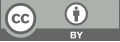
Research of target detection method YOLO
- 1 School of Computer Science and Technology, XiDian University, Xifeng Road, Xi 'an, China
* Author to whom correspondence should be addressed.
Abstract
Regression theory is used by YOLO technology to build the one stage detection technique. It merely employs a trunk CNN to predict various targets using the "feature extraction-direct regression" method. In comparison to previous algorithms, it detects things much faster and with far higher accuracy. Researchers have become interested in the YOLO model because it is widely employed in sectors such as autonomous driving, camera display, video surveillance, vehicle identification, face recognition, remote sensing satellite, infrared detection, and others. In this study, the model structural properties of the yolo model and the yolov1-v7 models are primarily analyzed and contrasted. According to the model design perspective, it compares and summarizes the structural enhancement and corresponding performance optimization of each model in the model structure, assesses the benefits and drawbacks of each model, and assesses their performance in light of the actual application impact of each model and the primary application fields, serving as a reference for the study of related topics. The article's summary and future direction are provided at the end.
Keywords
Artificial Intelligence, Target Detection, Image Processing, YOLO.
[1]. Redmon J, Divvala S, Girshick R, et al. You Only Look Once: Unified, Real-Time Object Detection. IEEE Conf. Com. Vis. Pat. Rec. 2016: 779-788.
[2]. Liu W, Anguelov D, Erhan D, et al. Ssd: Single shot multibox detector. Euro. Conf. Com. Vis., 2016: 21-37.
[3]. Redmon J, Farhadi A. Yolo 9000: Better, faster, stronger. IEEE Conf. Com. Vis. Pat. Rec. 2017. 6517-6525.
[4]. Girshick R, Donahue J, Darrell T, et al. Region-based convolutional networks for accurate object detection and segmentation. IEEE Trans. Pat. Anal. Mac. Intel., 2016, 38(1): 142-158.
[5]. Redmon J, Farhadi A. YOLOv3: An incremental improvement. arXiv preprint arXiv: 1804.02767, 2018.
[6]. Chen XL, Fang H, Lin TY, et al. Microsoft COCO captions: Data collection and evaluation server. arXiv preprint arXiv: 1504.00325, 2015.
[7]. Ren SQ, He KM, Girshick R, et al. Faster R-CNN: Towards real-time object detection with region proposal networks. Inter. Conf. Neu. Inf. Proc. Sys. Montréal, Canada. 2015. 91-99.
[8]. Girshick R. Fast R-CNN. IEEE Inter. Conf. Com. Vis. Santiago, Chile. 2015. 1440-1448
[9]. Bochkovskiy A, Wang C-Y, Mark-Liao H-Y. YOLOv4: Optimal Speed and Accuracy of Object Detection. arXiv preprint arXiv:2004.10934, 2020.
[10]. Liu S, Qi L, Qin H, et al. Path Aggregation Network for Instance Segmentation IEEE Conf. Com. Vis. Pat. Rec., 2018: 8759-8768.
[11]. Arthur D,Vassilvitskii S. k-means 1+:the advantages of careful seeding. Symp. Disc. Algo., 2007, 1027-1035.
[12]. Everingham M, Gool LV, Williams C K I, et al. The Pascal Visual Object Classes (VOC) Challenge. Inter. J. Com. Vis., 2010, 88(2):303-338.
[13]. Jocher Glenn. YOLOv5 release v6.1. https://github.com/ultralytics/yolov5 /releases/tag/v6.1, 2022. 2,7,10.
[14]. Wang C Y, Bochkovskiy A, Liao H Y M. YOLOv7: Trainable bag-of-freebies sets new state-of-the-art for real-time object detectors. arXiv preprint arXiv: 2207.02696, 2022.
[15]. Ge Z, Liu S, Wang F, et al. Yolox: Exceeding yolo series in 2021. arXiv preprint arXiv:2107.08430.
Cite this article
Nie,R. (2023). Research of target detection method YOLO. Applied and Computational Engineering,6,620-632.
Data availability
The datasets used and/or analyzed during the current study will be available from the authors upon reasonable request.
Disclaimer/Publisher's Note
The statements, opinions and data contained in all publications are solely those of the individual author(s) and contributor(s) and not of EWA Publishing and/or the editor(s). EWA Publishing and/or the editor(s) disclaim responsibility for any injury to people or property resulting from any ideas, methods, instructions or products referred to in the content.
About volume
Volume title: Proceedings of the 3rd International Conference on Signal Processing and Machine Learning
© 2024 by the author(s). Licensee EWA Publishing, Oxford, UK. This article is an open access article distributed under the terms and
conditions of the Creative Commons Attribution (CC BY) license. Authors who
publish this series agree to the following terms:
1. Authors retain copyright and grant the series right of first publication with the work simultaneously licensed under a Creative Commons
Attribution License that allows others to share the work with an acknowledgment of the work's authorship and initial publication in this
series.
2. Authors are able to enter into separate, additional contractual arrangements for the non-exclusive distribution of the series's published
version of the work (e.g., post it to an institutional repository or publish it in a book), with an acknowledgment of its initial
publication in this series.
3. Authors are permitted and encouraged to post their work online (e.g., in institutional repositories or on their website) prior to and
during the submission process, as it can lead to productive exchanges, as well as earlier and greater citation of published work (See
Open access policy for details).