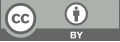
Reinforcement Learning in Practice: Muti-applications of Basic Theory
- 1 International School, Jinan University, Guangzhou, Guangdong, China
* Author to whom correspondence should be addressed.
Abstract
Reinforcement Learning (RL), as an essential branch of machine learning, has garnered significant attention due to its outstanding performance in complex decision-making tasks and dynamic environments, exemplified by notable achievements such as AlphaGo and DeepSeek in recent years. To provide researchers with a comprehensive perspective of current developments in this area, this paper systematically reviews RL from four distinct perspectives: fundamental theoretical frameworks, practical applications, existing limitations, and future outlook. Initially, core concepts and classical algorithms including Q-learning, SARSA, and Temporal Difference (TD) learning are clearly introduced, establishing a solid theoretical foundation. Then, practical implementations of RL are elaborated within three representative fields: path planning, voltage control and game theory, highlighting their significance and effectiveness through literature analysis. Moreover, this paper critically analyzes the limitations of current RL techniques, such as low sample efficiency, unstable training processes, and insufficient generalization. Finally, potential research directions, including hybrid learning paradigms and multi-agent collaborations, are proposed to inspire future advancements. The insights provided in this review aim to stimulate further theoretical innovation and practical breakthroughs in reinforcement learning.
Keywords
Reinforcement Learning, Path Planning, Voltage Control, Game Theory, Limitations
[1]. Ghallab, M., & Laruelle, H. (1994). Representation and control in IxTeT, a temporal planner. Journal of Artificial Intelligence Research, 1, 61–113. https://doi.org/10.1613/jair.9
[2]. Zhang, X., Wang, H., Zhang, J., & Zhao, Y. (2020). Hybrid path planning algorithm based on improved artificial potential field method and reinforcement learning. arXiv preprint. https://arxiv.org/abs/2006.15085v1
[3]. Zheng, G. Q., & Zhu, S. Q. (2004). An improved artificial potential field approach for path planning. Acta Automatica Sinica, 30(1), 89–93.
[4]. Sutton, R. S., & Barto, A. G. (2018). Reinforcement learning: An introduction (2nd ed.). MIT Press.
[5]. Mnih, V., Kavukcuoglu, K., Silver, D., Rusu, A. A., Veness, J., Bellemare, M. G., Graves, A., Riedmiller, M., Fidjeland, A. K., Ostrovski, G., Petersen, S., Beattie, C., Sadik, A., Antonoglou, I., King, H., Kumaran, D., Wierstra, D., Legg, S., & Hassabis, D. (2015). Human-level control through deep reinforcement learning. Nature, 518(7540), 529–533.
[6]. Ji, Y., Yun, K., Liu, Y., Xie, Z., & Liu, H. (2024). Neural-network-driven reward prediction as a heuristic: Advancing Q-learning for mobile robot path planning. arXiv preprint arXiv:2412.12650.
[7]. Pan, Q., Zhao, Y., & Gan, Y. (2025). Robot path planning based on improved Q-learning algorithm. Internet of Things Technologies, 2025(3), 23–30.
[8]. Tai, L., Paolo, G., & Liu, M. (2017). Virtual-to-real deep reinforcement learning: Continuous control of mobile robots for mapless navigation. Proceedings of the 2017 IEEE/RSJ International Conference on Intelligent Robots and Systems (IROS), 31–36.
[9]. Zhang, Y., & Gao, B. (2025). Research on AUV path planning based on deep reinforcement learning. Journal of Northeast Normal University (Natural Science Edition), 57(1), 53–62.
[10]. Kuznetsova, E., Li, Y. F., Ruiz, C., & Zio, E. (2013). Reinforcement learning for microgrid energy management.Energy, 59, 133–146.
[11]. Duan, J., Shi, D., Diao, R., Wang, H., Zhang, Z., & Bian, D. (2020). Deep-Reinforcement-Learning-Based Autonomous Voltage Control for Power Grid Operations.IEEE Transactions on Power Systems, 35(1), 814–817.
[12]. Zhang, Y., Xu, Y., Hu, W., & Wen, S. (2020). Distributed Voltage Regulation of Active DistributionNetworks Based on Enhanced Multi-Agent Deep Reinforcement Learning.IEEE Transactions on Power Systems, 35(6), 4964–4976.
[13]. OpenAI. (2019). OpenAI Five. https://openai.com/research/openai-five
[14]. Silver, D., Huang, A., Maddison, C. J., Guez, A., Sifre, L., Van Den Driessche, G., ... & Hassabis, D. (2016). Mastering the game of Go with deep neural networks and tree search. Nature, 529(7587), 484–489.
[15]. Silver, D., Schrittwieser, J., Simonyan, K., Antonoglou, I., Huang, A., Guez, A., ... & Hassabis, D. (2018). A general reinforcement learning algorithm that masters chess, shogi, and Go through self-play. Science, 362(6419), 1140–1144.
[16]. Vinyals, O., et al. (2019). Grandmaster level in StarCraft II using multi-agent reinforcement learning.Nature, 575, 350–354.
[17]. Dong, S., Li, C., Yang, G., Ge, Z., Cao, H., Chen, W., Yang, S., Chen, X., Li, W., & Gao, Y. (2025). A survey on solving and applications of hybrid game problems. Journal of Software, 36(1), 107–151.
[18]. Jin, X., Li, K., Jia, Q. S., Xia, H., Bai, Y., & Ren, D. (2020, November). A game-theoretic reinforcement learning approach for adaptive interaction at intersections. In 2020 Chinese Automation Congress (CAC) (pp. 4451-4456). IEEE.
[19]. Sun, S., & Wang, Q. (2025). Large-scale UAV swarm game confrontation based on improved multi-agent reinforcement learning. Journal of Southeast University (Natural Science Edition), 55(2), 123–134.
Cite this article
Su,H. (2025). Reinforcement Learning in Practice: Muti-applications of Basic Theory. Applied and Computational Engineering,158,65-73.
Data availability
The datasets used and/or analyzed during the current study will be available from the authors upon reasonable request.
Disclaimer/Publisher's Note
The statements, opinions and data contained in all publications are solely those of the individual author(s) and contributor(s) and not of EWA Publishing and/or the editor(s). EWA Publishing and/or the editor(s) disclaim responsibility for any injury to people or property resulting from any ideas, methods, instructions or products referred to in the content.
About volume
Volume title: Proceedings of CONF-SEML 2025 Symposium: Machine Learning Theory and Applications
© 2024 by the author(s). Licensee EWA Publishing, Oxford, UK. This article is an open access article distributed under the terms and
conditions of the Creative Commons Attribution (CC BY) license. Authors who
publish this series agree to the following terms:
1. Authors retain copyright and grant the series right of first publication with the work simultaneously licensed under a Creative Commons
Attribution License that allows others to share the work with an acknowledgment of the work's authorship and initial publication in this
series.
2. Authors are able to enter into separate, additional contractual arrangements for the non-exclusive distribution of the series's published
version of the work (e.g., post it to an institutional repository or publish it in a book), with an acknowledgment of its initial
publication in this series.
3. Authors are permitted and encouraged to post their work online (e.g., in institutional repositories or on their website) prior to and
during the submission process, as it can lead to productive exchanges, as well as earlier and greater citation of published work (See
Open access policy for details).