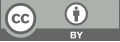
Automated Compliance Monitoring: A Machine Learning Approach for Digital Services Act Adherence in Multi-Product Platforms
- 1 Computer Science, University of Southern California, Los Angeles, CA
- 2 Computer Science, University of Southern California, Los Angeles, CA, USA
- 3 Computer Science, University of Texas at Dallas, Richardson, USA
- 4 Business Intelligence, Engineering School of Information and Digital Technologies, Villejuif, France
* Author to whom correspondence should be addressed.
Abstract
This paper presents an innovative machine learning approach for automated compliance monitoring of Digital Services Act (DSA) requirements across multi-product digital platforms. The proposed framework addresses the significant challenges of monitoring regulatory compliance in complex digital environments where manual verification processes prove insufficient and error-prone. The methodology introduces a formalized representation of DSA requirements through algorithmic processing and transforms these into machine-verifiable specifications using metamorphic testing principles and timed automata models. The core architecture implements a hybrid risk assessment model combining supervised and unsupervised learning techniques to evaluate compliance across heterogeneous platform environments. Comprehensive evaluation across multiple digital service categories demonstrates detection accuracy between 0.86-0.94 (F1-score) with processing efficiency ranging from 78% to 95% depending on platform characteristics. The multi-platform data integration pipeline achieves near real-time monitoring capabilities while respecting data protection constraints. The framework addresses key technical challenges including the complexity of requirement formalization, data access limitations, and adaptation to evolving regulatory interpretations. This research contributes significant advancements toward automated, scalable compliance verification solutions essential for effective implementation of the Digital Services Act across diverse digital service ecosystems.
Keywords
Digital Services Act, Compliance Monitoring, Machine Learning, Multi-platform Verification
[1]. Costa, M. (2020, October). Automated verification of compliance of non-functional requirements on mobile applications through metamorphic testing. In 2020 IEEE 13th International Conference on Software Testing, Validation and Verification (ICST) (pp. 421-423). IEEE.
[2]. Barati, M., Theodorakopoulos, G., & Rana, O. (2020, October). Automating GDPR compliance verification for cloud-hosted services. In 2020 International symposium on networks, computers and communications (ISNCC) (pp. 1-6). IEEE.
[3]. Gupta, A., Sharma, V., & Srivastava, R. (2021, December). BISRAC banking information security risk assessment and compliance model. In 2021 3rd International Conference on Advances in Computing, Communication Control and Networking (ICAC3N) (pp. 1447-1452). IEEE.
[4]. Botunac, I., Parlov, N., & Bosna, J. (2024, June). Opportunities of Gen AI in the Banking Industry with regards to the AI Act, GDPR, Data Act and DORA. In 2024 13th Mediterranean Conference on Embedded Computing (MECO) (pp. 1-6). IEEE.
[5]. Ceci, M., Sannier, N., Abualhaija, S., Shin, D., Bianculli, D., & Halling, M. (2024, April). Toward automated compliance checking of fund activities using runtime verification techniques. In Proceedings of the 1st IEEE/ACM Workshop on Software Engineering Challenges in Financial Firms (pp. 19-20).
[6]. Chen, J., Yan, L., Wang, S., & Zheng, W. (2024). Deep Reinforcement Learning-Based Automatic Test Case Generation for Hardware Verification. Journal of Artificial Intelligence General science (JAIGS) ISSN: 3006-4023, 6(1), 409-429.
[7]. Xu, Y., Liu, Y., Wu, J., & Zhan, X. (2024). Privacy by Design in Machine Learning Data Collection: An Experiment on Enhancing User Experience. Applied and Computational Engineering, 97, 64-68.
[8]. Xu, X., Xu, Z., Yu, P., & Wang, J. (2025). Enhancing User Intent for Recommendation Systems via Large Language Models. Preprints.
[9]. Yu, P., Xu, Z., Wang, J., & Xu, X. (2025). The Application of Large Language Models in Recommendation Systems. arXiv preprint arXiv:2501.02178.
[10]. Ma, D. (2024). AI-Driven Optimization of Intergenerational Community Services: An Empirical Analysis of Elderly Care Communities in Los Angeles. Artificial Intelligence and Machine Learning Review, 5(4), 10-25.
[11]. Ma, D., & Ling, Z. (2024). Optimization of Nursing Staff Allocation in Elderly Care Institutions: A Time Series Data Analysis Approach. Annals of Applied Sciences, 5(1).
[12]. Zheng, S., Zhang, Y., & Chen, Y. (2024). Leveraging Financial Sentiment Analysis for Detecting Abnormal Stock Market Volatility: An Evidence-Based Approach from Social Media Data. Academia Nexus Journal, 3(3).
[13]. Wang, P., Varvello, M., Ni, C., Yu, R., & Kuzmanovic, A. (2021, May). Web-lego: trading content strictness for faster webpages. In IEEE INFOCOM 2021-IEEE Conference on Computer Communications (pp. 1-10). IEEE.
[14]. Jiang, C., Jia, G., & Hu, C. (2024). AI-Driven Cultural Sensitivity Analysis for Game Localization: A Case Study of Player Feedback in East Asian Markets. Artificial Intelligence and Machine Learning Review, 5(4), 26-40.
[15]. Weng, J., & Jiang, X. (2024). Research on Movement Fluidity Assessment for Professional Dancers Based on Artificial Intelligence Technology. Artificial Intelligence and Machine Learning Review, 5(4), 41-54.
Cite this article
Wang,H.;Wu,J.;Ni,C.;Qian,K. (2025). Automated Compliance Monitoring: A Machine Learning Approach for Digital Services Act Adherence in Multi-Product Platforms. Applied and Computational Engineering,147,14-25.
Data availability
The datasets used and/or analyzed during the current study will be available from the authors upon reasonable request.
Disclaimer/Publisher's Note
The statements, opinions and data contained in all publications are solely those of the individual author(s) and contributor(s) and not of EWA Publishing and/or the editor(s). EWA Publishing and/or the editor(s) disclaim responsibility for any injury to people or property resulting from any ideas, methods, instructions or products referred to in the content.
About volume
Volume title: Proceedings of the 3rd International Conference on Mechatronics and Smart Systems
© 2024 by the author(s). Licensee EWA Publishing, Oxford, UK. This article is an open access article distributed under the terms and
conditions of the Creative Commons Attribution (CC BY) license. Authors who
publish this series agree to the following terms:
1. Authors retain copyright and grant the series right of first publication with the work simultaneously licensed under a Creative Commons
Attribution License that allows others to share the work with an acknowledgment of the work's authorship and initial publication in this
series.
2. Authors are able to enter into separate, additional contractual arrangements for the non-exclusive distribution of the series's published
version of the work (e.g., post it to an institutional repository or publish it in a book), with an acknowledgment of its initial
publication in this series.
3. Authors are permitted and encouraged to post their work online (e.g., in institutional repositories or on their website) prior to and
during the submission process, as it can lead to productive exchanges, as well as earlier and greater citation of published work (See
Open access policy for details).