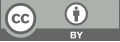
Research on the evolution of neural networks models in NLP
- 1 College of Engineering, University of Wisconsin – Madison, 1415 Engineering Drive, Madison, WI, United States of America, 53706
* Author to whom correspondence should be addressed.
Abstract
Since their first emergence, neural networks have produced significant results and have been an essential tool for solving natural language processing (NLP) problems. This paper aims to provide insight into the development of neural network models and their application in various NLP problems. The efficiency of the different approaches would be analyzed and insight into the different models would be provided. Overall, neural networks have been proven to be essential for solving problems related to NLP, and it is believed that further advancements in newer models would see a surge in performances of questions that were currently considered hard.
Keywords
Neural Network, Natural Language Processing, Algorithms, Machine Learning.
[1]. Y. Goldberg, ‘Neural network methods for natural language processing’, Synthesis lectures on human language technologies, vol. 10, Issue. 1, pp. 1–309, 2017.
[2]. D. Chen and C. D. Manning, “A fast and accurate dependency parser using neural networks,” in Proceedings of the 2014 conference on empirical methods in natural language processing (EMNLP), 2014, pp. 740–750.
[3]. M. Lewis and M. Steedman, “Improved CCG parsing with semi-supervised supertagging,” Transactions of the Association for Computational Linguistics, vol. 2, pp. 327–338, 2014.
[4]. M. M. Lopez and J. Kalita, “Deep Learning applied to NLP,” arXiv preprint arXiv:1703.03091, 2017.
[5]. N. Kalchbrenner, E. Grefenstette, and P. Blunsom, “A convolutional neural network for modelling sentences,” arXiv preprint arXiv:1404.2188, 2014.
[6]. S. Ruder, P. Ghaffari, and J. G. Breslin, “Insight-1 at semeval-2016 task 5: Deep learning for multilingual aspect-based sentiment analysis,” arXiv preprint arXiv:1609.02748, 2016.
[7]. X. Peng, "A Comparative Study of Neural Network for Text Classification," 2020 IEEE Conference on Telecommunications, Optics and Computer Science (TOCS), 2020, pp. 214-218, doi: 10.1109/TOCS50858.2020.9339702.
[8]. J. Xiao and Z. Zhou, "Research Progress of RNN Language Model," 2020 IEEE International Conference on Artificial Intelligence and Computer Applications (ICAICA), 2020, pp. 1285-1288, doi: 10.1109/ICAICA50127.2020.9182390.
[9]. S. S. Dodal and P. V. Kulkarni, "Multi-Lingual Information Retrieval Using Deep Learning," 2018 9th International Conference on Computing, Communication and Networking Technologies (ICCCNT), 2018, pp. 1-6, doi: 10.1109/ICCCNT.2018.8493789.
[10]. Staudemeyer, R.C. and E.R. Morris, Understanding LSTM--a tutorial into Long Short-Term Memory Recurrent Neural Networks. arXiv preprint arXiv:1909.09586, 2019.
[11]. Sak, H., A.W. Senior, and F. Beaufays, Long short-term memory recurrent neural network architectures for large scale acoustic modeling. 2014.
[12]. Hochreiter, S. and J. Schmidhuber, Long short-term memory. Neural computation, 1997. 9(8): p. 1735-1780.
[13]. C. A. Bahcevan, E. Kutlu and T. Yildiz, "Deep Neural Network Architecture for Part-of-Speech Tagging for Turkish Language," 2018 3rd International Conference on Computer Science and Engineering (UBMK), 2018, pp. 235-238, doi: 10.1109/UBMK.2018.8566272.
Cite this article
Zhao,Z. (2023). Research on the evolution of neural networks models in NLP. Applied and Computational Engineering,4,690-695.
Data availability
The datasets used and/or analyzed during the current study will be available from the authors upon reasonable request.
Disclaimer/Publisher's Note
The statements, opinions and data contained in all publications are solely those of the individual author(s) and contributor(s) and not of EWA Publishing and/or the editor(s). EWA Publishing and/or the editor(s) disclaim responsibility for any injury to people or property resulting from any ideas, methods, instructions or products referred to in the content.
About volume
Volume title: Proceedings of the 3rd International Conference on Signal Processing and Machine Learning
© 2024 by the author(s). Licensee EWA Publishing, Oxford, UK. This article is an open access article distributed under the terms and
conditions of the Creative Commons Attribution (CC BY) license. Authors who
publish this series agree to the following terms:
1. Authors retain copyright and grant the series right of first publication with the work simultaneously licensed under a Creative Commons
Attribution License that allows others to share the work with an acknowledgment of the work's authorship and initial publication in this
series.
2. Authors are able to enter into separate, additional contractual arrangements for the non-exclusive distribution of the series's published
version of the work (e.g., post it to an institutional repository or publish it in a book), with an acknowledgment of its initial
publication in this series.
3. Authors are permitted and encouraged to post their work online (e.g., in institutional repositories or on their website) prior to and
during the submission process, as it can lead to productive exchanges, as well as earlier and greater citation of published work (See
Open access policy for details).