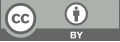
The application of extended kalman filtering based on SLAM
- 1 Hubei University of Automotive Technology
* Author to whom correspondence should be addressed.
Abstract
EKF (Extended Kalman Filter) is a non-linear state estimation algorithm based on the development of Kalman filtering technology. In SLAM (Simultaneous Localization and Mapping), EKF technology is widely used to realize robots' independent positioning and map construction. EKF technology mainly estimates the state of the robot by using the information provided by the sensor, and then realizes the robot's self-positioning and the environment's map. This paper uses EKF algorithm for testing, and analyses the simulation results, which compared with Kalman filtering technology, the main difference between the two is that EKF can handle non -linear dynamic systems. In SLAM, robots often detect environmental detection through sensors such as laser radar, camera, and then update the position and posture of the robot by the receiving sensor data. In this process, the EKF technology can perform non -linearity by sensor data by sensor data Transformation makes the state of the robot more accurately estimate. Specifically, Extended Kalman Filtering technology can be divided into two steps: prediction and update. In the predicted phase, EKF uses the current robotic status and motion equation to predict the robot status of the next moment; in the update stage, EKF uses sensors to measure data to update the current state of the robot. By repeating the prediction and updating two steps, you can get the trajectory of the robot movement and the map of the environment where the robot is located.
Keywords
extended kalman filter, SLAM, non-linear.
[1]. X. Liu, W.G Zhang, H. Zhong, X. X. Liu, “Study on SLAM -based rotor combination navigation algorithms,” Computer Measurement and Control, vol .2019,27(03),pp. 197-201.
[2]. T. Bailey and H. Durrant-Whyte, “Simultaneous localization and mapping (SLAM): part II,” IEEE Robot. Automat. Mag., vol. 13, no. 3, pp. 108–117, Sep. 2006.
[3]. B. Zheng and Z. Zhang, “An Improved EKF-SLAM for Mars Surface Exploration,” International Journal of Aerospace Engineering, vol. 2019, pp. 1–9, Feb. 2019.
[4]. L. Wei, Z. Z. Lei, M. Z. Qi, J. R. Yang, “Construction of three -dimensional maps of aircraft simulation cockpit based on visual SLAM,” Civil Aircraft Design and Research, vol. 2023, 148(01), pp.128-133.
[5]. Z. D. Lin, “Analysis of the SLAM problem based on the Extended Kalman Filter algorithm,” City Building, vol. 2020,17(11), pp.104-106.
[6]. T. H. Wu, “Non -linear navigation data processing theory and its application,” Shan Dong University, vol. 2018.
[7]. Y. Shi, H. Y. Wu, W. X. Wen, S. Y. Peng, “Study on SLAM research based on the extended Karman filter,” Electronic Design Engineering, vol. 2012,20(01), pp.104-106.
[8]. X. T. Wang, Y. L. Cai, H. N. Jiang, “SLAM algorithm based on adaptive and strong tracking EKF,” China Automation Society System Simulation Professional Committee, vol. 2022,05, pp.282-286.
[9]. D. F. Shen, G. F. Shang, G. Zhao, “Improve the EKF-SLAM automatic landing car positioning algorithm,” Journal of Xi'an University of Engineering, vol. 2022,36(06), pp.77-85.
[10]. P. C. Cao, J. Lu, Q. M. Gao, “SLAM technology research and analysis based on small plots based on EKF algorithm,” Southern Agricultural Machinery, vol. 2021,52(23), pp. 6+1-4.
[11]. T. Liu, “The preliminary application and simulation of the EKF-SLAM algorithm in the
[12]. asteroid speed measurement,” Scientific and Technological Information, vol. 2015,13(26), pp. 241-242.
[13]. Y. Li, “Map construction and path planning of underwater robots,” Nanjing Information Engineering University, vol. 2018.
[14]. Y. H. Cai, J. Tan, G. Wang, “Car Positioning Based on Lidar of Extended Kalman Filter Algorithm and Simultaneous Localization and Mapping,” Industrial Control Computer, vol. 2019,32(12), pp. 12-14.
Cite this article
Cai,X. (2023). The application of extended kalman filtering based on SLAM. Applied and Computational Engineering,12,46-51.
Data availability
The datasets used and/or analyzed during the current study will be available from the authors upon reasonable request.
Disclaimer/Publisher's Note
The statements, opinions and data contained in all publications are solely those of the individual author(s) and contributor(s) and not of EWA Publishing and/or the editor(s). EWA Publishing and/or the editor(s) disclaim responsibility for any injury to people or property resulting from any ideas, methods, instructions or products referred to in the content.
About volume
Volume title: Proceedings of the 2023 International Conference on Mechatronics and Smart Systems
© 2024 by the author(s). Licensee EWA Publishing, Oxford, UK. This article is an open access article distributed under the terms and
conditions of the Creative Commons Attribution (CC BY) license. Authors who
publish this series agree to the following terms:
1. Authors retain copyright and grant the series right of first publication with the work simultaneously licensed under a Creative Commons
Attribution License that allows others to share the work with an acknowledgment of the work's authorship and initial publication in this
series.
2. Authors are able to enter into separate, additional contractual arrangements for the non-exclusive distribution of the series's published
version of the work (e.g., post it to an institutional repository or publish it in a book), with an acknowledgment of its initial
publication in this series.
3. Authors are permitted and encouraged to post their work online (e.g., in institutional repositories or on their website) prior to and
during the submission process, as it can lead to productive exchanges, as well as earlier and greater citation of published work (See
Open access policy for details).