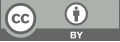
Machine learning models for house price prediction
- 1 Stuyvesant High School, 6617 Ovington Ct Brooklyn, NY 11204, United States
* Author to whom correspondence should be addressed.
Abstract
Housing prices have changed over the years, and there has been an increasing need to predict prices of future homes. This paper gives an overview of various machine learning models that can predict housing prices. There are numerous possible methods of pre-processing the data, so this paper explores ways to handle missing values and categorical data. In this study, the models of regression tree, random forest, XGBoost, gradient boosting, and LightGBM are described and used to predict housing prices. Machine learning models also have hyperparameters that can be adjusted, which can affect predictive accuracy. The methods are evaluated on several benchmark datasets. Based on the results, our approach is effective for the task of house price prediction.
Keywords
Machine Learning, House Price Prediction.
[1]. Cerda, P., Varoquaux, G., & Kégl, B. (2018). Similarity encoding for learning with dirty categorical variables. Machine Learning, 107(8), 1477–1494. doi:10.1007/s10994-018-5724-2.
[2]. Chen, T., & Guestrin, C. (2016). XGBoost: A Scalable Tree Boosting System. Proceedings of the 22nd ACM SIGKDD International Conference on Knowledge Discovery and Data Mining, 785–794. San Francisco, California, USA. doi:10.1145/2939672.2939785
[3]. Cock, D. D. (2011). Ames, Iowa: Alternative to the Boston Housing Data as an End of Semester Regression Project. Journal of Statistics Education, 19(3), null. doi:10.1080/10691898.2011.11889627
[4]. Cort J. Willmott, & Kenji Matsuura. (2005). Advantages of the mean absolute error (MAE) over the root mean square error (RMSE) in assessing average model performance. Climate Research, 30(1), 79–82. doi:10.3354/cr030079
[5]. Donders, A. R. T., van der Heijden, G. J. M. G., Stijnen, T., & Moons, K. G. M. (2006). Review: A gentle introduction to imputation of missing values. Journal of Clinical Epidemiology, 59(10), 1087–1091. doi:10.1016/j.jclinepi.2006.01.014
[6]. Friedman, J. H. (2001). Greedy function approximation: A gradient boosting machine. The Annals of Statistics, 29(5), 1189–1232. doi:10.1214/aos/1013203451
[7]. Hastie, T., Friedman, J., & Tisbshirani, R. (2017). The Elements of Statistical Learning: Data Mining, Inference, and Prediction. Springer.
[8]. James, G., Witten, D., Hastie, T., & Tibshirani, R. (2021). An Introduction to Statistical Learning: with Applications in R. Springer.
[9]. Ke, G., Meng, Q., Finley, T., Wang, T., Chen, W., Ma, W., … Liu, T.-Y. (2017). LightGBM: A Highly Efficient Gradient Boosting Decision Tree. Proceedings of the 31st International Conference on Neural Information Processing Systems, 3149–3157. Long Beach, California, USA. Red Hook, NY, USA: Curran Associates Inc.
[10]. Zulkifley, N., Rahman, S., Nor Hasbiah, U., & Ibrahim, I. (12 2020). House Price Prediction using a Machine Learning Model: A Survey of Literature. International Journal of Modern Education and Computer Science, 12, 46–54. doi:10.5815/ijmecs.2020.06.04.
Cite this article
Fang,L. (2023). Machine learning models for house price prediction. Applied and Computational Engineering,4,409-415.
Data availability
The datasets used and/or analyzed during the current study will be available from the authors upon reasonable request.
Disclaimer/Publisher's Note
The statements, opinions and data contained in all publications are solely those of the individual author(s) and contributor(s) and not of EWA Publishing and/or the editor(s). EWA Publishing and/or the editor(s) disclaim responsibility for any injury to people or property resulting from any ideas, methods, instructions or products referred to in the content.
About volume
Volume title: Proceedings of the 3rd International Conference on Signal Processing and Machine Learning
© 2024 by the author(s). Licensee EWA Publishing, Oxford, UK. This article is an open access article distributed under the terms and
conditions of the Creative Commons Attribution (CC BY) license. Authors who
publish this series agree to the following terms:
1. Authors retain copyright and grant the series right of first publication with the work simultaneously licensed under a Creative Commons
Attribution License that allows others to share the work with an acknowledgment of the work's authorship and initial publication in this
series.
2. Authors are able to enter into separate, additional contractual arrangements for the non-exclusive distribution of the series's published
version of the work (e.g., post it to an institutional repository or publish it in a book), with an acknowledgment of its initial
publication in this series.
3. Authors are permitted and encouraged to post their work online (e.g., in institutional repositories or on their website) prior to and
during the submission process, as it can lead to productive exchanges, as well as earlier and greater citation of published work (See
Open access policy for details).