sunil T ,Djebbouri K , et al.Forecasting Bitcoin prices using artificial intelligence: Combination of ML, SARIMA, and Facebook Prophet models[J].Technological Forecasting Social Change,2024,198122938-.
[2]. Harish K ,Sudhir S ,P. N , et al.A two level ensemble classification approach to forecast bitcoin prices[J].Kybernetes,2023,52(11):5041-5067.
[3]. Ruchi G ,E. J N .Metaheuristic Assisted Hybrid Classifier for Bitcoin Price Prediction[J].Cybernetics and Systems,2023,54(7):1037-1061.
[4]. Tyson M .Use TensorFlow to predict Bitcoin prices[J].InfoWorld.com,2023.
[5]. Sina F .Designing a forecasting assistant of the Bitcoin price based on deep learning using market sentiment analysis and multiple feature extraction[J].Soft Computing,2023,27(24):18803-18827.
[6]. Moinak M ,B. D V ,Michael F .Quantifying the asymmetric information flow between Bitcoin prices and electricity consumption[J].Finance Research Letters,2023,57.
[7]. Xiangling W ,Shusheng D .The impact of the Bitcoin price on carbon neutrality: Evidence from futures markets[J].Finance Research Letters,2023,56.
[8]. W. J G ,Sami J B ,Foued S , et al. Explainable artificial intelligence modeling to forecast bitcoin prices[J].International Review of Financial Analysis,2023,88.
[9]. Brahim G ,Sahbi M N ,Jean-Michel S , et al.Interactions between investors’ fear and greed sentiment and Bitcoin prices[J].North American Journal of Economics and Finance,2023,67.
[10]. Zaman S ,Yaqub U ,Saleem T .Analysis of Bitcoin’s price spike in context of Elon Musk’s Twitter activity[J].Global Knowledge Memory and Communication,2023,72(4/5):341-355.
Cite this article
Jin,Y. (2025). Optimizing Stock Price Prediction Based on Triangular Topology Aggregation Optimizer Using Long Short-term Memory Network. Applied and Computational Engineering,136,27-34.
Data availability
The datasets used and/or analyzed during the current study will be available from the authors upon reasonable request.
Disclaimer/Publisher's Note
The statements, opinions and data contained in all publications are solely those of the individual author(s) and contributor(s) and not of EWA Publishing and/or the editor(s). EWA Publishing and/or the editor(s) disclaim responsibility for any injury to people or property resulting from any ideas, methods, instructions or products referred to in the content.
About volume
Volume title: Proceedings of the 5th International Conference on Materials Chemistry and Environmental Engineering
© 2024 by the author(s). Licensee EWA Publishing, Oxford, UK. This article is an open access article distributed under the terms and
conditions of the Creative Commons Attribution (CC BY) license. Authors who
publish this series agree to the following terms:
1. Authors retain copyright and grant the series right of first publication with the work simultaneously licensed under a Creative Commons
Attribution License that allows others to share the work with an acknowledgment of the work's authorship and initial publication in this
series.
2. Authors are able to enter into separate, additional contractual arrangements for the non-exclusive distribution of the series's published
version of the work (e.g., post it to an institutional repository or publish it in a book), with an acknowledgment of its initial
publication in this series.
3. Authors are permitted and encouraged to post their work online (e.g., in institutional repositories or on their website) prior to and
during the submission process, as it can lead to productive exchanges, as well as earlier and greater citation of published work (See
Open access policy for details).