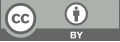
Control method and analysis of potential application based on memristor
- 1 Ulink college of Shanghai
* Author to whom correspondence should be addressed.
Abstract
Memristors have many advantages, such as small size, fast speed, and low power consumption. If the cross array is used as the memristor, a structure similar to the matrix can be obtained, which can store data and do calculation. Based on the characteristics of memristor, the realization of various control functions has a wide range of application prospects and advantages. The memristor matrix supply can be used to achieve relevant control, so that the control becomes simple and efficient. At the same time, memristor has outstanding advantages in arithmetic calculation, neural network, and artificial intelligence. This article introduces memristor and shows the theory needed to apply memristor to the control of robot arm, improve MAC calculation by memristor and the application in neural network. There is no doubt that the research in this paper will contribute to the further exploration of the control method of memristor and lay a solid foundation for its practical potential application theory in the future.
Keywords
memristor, matrix, cross array, neural network, MAC
[1]. L. Chua, “Memristor-The missing circuit element,” IEEE Transactions on Circuit Theory, vol. 18, no. 5, pp. 507–519, 1971.
[2]. D. B. Strukov, G. S. Snider, D. R. Stewart, and R. S. Williams, “The missing memristor found,” Nature, vol. 453, no. 7191, pp. 80–83, May 2008.
[3]. R. Lin, G. Shi, F. Qiao, C. Wang, and S. Wu, “Research progress and applications of memristor emulator circuits,” Microelectronics Journal, vol. 133, p. 105702, Mar. 2023.
[4]. A. A. Gismatulin, G. N. Kamaev, V. A. Volodin, and V. A. Gritsenko, “Charge Transport Mechanism in the Forming-Free Memristor Based on PECVD Silicon Oxynitride,” Electronics, vol. 12, no. 3, p. 598, Jan. 2023.
[5]. M. Lin, W. Luo, L. Li, Q. Han, and W. Lyu, “Design of the Threshold-Controllable Memristor Emulator Based on NDR Characteristics,” Micromachines, vol. 13, no. 6, p. 829, May 2022.
[6]. S. Wang et al., “Low power consumption photoelectric coupling perovskite memristor with adjustable threshold voltage,” Nanotechnology, vol. 32, no. 37, p. 375201, Jun. 2021.
[7]. A. S. Vidhyadharan and S. Vidhyadharan, “Memristor–CMOS hybrid ultra-low-power high-speed multivibrators,” Analog Integrated Circuits and Signal Processing, May 2021.
[8]. A. Ascoli, D. Baumann, R. Tetzlaff, L. O. Chua, and M. Hild, “Memristor‐enhanced humanoid robot control system – Part I: Theory behind the novel memcomputing paradigm,” International Journal of Circuit Theory and Applications, vol. 46, no. 1, pp. 155–183, Dec. 2017.
[9]. Q. Hong, H. Chen, J. Sun, and C. Wang, “Memristive Circuit Implementation of a Self-Repairing Network Based on Biological Astrocytes in Robot Application,” IEEE Transactions on Neural Networks and Learning Systems, vol. 33, no. 5, pp. 2106–2120, May 2022.
[10]. S. Zhu, L. Wang, Z. Dong, and S. Duan, “Convolution Kernel Operations on a Two-Dimensional Spin Memristor Cross Array,” Sensors, vol. 20, no. 21, p. 6229, Oct. 2020.
[11]. Q. Liu, L. Wang, J. Yang, Y. Wang, and S. Duan, “Fusion of Image Storage and Operation Based on Ag-Chalcogenide Memristor with Synaptic Plasticity,” Journal of Circuits, Systems and Computers, vol. 26, no. 10, p. 1750161, Mar. 2017.
[12]. C. Li et al., “Efficient and self-adaptive in-situ learning in multilayer memristor neural networks,” Nature Communications, vol. 9, no. 1, Jun. 2018.
[13]. H.-J. Liu, C.-L. Chen, X. Zhu, S.-Y. Sun, Q.-J. Li, and Z.-W. Li, “Memristor-based vector neural network architecture*,” Chinese Physics B, vol. 29, no. 2, p. 028502, Jan. 2020.
Cite this article
Gu,Z. (2023). Control method and analysis of potential application based on memristor. Applied and Computational Engineering,14,57-62.
Data availability
The datasets used and/or analyzed during the current study will be available from the authors upon reasonable request.
Disclaimer/Publisher's Note
The statements, opinions and data contained in all publications are solely those of the individual author(s) and contributor(s) and not of EWA Publishing and/or the editor(s). EWA Publishing and/or the editor(s) disclaim responsibility for any injury to people or property resulting from any ideas, methods, instructions or products referred to in the content.
About volume
Volume title: Proceedings of the 5th International Conference on Computing and Data Science
© 2024 by the author(s). Licensee EWA Publishing, Oxford, UK. This article is an open access article distributed under the terms and
conditions of the Creative Commons Attribution (CC BY) license. Authors who
publish this series agree to the following terms:
1. Authors retain copyright and grant the series right of first publication with the work simultaneously licensed under a Creative Commons
Attribution License that allows others to share the work with an acknowledgment of the work's authorship and initial publication in this
series.
2. Authors are able to enter into separate, additional contractual arrangements for the non-exclusive distribution of the series's published
version of the work (e.g., post it to an institutional repository or publish it in a book), with an acknowledgment of its initial
publication in this series.
3. Authors are permitted and encouraged to post their work online (e.g., in institutional repositories or on their website) prior to and
during the submission process, as it can lead to productive exchanges, as well as earlier and greater citation of published work (See
Open access policy for details).