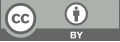
The application and challenges of artificial intelligence in speech recognition
- 1 University of Connecticut
* Author to whom correspondence should be addressed.
Abstract
This paper provides an overview of artificial intelligence (AI) and speech recognition technology, including its history, applications, challenges, and future prospects. AI-powered speech recognition technology has significantly improved over the years, and it is used in various applications, such as virtual assistants, voice-activated devices, and dictation software. The technology leverages machine learning algorithms that are trained on vast amounts of speech data to recognize and interpret human speech with accuracy levels that are comparable to those of humans. However, the technology still faces many challenges, such as speech variability and background noise, which make it challenging to develop speech recognition algorithms that can accurately recognize all types of speech. The article provides a comprehensive review of the technical aspects of automatic speech recognition, including the process involved, the algorithms used, and the challenges and opportunities for future research in this area. The paper also discusses the architecture of automatic speech recognition (ASR) systems and the main components that make up the system. The authors explain that ASR systems consist of three main components: the acoustic model, the language model, and the decoder. They also discuss the challenges that ASR systems face, such as speaker variability, noise, and limited vocabulary. Overall, this paper provides a detailed introduction to AI and speech recognition technology and its potential for various industries.
Keywords
speech recognition, ASR system, Mel-frequency cepstral coefficients (MFCCs), linear predictive coding (LPC), deep neural network (DNN)
[1]. Kayalibay B Jensen G van der Smagt P 2017 CNN-based segmentation of medical imaging data arXiv preprint arXiv:1701.03056.
[2]. Yu Q Wang J Jin Z et al. 2022 Pose-guided matching based on deep learning for assessing quality of action on rehabilitation training Biomedical Signal Processing and Contro 72 103323.
[3]. Hamarneh G Yang J McIntosh C et al 2005 3D live-wire-based semi-automatic segmentation of medical images Medical Imaging 2005: Image Processing SPIE 5747 pp 1597-1603.
[4]. Govindan K 2022 How artificial intelligence drives sustainable frugal innovation: A multitheoretical perspective IEEE Transactions on Engineering Management.
[5]. Arora S J and Rishi P S 2012 Automatic speech recognition: a review International Journal of Computer Applications 60.9.
[6]. Choudhary A and Ravi K 2012 Process speech recognition system using artificial intelligence technique International Journal of Soft Computing and Engineering (IJSCE) 2.
[7]. Benkerzaz S Youssef E and Abdeslam D 2019 A study on automatic speech recognition Journal of Information Technology Review 10.3 77-85.
[8]. Kumar, Deepak et al 2018 Skill squatting attacks on Amazon Alexa 27th {USENIX} Security Symposium ({USENIX} Security 18).
[9]. Velikovich L et al 2018 Semantic Lattice Processing in Contextual Automatic Speech Recognition for Google Assistant Interspeech.
[10]. MacArthur C A and Albert R C 2004 Dictation and speech recognition technology as test accommodations Exceptional Children 71.1 43-58.
Cite this article
Wang,B. (2023). The application and challenges of artificial intelligence in speech recognition. Applied and Computational Engineering,17,36-40.
Data availability
The datasets used and/or analyzed during the current study will be available from the authors upon reasonable request.
Disclaimer/Publisher's Note
The statements, opinions and data contained in all publications are solely those of the individual author(s) and contributor(s) and not of EWA Publishing and/or the editor(s). EWA Publishing and/or the editor(s) disclaim responsibility for any injury to people or property resulting from any ideas, methods, instructions or products referred to in the content.
About volume
Volume title: Proceedings of the 5th International Conference on Computing and Data Science
© 2024 by the author(s). Licensee EWA Publishing, Oxford, UK. This article is an open access article distributed under the terms and
conditions of the Creative Commons Attribution (CC BY) license. Authors who
publish this series agree to the following terms:
1. Authors retain copyright and grant the series right of first publication with the work simultaneously licensed under a Creative Commons
Attribution License that allows others to share the work with an acknowledgment of the work's authorship and initial publication in this
series.
2. Authors are able to enter into separate, additional contractual arrangements for the non-exclusive distribution of the series's published
version of the work (e.g., post it to an institutional repository or publish it in a book), with an acknowledgment of its initial
publication in this series.
3. Authors are permitted and encouraged to post their work online (e.g., in institutional repositories or on their website) prior to and
during the submission process, as it can lead to productive exchanges, as well as earlier and greater citation of published work (See
Open access policy for details).