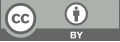
Financial Analysis: Intelligent Financial Data Analysis System Based on LLM-RAG
- 1 University of Pennsylvania, Pennsylvania, US
- 2 H. Milton Stewart School of Industrial and Systems Engineering, Georgia Institute of Technology, Atlanta, GA, USA
- 3 Tepper School of Business, Carnegie Mellon University, Pittsburgh, PA, USA
* Author to whom correspondence should be addressed.
Abstract
In the modern financial sector, the exponential growth of data has made efficient and accurate financial data analysis increasingly crucial. Traditional methods, such as statistical analysis and rule-based systems, often struggle to process and derive meaningful insights from complex financial information effectively. These conventional approaches face inherent limitations in handling unstructured data, capturing intricate market patterns, and adapting to rapidly evolving financial contexts, resulting in reduced accuracy and delayed decision-making processes. To address these challenges, this paper presents an intelligent financial data analysis system that integrates Large Language Models (LLMs) with Retrieval-Augmented Generation (RAG) technology. Our system incorporates three key components: a specialized preprocessing module for financial data standardization, an efficient vector-based storage and retrieval system, and a RAG-enhanced query processing module. Using the NASDAQ financial fundamentals dataset from 2010 to 2023, we conducted comprehensive experiments to evaluate system performance. Results demonstrate significant improvements across multiple metrics: the fully optimized configuration (gpt-3.5-turbo-1106+RAG) achieved 78.6% accuracy and 89.2% recall, surpassing the baseline model by 23 percentage points in accuracy while reducing response time by 34.8%. The system also showed enhanced efficiency in handling complex financial queries, though with a moderate increase in memory utilization. Our findings validate the effectiveness of integrating RAG technology with LLMs for financial analysis tasks and provide valuable insights for future developments in intelligent financial data processing systems.
Keywords
Intelligent financial analysis, LLM-RAG, NASDAQ data, Model comparison, Technology optimization
[1]. Arslan M, Munawar S, Cruz C. Business insights using RAG–LLMs: a review and case study[J]. Journal of Decision Systems, 2024: 1-30.
[2]. Arslan M, Munawar S, Cruz C. Business insights using RAG–LLMs: a review and case study[J]. Journal of Decision Systems, 2024: 1-30.
[3]. Zhang B, Yang H, Zhou T, et al. Enhancing financial sentiment analysis via retrieval augmented large language models[C]//Proceedings of the fourth ACM international conference on AI in finance. 2023: 349-356.
[4]. Wang S, Tan J, Dou Z, et al. OmniEval: An Omnidirectional and Automatic RAG Evaluation Benchmark in Financial Domain[J]. arXiv preprint arXiv:2412.13018, 2024.
[5]. Lim J H, Suh J W. A Study on implementing the most optimized RAG system for financial document using AutoRAG[C]//Annual Conference of KIPS. Korea Information Processing Society, 2024: 521-522.
[6]. Mao K, Liu Z, Qian H, et al. RAG-Studio: Towards In-Domain Adaptation of Retrieval Augmented Generation Through Self-Alignment[C]//Findings of the Association for Computational Linguistics: EMNLP 2024. 2024: 725-735.
[7]. Yepes A J, You Y, Milczek J, et al. Financial report chunking for effective retrieval augmented generation[J]. arXiv preprint arXiv:2402.05131, 2024.
Cite this article
Wang,J.;Ding,W.;Zhu,X. (2025). Financial Analysis: Intelligent Financial Data Analysis System Based on LLM-RAG. Applied and Computational Engineering,145,182-189.
Data availability
The datasets used and/or analyzed during the current study will be available from the authors upon reasonable request.
Disclaimer/Publisher's Note
The statements, opinions and data contained in all publications are solely those of the individual author(s) and contributor(s) and not of EWA Publishing and/or the editor(s). EWA Publishing and/or the editor(s) disclaim responsibility for any injury to people or property resulting from any ideas, methods, instructions or products referred to in the content.
About volume
Volume title: Proceedings of the 3rd International Conference on Software Engineering and Machine Learning
© 2024 by the author(s). Licensee EWA Publishing, Oxford, UK. This article is an open access article distributed under the terms and
conditions of the Creative Commons Attribution (CC BY) license. Authors who
publish this series agree to the following terms:
1. Authors retain copyright and grant the series right of first publication with the work simultaneously licensed under a Creative Commons
Attribution License that allows others to share the work with an acknowledgment of the work's authorship and initial publication in this
series.
2. Authors are able to enter into separate, additional contractual arrangements for the non-exclusive distribution of the series's published
version of the work (e.g., post it to an institutional repository or publish it in a book), with an acknowledgment of its initial
publication in this series.
3. Authors are permitted and encouraged to post their work online (e.g., in institutional repositories or on their website) prior to and
during the submission process, as it can lead to productive exchanges, as well as earlier and greater citation of published work (See
Open access policy for details).