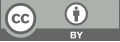
Crop disease recognition based on the YOLOv5 and transfer learning
- 1 University of Electronic Science and Technology
* Author to whom correspondence should be addressed.
Abstract
Crop disease detection is an important factor in agricultural production. Traditional object detection methods can't effectively screen key features, resulting in weak crop disease control in many countries. In recent years, several convolutional neural networks for object detection have been proposed, which makes it possible to apply computer vision to crop disease identification through deep learning. YOLOv5 is an advanced object detection network, which can extract key features and use human visual attention mechanism as a reference. This paper would evaluate the performance of four pre-trained models of YOLOv5 in object detection of crop diseases. And transfer learning was used to train the corresponding dataset. The experiment results have showed that the F1 values of the four models all reached above 0.93, and the Yolov5x got the best result, which achieved 0.963. Furthermore, the detection accuracy of the four models has reached more than 98%. This shows that the YOLOv5 series network models have great application prospects in the identification of crop diseases. In the near future, the object detection model can be applied to various mobile devices, even unmanned aerial vehicles, which would play a significant role in crop disease prevention.
Keywords
crop disease classification, YOLOv5, image processing, deep learning
[1]. Jagan K, Balasubramanian M, Palanivel S.: Detection and Recognition of Diseases from Paddy Plant Leaf Images[J]. International Journal of Computer Applications, 144(12):34-41(2016).
[2]. H. Waghmare, R. Kokare, Y. Dandawate, Detection and classification of diseases of Grape plant using opposite colour Local Binary Pattern feature and machine learning for automated Decision Support System, 2016 3rd International Conference on Signal Processing and Integrated Networks (SPIN), pp. 513-518(2016).
[3]. Mohammadpoor M, Nooghabi M G, Ahmedi Z. An Intelligent Technique for Grape Fanleaf Virus Detection[J]. International Journal of Interactive Multimedia and Artificial Intelligence, InPress(InPress):1(2020).
[4]. Atanassova S, Nikolov P, Valchev N, et al. Early detection of powdery mildew (Podosphaera xanthii) on cucumber leaves based on visible and near-infrared spectroscopy[C]// 10th Jubilee International Conference of the Balkan Physical Union (2019).
[5]. Zhu J, Wu A, Wang X, et al. Identification of grape diseases using image analysis and BP neural networks[J]. Multimedia Tools and Applications (2019).
[6]. K. P. Ferentinos, Deep learning models for plant disease detection and diagnosis, Computers and Electronics in Agriculture, vol. 145, pp. 311318 (2018).
[7]. H. A. Atabay, Deep residual learning for tomato plant leaf disease identification, Journal of Theoretical & Applied Information Technology, vol. 95(24) (2017).
[8]. Liu, W. Anguelov, D.; Erhan, D.; Szegedy, C.; Reed, S.; Cheng-Yang, F.; Berg, A.C. SSD: Single Shot MulfiBox Detector. In European Conference on Computer Vision; pp. 21–37. Springer: Cham, Switzerland (2016).
[9]. Wang, H.; Shang, S.; Wang, D.; He, X.; Feng, K.; Zhu, H. Plant Disease Detection and Classification Method Based on the Optimized Lightweight YOLOv5 Model. Agriculture 2022, 12, 931 (2022).
[10]. Kaggle web, https://www.kaggle.com/datasets/nurnob101/rice-disease,last accessed 2022/09/14
[11]. Kaggle web, https://www.kaggle.com/datasets/adefiqri12/riceleafsv3, last accessed 2022/09/14
[12]. labelImg web, https://github.com/heartexlabs/labelImg, last accessed 2022/08/14
[13]. Ren S, He K, Girshick R, et al. Faster r-cnn: Towards real-time object detection with region proposal networks[J]. Advances in neural information processing systems, 28 (2015).
[14]. Wei L, Dragomir A, Dumitru E, et al. SSD: Single Shot MultiBox Detector[J]. Springer, Cham (2016).
[15]. J. Redmon, S. Divvala, R. Girshick and A. Farhadi, You Only Look Once: Unified, Real-Time Object Detection, 2016 IEEE Conference on Computer Vision and Pattern Recognition (CVPR), pp. 779-788 (2016).
[16]. J. Redmon and A. Farhadi, "YOLO9000: Better, Faster, Stronger," 2017 IEEE Conference on Computer Vision and Pattern Recognition (CVPR), pp. 6517-6525 (2017).
[17]. Redmon J, Farhadi A. Yolov3: An incremental improvement. arXiv: 1804.02767 (2018).
[18]. Liu S, Qi L, Qin H, et al. Path Aggregation Network for Instance Segmentation[C]// 2018 IEEE/CVF Conference on Computer Vision and Pattern Recognition (CVPR). IEEE (2018).
[19]. Dong-Yan Zhang, Han-Sen Luo, Dao-Yong Wang, Xin-Gen Zhou, Wei-Feng Li, Chun-Yan Gu, Gan Zhang, Fang-Ming He, Assessment of the levels of damage caused by Fusarium head blight in wheat using an improved YoloV5 method, Computers and Electronics in Agriculture, Vol. 198 (2022).
[20]. Jiangtao Qi, Xiangnan Liu, Kai Liu, Farong Xu, Hui Guo, Xinliang Tian, Mao Li, Zhiyuan Bao, Yang Li, An improved YOLOv5 model based on visual attention mechanism: Application to recognition of tomato virus disease, Computers and Electronics in Agriculture, Vol. 194 (2022).
[21]. Bahdanau, D., Cho, K., & Bengio, Y. Neural machine translation by jointly learning to align and translate. Computer Science (2014).
Cite this article
Ding,Q. (2023). Crop disease recognition based on the YOLOv5 and transfer learning. Applied and Computational Engineering,13,46-57.
Data availability
The datasets used and/or analyzed during the current study will be available from the authors upon reasonable request.
Disclaimer/Publisher's Note
The statements, opinions and data contained in all publications are solely those of the individual author(s) and contributor(s) and not of EWA Publishing and/or the editor(s). EWA Publishing and/or the editor(s) disclaim responsibility for any injury to people or property resulting from any ideas, methods, instructions or products referred to in the content.
About volume
Volume title: Proceedings of the 5th International Conference on Computing and Data Science
© 2024 by the author(s). Licensee EWA Publishing, Oxford, UK. This article is an open access article distributed under the terms and
conditions of the Creative Commons Attribution (CC BY) license. Authors who
publish this series agree to the following terms:
1. Authors retain copyright and grant the series right of first publication with the work simultaneously licensed under a Creative Commons
Attribution License that allows others to share the work with an acknowledgment of the work's authorship and initial publication in this
series.
2. Authors are able to enter into separate, additional contractual arrangements for the non-exclusive distribution of the series's published
version of the work (e.g., post it to an institutional repository or publish it in a book), with an acknowledgment of its initial
publication in this series.
3. Authors are permitted and encouraged to post their work online (e.g., in institutional repositories or on their website) prior to and
during the submission process, as it can lead to productive exchanges, as well as earlier and greater citation of published work (See
Open access policy for details).