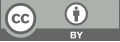
Improving U-net Model for Pulmonary Nodule Segmentation Through Attention Mechanism and Post-processing Module
- 1 Yunnan University, Kunming City, Yunnan Province, 650500, China
* Author to whom correspondence should be addressed.
Abstract
Pulmonary nodule segmentation plays a crucial role in the early detection and diagnosis of lung cancer, significantly impacting patient outcomes. The U-net model has emerged as a useful architecture in medical image processing like pulmonary nodule segmentation, gaining widespread popularity. However, U-net suffers from poor fine segmentation capabilities and the presence of noise in the segmentation results. In this study, we propose an Enhanced U-net model to improve segmentation results. The Enhanced U-net uses ECA and CRF modules. ECA module can make the model focus more on important features and improve the fine segmentation ability of the model. Meanwhile, CRF module allows the model to further refine the results, reduce the noise and boundary discontinuities. Utilizing the LIDC dataset, we evaluate the model’s performance through indicators such as recall, IoU, Dice score. Our findings show that Enhanced U-net can achieve the best performance among all U-net based models. And Enhanced U-net can significantly improve segmentation outcomes for small or blurred nodules.
Keywords
Pulmonary nodule, Semantic segmentation, U-net, Attention mechanism
[1]. Chon A, Balachandar N, Lu P. Deep convolutional neural networks for lung cancer detection[J]. Standford University, 2017: 1-9.
[2]. Armato III, Samuel G. et al., “Data From LIDC-IDRI.” The Cancer Imaging Archive, 2015, doi: 10.7937/K9/TCIA.2015.LO9QL9SX.
[3]. Kostis W J, Reeves A P, Yankelevitz D F, et al. Three-dimensional segmentation and growth-rate estimation of small pulmonary nodules in helical CT images[J]. IEEE transactions on medical imaging, 2003, 22(10): 1259-1274.
[4]. Dehmeshki J, Amin H, Valdivieso M, et al. Segmentation of pulmonary nodules in thoracic CT scans: a region growing approach[J]. IEEE transactions on medical imaging, 2008, 27(4): 467-480.
[5]. Kumar D, Wong A, Clausi D A. Lung nodule classification using deep features in CT images[C]//2015 12th conference on computer and robot vision. IEEE, 2015: 133-138.
[6]. Long J, Shelhamer E, Darrell T. Fully convolutional networks for semantic segmentation[C]//Proceedings of the IEEE conference on computer vision and pattern recognition. 2015: 3431-3440.
[7]. Ronneberger O, Fischer P, Brox T. U-net: Convolutional networks for biomedical image segmentation[C]//Medical image computing and computer-assisted intervention–MICCAI 2015: 18th international conference, Munich, Germany, October 5-9, 2015, proceedings, part III 18. Springer International Publishing, 2015: 234-241.
[8]. Çiçek Ö, Abdulkadir A, Lienkamp S S, et al. 3D U-Net: learning dense volumetric segmentation from sparse annotation[C]//Medical Image Computing and Computer-Assisted Intervention–MICCAI 2016: 19th International Conference, Athens, Greece, October 17-21, 2016, Proceedings, Part II 19. Springer International Publishing, 2016: 424-432.
[9]. Hu J, Shen L, Sun G. Squeeze-and-excitation networks[C]//Proceedings of the IEEE conference on computer vision and pattern recognition. 2018: 7132-7141.
[10]. Wang Q, Wu B, Zhu P, et al. ECA-Net: Efficient channel attention for deep convolutional neural networks[C]//Proceedings of the IEEE/CVF conference on computer vision and pattern recognition. 2020: 11534-11542.
[11]. Huang Z, Xu W, Yu K. Bidirectional LSTM-CRF models for sequence tagging[J]. arXiv preprint arXiv:1508.01991, 2015.
Cite this article
Cao,Y. (2025). Improving U-net Model for Pulmonary Nodule Segmentation Through Attention Mechanism and Post-processing Module. Applied and Computational Engineering,156,18-25.
Data availability
The datasets used and/or analyzed during the current study will be available from the authors upon reasonable request.
Disclaimer/Publisher's Note
The statements, opinions and data contained in all publications are solely those of the individual author(s) and contributor(s) and not of EWA Publishing and/or the editor(s). EWA Publishing and/or the editor(s) disclaim responsibility for any injury to people or property resulting from any ideas, methods, instructions or products referred to in the content.
About volume
Volume title: Proceedings of CONF-SEML 2025 Symposium: Intefrating AI into Software Engineering
© 2024 by the author(s). Licensee EWA Publishing, Oxford, UK. This article is an open access article distributed under the terms and
conditions of the Creative Commons Attribution (CC BY) license. Authors who
publish this series agree to the following terms:
1. Authors retain copyright and grant the series right of first publication with the work simultaneously licensed under a Creative Commons
Attribution License that allows others to share the work with an acknowledgment of the work's authorship and initial publication in this
series.
2. Authors are able to enter into separate, additional contractual arrangements for the non-exclusive distribution of the series's published
version of the work (e.g., post it to an institutional repository or publish it in a book), with an acknowledgment of its initial
publication in this series.
3. Authors are permitted and encouraged to post their work online (e.g., in institutional repositories or on their website) prior to and
during the submission process, as it can lead to productive exchanges, as well as earlier and greater citation of published work (See
Open access policy for details).