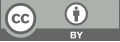
Review on short-term traffic flow prediction methods under big data
- 1 Beijing Jiatong University
* Author to whom correspondence should be addressed.
Abstract
The goal of traffic forecast is to predict the related traffic situation in the future according to the historical concept. The predicted angle can be divided into short-term prediction and long-term prediction. This method can be used to solve the increasingly serious urban traffic congestion problem, and researchers have proposed a deep learning model to help decision makers in the field of traffic control. It has made great contributions to improving future road capacity and optimizing intelligent transportation services. In this paper, short-term traffic forecast related documents under big data are helpful after sorting out, and the traffic flow data characteristics of intelligent transportation system and related correction methods are analysed. Secondly, it then classifies the applications involved by big data algorithm calculation according to various related principles, and summarizes the prediction accuracy, computational complexity, applicable interval and computation time of each type of algorithm in an overview. According to the relevant data, the combination forecasting model is effectively diversified, and combined with the related combination forecasting, I hope to improve the forecasting accuracy of the future development prospect.
Keywords
short-term traffic flow forecast, forecast models, intelligent transport system.
[1]. Alexopoulos C, Keramidis P, Pereira G V, Charalabidis Y 2022 Towards Smart Cities 4.0: Digital Participation in Smart Cities Solutions and the Use of Disruptive Technologies M. Themistocleous, M. Papadaki. Information Systems (emcis 2021). Cham: Springer International Publishing Ag, 437: 258–273.
[2]. Fu R, Zhang Z and Li L 2016 Using LSTM and GRU Neural Network Methods for Traffic Flow Prediction. 31st Youth Academic Annual Conference of Chinese Association of Automation New York: Ieee, 324–328.
[3]. Nagy A M, Simon V 2018 Survey on traffic prediction in smart cities. Pervasive and Mobile Computing, 50: 148–163.
[4]. Kong F, Li J, Jiang B, Tianyuan Z and Houbing S 2019 Big data-driven machine learning-enabled Traffic flow prediction. Transactions on Emerging Telecommunications Technologies, Hoboken: Wiley, 30(9): E3482.
[5]. Jing Y, Hu H, Siye G, Xuan W, and Fangqiu C 2021 Short-term Prediction of Urban Rail Transit Passenger Flow in External Passenger Transport Hub Based on LSTM-LGB-DRS. IEEE Transactions on Intelligent Transportation Systems, Piscataway: Ieee-Inst Electrical Electronics Engineers Inc, 22(7): 4611-4621.
[6]. Meng K, Shi C and Meng Y 2018 Vehicle Action Prediction Using Artificial Intelligence. M. A. Wani, M. Kantardzic, M. Sayedmouchaweh, wait. 17th IEEE International Conference on Machine Learning and Applications (ICMLA). New York: IEEE, 2018:1231-1236.
[7]. Jiahe Y, Honghui L, Yanhui B and Yingli L 2021 Spatial-temporal Traffic flow Restoration Data and Prediction Method Based on the Tensor Decomposition. Applied Sciences, Multidisciplinary Digital Publishing Institute, 2021,11(19): 9220.
[8]. Ma, C, Dai, G, and Zhou J 2021 Short-term Traffic Flow Prediction for Urban Road Sections Based on Time Series Analysis and LSTM_BILSTM Method. IEEE Transactions on Intelligent Transportation Systems, 23(6), 5615-5624.
[9]. El Faouzi N-E, Leung H, Kurian A. 2011 Data fusion in intelligent transportation systems: Progress and challenges - A survey. Information Fusion, Amsterdam: Elsevier, 12(1): 4-10.
[10]. Ding, F, Zhang, Z, Zhou, Y, Chen, X, and Ran B. 2019 Large-scale full-coverage traffic speed estimation under extreme traffic conditions using a big data and deep learning approach: Case study in China. Journal of Transportation Engineering, Part A: Systems, 145(5), 05019001.
[11]. Okutani I, Stephanedes Y. 1984 Dynamic Prediction of Traffic Volume Through Kalman Filtering Theory. Transportation Research Part B-Methodological, Oxford: Pergamon-Elsevier Science Ltd, 18(1): 1-11.
[12]. Guoguang H, Shoufeng M and Yu L 2002 Time Series Prediction method based on wavelet decomposition and reconstruction. Acta Automatica, 2002(6) : 1012-1014.
[13]. Zhai, H, Cui, L, Nie Y, Xu X, and Zhang W. 2018 A comprehensive comparative analysis of the basic theory of the short term bus passenger flow prediction. Symmetry, 10(9), 369.
[14]. Forbes G, Hall F. 1990 The Applicability of Catastrophe-Theory in Modeling Freeway Traffic Operations. Transportation Research Part a-Policy and Practice, Oxford: Pergamon-Elsevier Science Ltd, 24(5): 335-344.
[15]. Bengio Y, Simard P, Frasconi P. 1994 Learning Long-Term Dependencies with Gradient Descent Is Difficult. IEEE Transactions on Neural Networks, Piscataway: Ieee-Inst Electrical Electronics Engineers Inc, 5(2): 157-166.
[16]. Yao L, Mao C, Luo Y. 2019 Graph Convolutional Networks for Text Classification. Thirty-Third Aaai Conference on Artificial Intelligence / Thirty-First Innovative Applications of Artificial Intelligence Conference / Ninth Aaai Symposium on Educational Advances in Artificial Intelligence. Palo Alto: Assoc Advancement Artificial Intelligence, 2019: 7370-7377.
[17]. Zhu M, Yang X. 2019 Chinese Texts Classification System. IEEE 2nd International Conference on Information and Computer Technologies (icict). New York: IEEE, 2019: 149-152.
[18]. Yu F, Wei d, Zhang S, et. al. 2019 3D CNN-based Accurate Prediction for Large-scale Traffic flow. International Conference on Intelligent Transportation Engineering (icite 2019). New York: IEEE, 2019:99-103.
[19]. Huang y, Du j, Yang Z, et. al. 2022 A Survey on Trajectory-Prediction Methods for Autonomous Driving. IEEE Transactions on Intelligent Vehicles, Piscataway: Ieee-Inst Electrical Electronics Engineers Inc, 7(3): 652-674.
[20]. Djuric N, RADOSAVLJEVIC v, Cui H, et. al. 2020 Uncertainty-aware Short-term Motion Prediction of Traffic Actors for Autonomous Driving. IEEE Winter Conference on Applications of Computer Vision (WACV). Los Alamitos: IEEE Computer Soc, 2020:2084-2093.
[21]. Rumelhart D, Hinton G, Williams R. 1986 Learning Representations by Back-Propagating Errors. Nature, London: Macmillan Magazines Ltd, 323(6088): 533-536.
[22]. Zhang S, Yao y, Hu J, et. al. 2019 Deep Autoencoder Neural Networks for Short-Term Traffic Congestion Prediction of Transportation Networks. Sensors, Basel: MDPI, 19(10): 2229.
[23]. Xu D, Wang y, Jia l, et. al. 2017 Real-time road traffic state prediction based on Arima and Kalman filter. Frontiers of Information Technology and Electronic Engineering, Hangzhou: Zhejiang Univ, 18(2): 287-302.
[24]. Bates J, Granger C 1969 Combination of forecasts. Operational Research Quarterly, Oxford: Pergamon-Elsevier Science Ltd, 1969, 20(4): 451-455.
Cite this article
Tang,S. (2023). Review on short-term traffic flow prediction methods under big data. Applied and Computational Engineering,10,233-239.
Data availability
The datasets used and/or analyzed during the current study will be available from the authors upon reasonable request.
Disclaimer/Publisher's Note
The statements, opinions and data contained in all publications are solely those of the individual author(s) and contributor(s) and not of EWA Publishing and/or the editor(s). EWA Publishing and/or the editor(s) disclaim responsibility for any injury to people or property resulting from any ideas, methods, instructions or products referred to in the content.
About volume
Volume title: Proceedings of the 2023 International Conference on Mechatronics and Smart Systems
© 2024 by the author(s). Licensee EWA Publishing, Oxford, UK. This article is an open access article distributed under the terms and
conditions of the Creative Commons Attribution (CC BY) license. Authors who
publish this series agree to the following terms:
1. Authors retain copyright and grant the series right of first publication with the work simultaneously licensed under a Creative Commons
Attribution License that allows others to share the work with an acknowledgment of the work's authorship and initial publication in this
series.
2. Authors are able to enter into separate, additional contractual arrangements for the non-exclusive distribution of the series's published
version of the work (e.g., post it to an institutional repository or publish it in a book), with an acknowledgment of its initial
publication in this series.
3. Authors are permitted and encouraged to post their work online (e.g., in institutional repositories or on their website) prior to and
during the submission process, as it can lead to productive exchanges, as well as earlier and greater citation of published work (See
Open access policy for details).