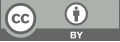
The Evolution and Convergence of Artificial Intelligence and Intelligent Robotics
- 1 Beijing University of Posts and Telecommunications, Beijing, China, 100080
* Author to whom correspondence should be addressed.
Abstract
Nowadays, AI's development, especially the emergence of large models, has given it a depth of thinking and generalization ability it never had before, and this ability can be applied across the board. As a field that has long intersected with AI technology, intelligent robots have shifted from past modes of thought and technical routes to today's large models. This paper comprehensively traces the technical evolution of intelligent robots from their inception to now, focusing on their path of integration with AI. Using literature research, comparative analysis, and trend analysis, combined with in - depth technical analysis, it identifies key moments in the evolution of intelligent robot technology and the internal logic of its integration with AI. The research indicates that the convergence of artificial intelligence and robotics has progressed from mere perception to cognitive capabilities, transitioning from specialized to general-purpose applications. Moving forward, this integration is expected to become increasingly prevalent as technological advancements steer towards broader applications.
Keywords
AI Robot, Multimodality, Artificial Intelligence, Reinforcement Learning, large language model
[1]. Lin, L. J. (1992). Reinforcement learning for robots using neural networks. Carnegie Mellon University.
[2]. Mnih, V., Badia, A. P., Mirza, M., Graves, A., Lillicrap, T., Harley, T., ... & Kavukcuoglu, K. (2016, June). Asynchronous methods for deep reinforcement learning. In International conference on machine learning (pp. 1928-1937). PmLR.
[3]. Gu, S., Holly, E., Lillicrap, T., & Levine, S. (2017, May). Deep reinforcement learning for robotic manipulation with asynchronous off-policy updates. In 2017 IEEE international conference on robotics and automation (ICRA) (pp. 3389-3396). IEEE.
[4]. Mirowski, P., Pascanu, R., Viola, F., Soyer, H., Ballard, A. J., Banino, A., ... & Hadsell, R. (2016). Learning to navigate in complex environments. arXiv preprint arXiv:1611.03673.
[5]. Agrawal, P., Carreira, J., & Malik, J. (2015). Learning to see by moving. In Proceedings of the IEEE international conference on computer vision (pp. 37-45).
[6]. Peng, X. B., Andrychowicz, M., Zaremba, W., & Abbeel, P. (2018, May). Sim-to-real transfer of robotic control with dynamics randomization. In 2018 IEEE international conference on robotics and automation (ICRA) (pp. 3803-3810). IEEE.
[7]. Florence, P. R., Manuelli, L., & Tedrake, R. (2018). Dense object nets: Learning dense visual object descriptors by and for robotic manipulation. arXiv preprint arXiv:1806.08756.
[8]. Calandra, R., Owens, A., Jayaraman, D., Lin, J., Yuan, W., Malik, J., ... & Levine, S. (2018). More than a feeling: Learning to grasp and regrasp using vision and touch. IEEE Robotics and Automation Letters, 3(4), 3300-3307.
[9]. Mirchandani, S., Xia, F., Florence, P., Ichter, B., Driess, D., Arenas, M. G., ... & Zeng, A. (2023). Large language models as general pattern machines. arXiv preprint arXiv:2307.04721.
[10]. Brohan, A., Brown, N., Carbajal, J., Chebotar, Y., Dabis, J., Finn, C., ... & Zitkovich, B. (2022). Rt-1: Robotics transformer for real-world control at scale. arXiv preprint arXiv:2212.06817.
[11]. Brohan, A., Brown, N., Carbajal, J., Chebotar, Y., Chen, X., Choromanski, K., ... & Zitkovich, B. (2023). Rt-2: Vision-language-action models transfer web knowledge to robotic control. arXiv preprint arXiv:2307.15818.
[12]. Liang, J., Huang, W., Xia, F., Xu, P., Hausman, K., Ichter, B., ... & Zeng, A. (2023, May). Code as policies: Language model programs for embodied control. In 2023 IEEE International Conference on Robotics and Automation (ICRA) (pp. 9493-9500). IEEE.
[13]. Ahn, M., Brohan, A., Brown, N., Chebotar, Y., Cortes, O., David, B., ... & Zeng, A. (2022). Do as i can, not as i say: Grounding language in robotic affordances. arXiv preprint arXiv:2204.01691.
[14]. Gupta, A., Savarese, S., Ganguli, S., & Fei-Fei, L. (2021). Embodied intelligence via learning and evolution. Nature communications, 12(1), 5721.
[15]. Driess, D., Xia, F., Sajjadi, M. S., Lynch, C., Chowdhery, A., Wahid, A., ... & Florence, P. (2023). Palm-e: An embodied multimodal language model.
Cite this article
Hong,M. (2025). The Evolution and Convergence of Artificial Intelligence and Intelligent Robotics. Applied and Computational Engineering,150,212-217.
Data availability
The datasets used and/or analyzed during the current study will be available from the authors upon reasonable request.
Disclaimer/Publisher's Note
The statements, opinions and data contained in all publications are solely those of the individual author(s) and contributor(s) and not of EWA Publishing and/or the editor(s). EWA Publishing and/or the editor(s) disclaim responsibility for any injury to people or property resulting from any ideas, methods, instructions or products referred to in the content.
About volume
Volume title: Proceedings of the 3rd International Conference on Software Engineering and Machine Learning
© 2024 by the author(s). Licensee EWA Publishing, Oxford, UK. This article is an open access article distributed under the terms and
conditions of the Creative Commons Attribution (CC BY) license. Authors who
publish this series agree to the following terms:
1. Authors retain copyright and grant the series right of first publication with the work simultaneously licensed under a Creative Commons
Attribution License that allows others to share the work with an acknowledgment of the work's authorship and initial publication in this
series.
2. Authors are able to enter into separate, additional contractual arrangements for the non-exclusive distribution of the series's published
version of the work (e.g., post it to an institutional repository or publish it in a book), with an acknowledgment of its initial
publication in this series.
3. Authors are permitted and encouraged to post their work online (e.g., in institutional repositories or on their website) prior to and
during the submission process, as it can lead to productive exchanges, as well as earlier and greater citation of published work (See
Open access policy for details).