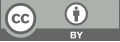
Serveless-based High-Dimensional Matrix Operations and Their Financial Applications
- 1 School of Telecommunications Engineering, Xidian University, Xi'an City, China
* Author to whom correspondence should be addressed.
Abstract
This paper systematically analyzes multivariate methods for high-dimensional matrix computation and their optimization strategies for applications in finance. At the level of high-dimensional computation, it focuses on the technical characteristics of direct methods, iterative methods , and randomized algorithms , which reveal their efficiency gains in financial derivatives pricing, risk matrix modeling, and other scenarios. For serverless architecture, the study focuses on its core advantages of elastic scaling and on-demand billing, through parallel task slicing and cost optimization, while analyzing the limitations of its stateless design on the adaptation of iterative algorithms and the constraints of cold-start latency on high-frequency trading. In addition, the article delves into the special challenges of financial modeling, including the cubic complexity pressure of high-dimensional operations, real-time conflicts of missing data interpolation, and privacy compliance requirements, and discusses hybrid architectures (serverless with local GPU synergy) and middleware (Redis, AWS Step Functions) as the current transitional solutions for balancing efficiency and state. The research also addresses the challenges of nonlinear dynamic modeling and interpretability requirements for machine learning-driven models, providing a multidimensional analytical framework for technology adaptability.
Keywords
Serveless, High-Dimensional Matrix, Financial Applications
[1]. Dean, J., Ghemawat, S.: 'MapReduce: Simplified Data Processing on Large Clusters’, Commun. ACM, 2008, 51, (1), pp. 107–113
[2]. Amazon Web Services (AWS): 'AWS Lambda: A Serverless Compute Service’, AWS Whitepaper, 2020
[3]. Strang, G.: 'Introduction to Linear Algebra’, 5th edn., Wellesley-Cambridge Press, 2016
[4]. Halko, N., Martinsson, P.G., Tropp, J.A.: 'Finding Structure with Randomness: Probabilistic Algorithms for Constructing Approximate Matrix Decompositions’, SIAM Rev., 2011, 53, (2), pp. 217–288
[5]. Duff, I.S., Erisman, A.M., Reid, J.K.: 'Direct Methods for Sparse Matrices’, Oxford Univ. Press, 2017
[6]. AWS Case Study: 'High-Performance Matrix Computation on AWS Lambda’, AWS Comput. Blog, 2022
[7]. J.P. Morgan Chase & Co.: 'Hybrid Serverless Architecture for Financial Risk Modeling’, Tech. Rep., 2023
[8]. AWS Documentation: 'Reducing Cold Starts with AWS Lambda SnapStart’, AWS Developer Guide, 2023
[9]. Smith, R.: 'Accelerating Financial Simulations with Serverless Computing’, J. Financ. Eng., 2021, 18, (3), pp. 45–60
[10]. Kairouz, P., et al.: 'Advances and Open Problems in Federated Learning’, arXiv preprint arXiv:1912.04977, 2019
Cite this article
Ying,S. (2025). Serveless-based High-Dimensional Matrix Operations and Their Financial Applications. Applied and Computational Engineering,158,11-16.
Data availability
The datasets used and/or analyzed during the current study will be available from the authors upon reasonable request.
Disclaimer/Publisher's Note
The statements, opinions and data contained in all publications are solely those of the individual author(s) and contributor(s) and not of EWA Publishing and/or the editor(s). EWA Publishing and/or the editor(s) disclaim responsibility for any injury to people or property resulting from any ideas, methods, instructions or products referred to in the content.
About volume
Volume title: Proceedings of CONF-SEML 2025 Symposium: Machine Learning Theory and Applications
© 2024 by the author(s). Licensee EWA Publishing, Oxford, UK. This article is an open access article distributed under the terms and
conditions of the Creative Commons Attribution (CC BY) license. Authors who
publish this series agree to the following terms:
1. Authors retain copyright and grant the series right of first publication with the work simultaneously licensed under a Creative Commons
Attribution License that allows others to share the work with an acknowledgment of the work's authorship and initial publication in this
series.
2. Authors are able to enter into separate, additional contractual arrangements for the non-exclusive distribution of the series's published
version of the work (e.g., post it to an institutional repository or publish it in a book), with an acknowledgment of its initial
publication in this series.
3. Authors are permitted and encouraged to post their work online (e.g., in institutional repositories or on their website) prior to and
during the submission process, as it can lead to productive exchanges, as well as earlier and greater citation of published work (See
Open access policy for details).