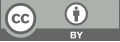
Performance analysis of using multimodal embedding and word embedding transferred to sentiment classification
- 1 Materials science and engineering, Hunan University of Technology, ZhuZhou, 412007, China
* Author to whom correspondence should be addressed.
Abstract
Multimodal machine learning is one of artificial intelligence's most important research topics. Contrastive Language-Image Pretraining (CLIP) is one of the applications of multimodal machine Learning and is well applied to computer vision. However, there is a research gap in applying CLIP in natural language processing. Therefore, based on IMDB, this paper applies the multimodal features of CLIP and three other pre-trained word vectors, Glove, Word2vec, and BERT, to compare their effects on sentiment classification of natural language processing, to test the performance of CLIP multimodal feature tuning in natural language processing. The results show that the multimodal feature of CLIP does not produce a significant effect on sentiment classification, and other multimodal features gain better effects. The highest accuracy is produced by BERT, and the Word embedding of CLIP is the lowest of the four accuracies of word embedding. At the same time, glove and word2vec are relatively close. The reason may be that the pre-trained CLIP model learns SOTA image representations from pictures and their descriptions, which is unsuitable for sentiment classification tasks. The specific reason remains untested.
Keywords
CLIP, Multimodal Machine Learning, Sentiment Classification, BERT.
[1]. Tomas Mikolov, Kai Chen, Greg Corrado, and Jeffrey Dean. Efficient estimation of word representations in vector space. arXiv preprint arXiv:1301.3781, 2013.
[2]. Jeffrey Pennington, Richard Socher, and Christopher Manning. 2014. GloVe: Global Vectors for Word Representation. In Proceedings of the 2014 Conference on Empirical Methods in Natural Language Processing (EMNLP), pages 1532–1543, Doha, Qatar.
[3]. Jacob Devlin, Ming-Wei Chang, Kenton Lee, and Kristina Toutanova. Bert: Pre-training of deep bidirectional transformers for language understanding. arXiv preprint arXiv:1810.04805, 2018.
[4]. Desai, K. and Johnson, J. Virtex: Learning visual rep-resentations from textual annotations. arXiv preprint arXiv:2006.06666, 2020.
[5]. Alec Radford, Jong Wook Kim et al. Learning Transferable Visual Models From Natural Language Supervision arXiv:2103.00020 ,2021.Association for Computational Linguistics.
[6]. Tenindra Abeywickrama, Muhammad Aamir Cheema, David Taniar: k-Nearest Neighbors on Road Networks: A Journey in Experimentation and In-Memory Implementation. CoRR abs/1601.01549 ,2016
[7]. Vaswani, Ashish, et al. Attention is all you need. Advances in neural information processing systems 30 (2017).
[8]. Elman, J. L. . Finding Structure in Time.Cognitive Science 14.2(1990):179-211.
[9]. Hochreiter, S. , and J. Schmidhuber . Long Short-Term Memory. Neural Computation 9.8(1997):1735-1780.
[10]. Matthew E. Peters, Waleed Ammar, Chandra Bhagavatula, Russell Power.Semi-supervised sequence tagging with bidirectional language models. arXiv:1705.00108,2017
Cite this article
Zou,Z. (2023). Performance analysis of using multimodal embedding and word embedding transferred to sentiment classification. Applied and Computational Engineering,5,417-422.
Data availability
The datasets used and/or analyzed during the current study will be available from the authors upon reasonable request.
Disclaimer/Publisher's Note
The statements, opinions and data contained in all publications are solely those of the individual author(s) and contributor(s) and not of EWA Publishing and/or the editor(s). EWA Publishing and/or the editor(s) disclaim responsibility for any injury to people or property resulting from any ideas, methods, instructions or products referred to in the content.
About volume
Volume title: Proceedings of the 3rd International Conference on Signal Processing and Machine Learning
© 2024 by the author(s). Licensee EWA Publishing, Oxford, UK. This article is an open access article distributed under the terms and
conditions of the Creative Commons Attribution (CC BY) license. Authors who
publish this series agree to the following terms:
1. Authors retain copyright and grant the series right of first publication with the work simultaneously licensed under a Creative Commons
Attribution License that allows others to share the work with an acknowledgment of the work's authorship and initial publication in this
series.
2. Authors are able to enter into separate, additional contractual arrangements for the non-exclusive distribution of the series's published
version of the work (e.g., post it to an institutional repository or publish it in a book), with an acknowledgment of its initial
publication in this series.
3. Authors are permitted and encouraged to post their work online (e.g., in institutional repositories or on their website) prior to and
during the submission process, as it can lead to productive exchanges, as well as earlier and greater citation of published work (See
Open access policy for details).