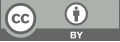
Improved time-aware debiasing models for recommender systems
- 1 Cranbrook Schools
- 2 Dalian University of Technology
- 3 The Affiliated High School of Peking University
- 4 The University of Sydney
- 5 University of California
- 6 University College Dublin
* Author to whom correspondence should be addressed.
Abstract
Recommender systems are affected by user selection bias during user interactions. Previously, Huang, Jin et al. proposed the TMF-DANCER model to deal with the fact that selection bias is dynamic, and the popularity of an item and user preferences may change drastically over time. However, the previous time-aware methods did not consider the continuity of time, and performing collaborative filtering with matrix factorization may not be able to capture the complex structure of user interaction data. Our methods enhance the effectiveness of time-aware debiasing by utilizing the time-varying sequential method to alleviate the discontinuity of time-based on the frequency-based method, Replacing the matrix factorization with neural collaborative filtering (NCF). We used movie lens 100k as the data for training. The experimental results indicate that methods incorporating the time-varying sequential method and NCF have better performance in rating prediction than debiasing methods without.
Keywords
Selection bias, Dynamic user preferences, Time-varying sequential method, Time-aware Neural Collaborative Filtering
[1]. Huang, Jin, Harrie Oosterhuis, and Maarten de Rijke. “It is different when items are older: Debiasing Recommendations when Selection Bias and User Preferences are dynamic.” Proceedings of the Fifteenth ACM International Conference on Web Search and Data Mining. 2022.
[2]. He, Xiangnan, et al. “Neural Collaborative Filtering.” Proceedings of the 26th international conference on world wide web. 2017.
[3]. Schnabel, Tobias, et al. “Recommendations as treatments: Debiasing learning and evaluation.” international conference on machine learning. PMLR, 2016.
[4]. Saito, Yuta, et al. “Unbiased recommender learning from missing-not-at-random implicit feedback.” Proceedings of the 13th International Conference on Web Search and Data Mining. 2020.
[5]. Wu, Xinwei, et al. “Unbiased learning to rank in feeds recommendation.” Proceedings of the 14th ACM International Conference on Web Search and Data Mining. 2021.
[6]. Islam, Rashidul, et al. “Debiasing career recommendations with neural fair collaborative filtering.” Proceedings of the Web Conference 2021. 2021.
[7]. Steck, H. Training and testing of recommender systems on data missing not at random. In KDD, pp. 713–722, 2010.
[8]. Wang, Xiaojie, et al. “Doubly robust joint learning for recommendation on data missing not at random.” International Conference on Machine Learning. PMLR, 2019.
[9]. Hanley JA, McNeil BJ (1982) The meaning and use of the area under a receiver operating characteristic (ROC) curve. Radiology 143:29–36.
Cite this article
Wang,S.;Wu,X.;Zhao,L.;Xue,Y.;Xia,R.;Sun,Z. (2023). Improved time-aware debiasing models for recommender systems. Applied and Computational Engineering,6,1560-1576.
Data availability
The datasets used and/or analyzed during the current study will be available from the authors upon reasonable request.
Disclaimer/Publisher's Note
The statements, opinions and data contained in all publications are solely those of the individual author(s) and contributor(s) and not of EWA Publishing and/or the editor(s). EWA Publishing and/or the editor(s) disclaim responsibility for any injury to people or property resulting from any ideas, methods, instructions or products referred to in the content.
About volume
Volume title: Proceedings of the 3rd International Conference on Signal Processing and Machine Learning
© 2024 by the author(s). Licensee EWA Publishing, Oxford, UK. This article is an open access article distributed under the terms and
conditions of the Creative Commons Attribution (CC BY) license. Authors who
publish this series agree to the following terms:
1. Authors retain copyright and grant the series right of first publication with the work simultaneously licensed under a Creative Commons
Attribution License that allows others to share the work with an acknowledgment of the work's authorship and initial publication in this
series.
2. Authors are able to enter into separate, additional contractual arrangements for the non-exclusive distribution of the series's published
version of the work (e.g., post it to an institutional repository or publish it in a book), with an acknowledgment of its initial
publication in this series.
3. Authors are permitted and encouraged to post their work online (e.g., in institutional repositories or on their website) prior to and
during the submission process, as it can lead to productive exchanges, as well as earlier and greater citation of published work (See
Open access policy for details).