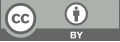
Research on the Application of Machine Learning in VaR/CVaR Measurement of Market, Credit, and Operational Risks
- 1 University of Melbourne, Melbourne, Australia
* Author to whom correspondence should be addressed.
Abstract
This study explores the integration of machine learning techniques in measuring market, credit, and operational value-at-risk (VaR) and conditional value-at-risk (VaR). By integrating traditional tools such as historical simulation method and Monte Carlo simulation with intelligent algorithms such as support vector machine and random survival forest, it breaks the limitations of traditional methods in dealing with high-dimensional heterogeneous data. The entire experimental system, including data cleaning, algorithm adaptation, and parameter optimization, was constructed. Empirical results show that the intelligent model has outstanding performance in extreme market volatility warning and credit default prediction scenarios. Especially in market stress tests, the gap risk capture accuracy of the model is increased by 40% compared with the traditional method, the default identification accuracy of the credit rating model is 89%, and the operational risk warning time is reduced by 60%. The results provide technical support to financial institutions in implementing dynamic risk control systems and encourage the transformation of the risk management paradigm from static assessment to intelligent early warning.
Keywords
Machine Learning, VaR, CVaR, Market Risk, Credit Risk, Operational Risk
[1]. Bollt, E. (2021). On explaining the surprising success of reservoir computing forecaster of chaos? The universal machine learning dynamical system with contrast to VAR and DMD. Chaos: An Interdisciplinary Journal of Nonlinear Science, 31(1).
[2]. Kaveh, A., Dadras Eslamlou, A., Javadi, S. M., & Geran Malek, N. (2021). Machine learning regression approaches for predicting the ultimate buckling load of variable-stiffness composite cylinders. Acta Mechanica, 232, 921-931..
[3]. Zhang, J., Cheng, T., Guo, W., Xu, X., Qiao, H., Xie, Y., & Ma, X. (2021). Leaf area index estimation model for UAV image hyperspectral data based on wavelength variable selection and machine learning methods. Plant Methods, 17(1), 49.
[4]. Christensen, K., Siggaard, M., & Veliyev, B. (2023). A machine learning approach to volatility forecasting. Journal of Financial Econometrics, 21(5), 1680-1727.
[5]. Cui, P., & Athey, S. (2022). Stable learning establishes some common ground between causal inference and machine learning. Nature Machine Intelligence, 4(2), 110-115.
[6]. Ryo, M. (2022). Explainable artificial intelligence and interpretable machine learning for agricultural data analysis. Artificial Intelligence in Agriculture, 6, 257-265.
[7]. Akkaya, B., Sener, E., & Gursu, C. (2022, June). A comparative study of heart disease prediction using machine learning techniques. In 2022 International Congress on Human-Computer Interaction, Optimization and Robotic Applications (HORA) (pp. 1-8). IEEE.
[8]. Hardini, M., Riza Chakim, M. H., Magdalena, L., Kenta, H., Rafika, A. S., & Julianingsih, D. (2023). Image-based air quality prediction using convolutional neural networks and machine learning. Aptisi Transactions on Technopreneurship (ATT).
[9]. Setiadi, D. R. I. M., Islam, H. M. M., Trisnapradika, G. A., & Herowati, W. (2024). Analyzing Preprocessing Impact on Machine Learning Classifiers for Cryotherapy and Immunotherapy Dataset. Journal of Future Artificial Intelligence and Technologies, 1(1), 39-50.
[10]. Krzyziński, M., Spytek, M., Baniecki, H., & Biecek, P. (2023). SurvSHAP (t): time-dependent explanations of machine learning survival models. Knowledge-Based Systems, 262, 110234.
[11]. Terraza, V., Boru İpek, A., & Rounaghi, M. M. (2024). The nexus between the volatility of Bitcoin, gold, and American stock markets during the COVID-19 pandemic: evidence from VAR-DCC-EGARCH and ANN models. Financial Innovation, 10(1), 22.
[12]. Ali, T. H., Raza, M. S., & Abdulqader, Q. M. (2024). VAR TIME SERIES ANALYSIS USING WAVELET SHRINKAGE WITH APPLICATION. Science Journal of University of Zakho, 12(3), 345-355.
Cite this article
Song,Z. (2025). Research on the Application of Machine Learning in VaR/CVaR Measurement of Market, Credit, and Operational Risks. Applied and Computational Engineering,145,129-134.
Data availability
The datasets used and/or analyzed during the current study will be available from the authors upon reasonable request.
Disclaimer/Publisher's Note
The statements, opinions and data contained in all publications are solely those of the individual author(s) and contributor(s) and not of EWA Publishing and/or the editor(s). EWA Publishing and/or the editor(s) disclaim responsibility for any injury to people or property resulting from any ideas, methods, instructions or products referred to in the content.
About volume
Volume title: Proceedings of the 3rd International Conference on Software Engineering and Machine Learning
© 2024 by the author(s). Licensee EWA Publishing, Oxford, UK. This article is an open access article distributed under the terms and
conditions of the Creative Commons Attribution (CC BY) license. Authors who
publish this series agree to the following terms:
1. Authors retain copyright and grant the series right of first publication with the work simultaneously licensed under a Creative Commons
Attribution License that allows others to share the work with an acknowledgment of the work's authorship and initial publication in this
series.
2. Authors are able to enter into separate, additional contractual arrangements for the non-exclusive distribution of the series's published
version of the work (e.g., post it to an institutional repository or publish it in a book), with an acknowledgment of its initial
publication in this series.
3. Authors are permitted and encouraged to post their work online (e.g., in institutional repositories or on their website) prior to and
during the submission process, as it can lead to productive exchanges, as well as earlier and greater citation of published work (See
Open access policy for details).