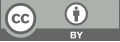
Identifying and updating local optimization methods in extended Kalman filter SLAM
- 1 Hong Kong University of Science and Technology Computer Engineering, HongKong, China, 999077
* Author to whom correspondence should be addressed.
Abstract
he essence of simultaneous location and mapping (SLAM) is an estimation problem. It requires mobile robots to use the sensor information to estimate the structure of the external environment in real-time and their position in the background. Smith and Cheeseman first applied the EKF estimation method to slam in the last century. The SLAM algorithm based on an extended Kalman filter (EKF-SLAM for short) is one of the research hotspots of slam technology. The EKF is improved based on the Kalman filter to make the Kalman filter suitable for nonlinear dynamic systems to solve the estimation problem of the nonlinear system model. Therefore, the Kalman filter can be applied to slam technology. The slam model based on filtering mainly includes three equations: Firstly, is to use the equation of motion, which will increase the uncertainty of robot positioning. Then the equation for initializing the guidepost according to the observation initializes the new state quantity according to the observation information. Lastly is the projection equation of the landmark state to comment, update and correct the condition according to the observation information to reduce the uncertainty. In the implementation, there exists a problem regarding storing the obstacle location. The current standard practice is to abandon all the existing data when the location number reaches the threshold. This will cause calculation time waste and instant high error [1]. In this paper, several improvement methods try to fully utilize the received message by different algorithms built on the ROS system. These include abandoning local data logic, updating data instead of deleting data, and retaining user data when updating new data. These advanced methods designed for EKF-SLAM will provide a more precise outcome with a low error range.
Keywords
extended Kalman Filter, SLAM, SLAM improvement algorithm
[1]. F. Qiang, "Analysis and Implementation of Improved SLAM Algorithm for Mobile Robot," Ordnance Industry Automation, pp. 86-89, 8 2018.
[2]. F. Zhou, "Research on navigation method of Lunar Rover," Proceedings of the 37th Chinese Control Conference, pp. 4803-4808, 25-27 6 2018.
[3]. L. M. Paz, "Divide and Conquer: EKF SLAM in O(n)," IEEE TRANSACTIONS ON ROBOTICS, VOL. 24, pp. 1107-1120, 10 2008.
[4]. C. Yanghua, "Car Positioning Based on Lidar of Extended Kalman Filter(EKF) Algorithm," Industrial Control Computer, pp. 12-17, 2019.
[5]. C. Jun and Z. Bi, "Improved EKF-SLAM algorithm based on landmark observation," Automation & Information Engineering, pp. 21-26, 2014.
[6]. Z. Wandong, "SLAM algorithm research in a complicated environment," Harbin Engineering University, 2019.
Cite this article
Liu,H. (2023). Identifying and updating local optimization methods in extended Kalman filter SLAM. Applied and Computational Engineering,4,569-573.
Data availability
The datasets used and/or analyzed during the current study will be available from the authors upon reasonable request.
Disclaimer/Publisher's Note
The statements, opinions and data contained in all publications are solely those of the individual author(s) and contributor(s) and not of EWA Publishing and/or the editor(s). EWA Publishing and/or the editor(s) disclaim responsibility for any injury to people or property resulting from any ideas, methods, instructions or products referred to in the content.
About volume
Volume title: Proceedings of the 3rd International Conference on Signal Processing and Machine Learning
© 2024 by the author(s). Licensee EWA Publishing, Oxford, UK. This article is an open access article distributed under the terms and
conditions of the Creative Commons Attribution (CC BY) license. Authors who
publish this series agree to the following terms:
1. Authors retain copyright and grant the series right of first publication with the work simultaneously licensed under a Creative Commons
Attribution License that allows others to share the work with an acknowledgment of the work's authorship and initial publication in this
series.
2. Authors are able to enter into separate, additional contractual arrangements for the non-exclusive distribution of the series's published
version of the work (e.g., post it to an institutional repository or publish it in a book), with an acknowledgment of its initial
publication in this series.
3. Authors are permitted and encouraged to post their work online (e.g., in institutional repositories or on their website) prior to and
during the submission process, as it can lead to productive exchanges, as well as earlier and greater citation of published work (See
Open access policy for details).