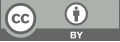
Application of Machine Learning and Deep Learning in Weather-related Forecasting
- 1 Computer Science, Hainan Bielefeld University of Applied Sciences, Danzhou, Hainan, China
* Author to whom correspondence should be addressed.
Abstract
Weather plays a broad and decisive role in many areas. Its volatility can disrupt traffic and endanger lives. It is therefore imperative to accurately predict its impact. Improved forecasting accuracy can aid multi-industry decision making. Traffic authorities can control traffic in advance; Agriculture can adjust its strategy in time; Resource allocation can be optimized in the energy sector. The rise of machine learning and deep learning technologies has opened up new prospects for weather-related forecasting. This article takes a methodical look at the application of machine learning and deep learning to traffic and weather forecasting, dissecting the details of model construction, data processing processes, and performance evaluation metrics. By comparing the advantages and disadvantages of each model, it provides ideas for model improvement, powerfully guides future research direction, and lays the foundation for building a more accurate prediction framework. The research direction of this thesis has far-reaching theoretical and practical value.
Keywords
Machine learning, deep learning, weather prediction, model application
[1]. Li, H. (2022). Machine learning methods. Tsinghua University Press.
[2]. Lee, J., Hong, B., Lee, K., & Jang, Y. J. (2015, December). A prediction model of traffic congestion using weather data. In 2015 IEEE International Conference on Data Science and Data Intensive Systems (pp. 81-88). IEEE.
[3]. Liu, Y., Fang, R., Su, Y., & Xiao, R. (2021). Research on regional landslide disaster warning model based on machine learning. Journal of Engineering Geology, 29(1), 116-124. https://doi.org/10.13544/j.cnki.jeg.2020-533
[4]. nardi, S., Sinambela, M., Humaidi, S., & Situmorang, M. (2024, November). Application of convolutional neural networks (CNN) for optimizing route changes based on dynamic weather conditions and travel time. In Proceedings of the National and International Seminar of the Faculty of Engineering and Computer Science, Dharmawangsa University (Vol. 1, No. 1, pp. 183-197).
[5]. Betkier, I., & Oszczypała, M. (2024). A novel approach to traffic modelling based on road parameters, weather conditions and GPS data using feedforward neural networks. Expert Systems with Applications, 245, 123067.
[6]. herstinsky, A. (2020). Fundamentals of recurrent neural network (RNN) and long short-term memory (LSTM) network. Physica D: Nonlinear Phenomena, 404, 132306.
[7]. Jaderberg, M., Simonyan, K., & Zisserman, A. (2015). Spatial transformer networks. Advances in Neural Information Processing Systems, 28.
[8]. Han, K., Xiao, A., Wu, E., Guo, J., Xu, C., & Wang, Y. (2021). Transformer in transformer. Advances in Neural Information Processing Systems, 34, 15908-15919.
[9]. Zhao, H., Jiang, L., Jia, J., Torr, P. H., & Koltun, V. (2021). Point transformer. In Proceedings of the IEEE/CVF International Conference on Computer Vision (pp. 16259-16268).
[10]. Zhang, Y. (2023). Interactive spatial co-location pattern mining based on machine learning (Doctoral dissertation, Guilin University of Electronic Technology).
Cite this article
Ye,Z. (2025). Application of Machine Learning and Deep Learning in Weather-related Forecasting. Applied and Computational Engineering,140,38-42.
Data availability
The datasets used and/or analyzed during the current study will be available from the authors upon reasonable request.
Disclaimer/Publisher's Note
The statements, opinions and data contained in all publications are solely those of the individual author(s) and contributor(s) and not of EWA Publishing and/or the editor(s). EWA Publishing and/or the editor(s) disclaim responsibility for any injury to people or property resulting from any ideas, methods, instructions or products referred to in the content.
About volume
Volume title: Proceedings of the 3rd International Conference on Mechatronics and Smart Systems
© 2024 by the author(s). Licensee EWA Publishing, Oxford, UK. This article is an open access article distributed under the terms and
conditions of the Creative Commons Attribution (CC BY) license. Authors who
publish this series agree to the following terms:
1. Authors retain copyright and grant the series right of first publication with the work simultaneously licensed under a Creative Commons
Attribution License that allows others to share the work with an acknowledgment of the work's authorship and initial publication in this
series.
2. Authors are able to enter into separate, additional contractual arrangements for the non-exclusive distribution of the series's published
version of the work (e.g., post it to an institutional repository or publish it in a book), with an acknowledgment of its initial
publication in this series.
3. Authors are permitted and encouraged to post their work online (e.g., in institutional repositories or on their website) prior to and
during the submission process, as it can lead to productive exchanges, as well as earlier and greater citation of published work (See
Open access policy for details).