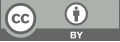
Comparison and analysis of the machine learning in the movie subtitle document classification
- 1 Faculty of Computer Intelligence, Peking University, Beijing, Beijing, 100091, China
- 2 Faculty of Science, University of Melbourne, Parkville, Melbourne, 3053, Australia
- 3 Faculty of Engineering, University of Sydney, Camperdown, Sydney, 2006, Australia
* Author to whom correspondence should be addressed.
Abstract
With the rapid iteration of film and technology, the quantity of movie related subtitle document has been rapidly expanded. However, the issue of classifying these documents is becoming non-negligible. This paper aims to compare different methods of classification of every machine learning algorithm on movie subtitle document and then analyse the result of each method. For the classification of movie subtitle document, this paper mostly does the following features of the work: firstly, retrieve of movie subtitle document and pre-processing step of text document. Secondly, deletion of the irrelevant text and extraction of the related texts, the methods are unsupervised machine learning approach and supervised machine learning approach respectively. Furthermore, analyse each classifier in various model which applied with different approach. Lastly, compare the result of different approach and each model then display the results.
Keywords
Movie subtitle classification, supervised learning, unsupervised learning.
[1]. Pimpalkar, A. P., and Raj, R. J. R 2020 Influence of pre-processing strategies on the performance of ML classifiers exploiting TF-IDF and BOW features. ADCAIJ: Advances in Distributed Computing and Artificial Intelligence Journal, 9, 49.
[2]. A, Geron, Hands-On Machine Learning with Scikit-Learn and TensorFlow, 7th Edition, 67-179.
[3]. YIFY Subtitles for English Movies, Available at: https://yts-subs.com/, Accessed: 10 December 2020.
[4]. Liu, L., Kang, J., Yu, J., and Wang, Z 2005 A comparative study on unsupervised feature selection methods for text clustering. In 2005 International Conference on Natural Language Processing and Knowledge Engineering, 597-601.
[5]. Hasan, M. M., Dip, S. T., Kamruzzaman, T. M., Akter, S., and Salehin, I 2021 December. Movie Subtitle Document Classification Using Unsupervised Machine Learning Approach. In 2021 IEEE 6th International Conference on Computing, Communication and Automation (ICCCA), 219-224.
[6]. Rajawat A.S., et.al 2022 Efficient Deep Learning for Reforming Authentic Content Searching on Big Data. In: Bianchini M., Piuri V., Das S., Shaw R.N. (eds) Advanced Computing and Intelligent Technologies. Lecture Notes in Networks and Systems, 218.
[7]. F. Liu, G. Zhang and J. Lu, 2020 Heterogeneous Domain Adaptation: An Unsupervised Approach, IEEE Transactions on Neural Networks and Learning Systems, 31, 5588-5602.
[8]. Hasan, M. M., Dip, S. T., Rahman, T., Akter, M. S., and Salehin, I 2021 Multilabel Movie Genre Classification from Movie Subtitle: Parameter Optimized Hybrid Classifier. In 2021 4th International Symposium on Advanced Electrical and Communication Technologies (ISAECT), 1-6.
[9]. S. C. Dharmadhikari, M. Ingle and P. Kulkarni 2011 Empirical Studies on Machine Learning Based Text Classification Algorithms, Advanced Computing: An International Journal (ACIJ), 2, No.6.
[10]. Y. F. Huang and S. H. Wang 2012 Movie Genre Classification Using SVM with Audio and Video Features, Lecture Notes in Computer Science, 1–10.
[11]. Sentiment Symposium Tutorial, Available: http://sentiment.christopherpotts.net/codedata/happyfuntokenizing.py, Accessed: 03 January 2020.
[12]. Bitbucket, Available at: https://bitbucket.org/jaganadhg/twittertokenize/src/, Accessed: 12 March 2020.
[13]. F. Sebastiani 2002 Machine learning in automated text categorization, ACM Computing Surveys, 34, 1–47.
[14]. C. Moral, A. D. Antonio, R. Imbert and J. Ramirez 2014 A survey of stemming algorithms in information retrieval, Politecnica de Madrid, 19.
Cite this article
Chu,B.;Xu,D.;Wu,D. (2023). Comparison and analysis of the machine learning in the movie subtitle document classification. Applied and Computational Engineering,5,642-648.
Data availability
The datasets used and/or analyzed during the current study will be available from the authors upon reasonable request.
Disclaimer/Publisher's Note
The statements, opinions and data contained in all publications are solely those of the individual author(s) and contributor(s) and not of EWA Publishing and/or the editor(s). EWA Publishing and/or the editor(s) disclaim responsibility for any injury to people or property resulting from any ideas, methods, instructions or products referred to in the content.
About volume
Volume title: Proceedings of the 3rd International Conference on Signal Processing and Machine Learning
© 2024 by the author(s). Licensee EWA Publishing, Oxford, UK. This article is an open access article distributed under the terms and
conditions of the Creative Commons Attribution (CC BY) license. Authors who
publish this series agree to the following terms:
1. Authors retain copyright and grant the series right of first publication with the work simultaneously licensed under a Creative Commons
Attribution License that allows others to share the work with an acknowledgment of the work's authorship and initial publication in this
series.
2. Authors are able to enter into separate, additional contractual arrangements for the non-exclusive distribution of the series's published
version of the work (e.g., post it to an institutional repository or publish it in a book), with an acknowledgment of its initial
publication in this series.
3. Authors are permitted and encouraged to post their work online (e.g., in institutional repositories or on their website) prior to and
during the submission process, as it can lead to productive exchanges, as well as earlier and greater citation of published work (See
Open access policy for details).