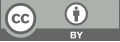
Overcoming data silos in healthcare: The potential of blockchain and federated learning on the Hedera platform
- 1 South China Normal University
* Author to whom correspondence should be addressed.
Abstract
The lack of access to extensive and varied datasets remains one of the major issues facing the field of machine learning, despite recent advancements. This is especially true in the healthcare sector, where it can be challenging to gather and use patient data for research because it is frequently compartmentalized across many healthcare providers. By enabling secure and privacy-preserving access to distributed data, blockchain technology, and federated learning have the potential to overcome these difficulties. In this article, we'll look at how federated learning and blockchain are used in the healthcare industry and talk about their benefits and drawbacks. We will also examine the Hedera platform, which makes use of blockchain technology and a new algorithm called Gossip Degree to provide a revolutionary method of federated learning. We will also go over the potential effects of federated learning on the healthcare sector and what it means for future research.
Keywords
federated learning, blockchain, Hedera
[1]. Zhang, Xiuxian, et al. "Hashgraph Based Federated Learning for Secure Data Sharing." Wireless and Satellite Systems: 11th EAI International Conference, WiSATS 2020, Nanjing, China, September 17-18, 2020, Proceedings, Part II. Springer International Publishing, 2021.
[2]. Hu, Chenghao, Jingyan Jiang, and Zhi Wang. "Decentralized federated learning: A segmented gossip approach." arXiv preprint arXiv:1908.07782 (2019).
[3]. Monrat A A, Schelén O, Andersson K. A survey of blockchain from the perspectives of applications, challenges, and opportunities. IEEE Access, 2019, 7: 117134-117151.
[4]. Zhou Q, Huang H, Zheng Z, et al. Solutions to scalability of blockchain: A survey. Ieee Access, 2020, 8: 16440-16455.
[5]. Berdik D, Otoum S, Schmidt N, et al. A survey on blockchain for information systems management and security. Information Processing & Management, 2021, 58(1): 102397.
[6]. Lu Y. The blockchain: State-of-the-art and research challenges. Journal of Industrial Information Integration, 2019, 15: 80-90.
[7]. Kholidy H A, Kamaludeen R. An Innovative Hashgraph-based Federated Learning Approach for Multi Domain 5G Network Protection//2022 IEEE Future Networks World Forum (FNWF). IEEE, 2022: 139-146.
[8]. Zhang X, Zhao L, Li J, et al. Hashgraph Based Federated Learning for Secure Data Sharing//Wireless and Satellite Systems: 11th EAI International Conference, WiSATS 2020, Nanjing, China, September 17-18, 2020, Proceedings, Part II. Springer International Publishing, 2021: 556-565.
[9]. Abid Haleem, Mohd Javaid, Ravi Pratap Singh, Rajiv Suman, Shanay Rab, Blockchain technology applications in healthcare: An overview, International Journal of Intelligent Networks, Volume 2, 2021, Pages 130-139, ISSN 2666-6030.
[10]. Shah K, Kanani S, Patel S, et al. Blockchain‐based object detection scheme using federated learning. Security and Privacy, 2023, 6(1): e276.
Cite this article
He,J. (2023). Overcoming data silos in healthcare: The potential of blockchain and federated learning on the Hedera platform. Applied and Computational Engineering,18,1-4.
Data availability
The datasets used and/or analyzed during the current study will be available from the authors upon reasonable request.
Disclaimer/Publisher's Note
The statements, opinions and data contained in all publications are solely those of the individual author(s) and contributor(s) and not of EWA Publishing and/or the editor(s). EWA Publishing and/or the editor(s) disclaim responsibility for any injury to people or property resulting from any ideas, methods, instructions or products referred to in the content.
About volume
Volume title: Proceedings of the 5th International Conference on Computing and Data Science
© 2024 by the author(s). Licensee EWA Publishing, Oxford, UK. This article is an open access article distributed under the terms and
conditions of the Creative Commons Attribution (CC BY) license. Authors who
publish this series agree to the following terms:
1. Authors retain copyright and grant the series right of first publication with the work simultaneously licensed under a Creative Commons
Attribution License that allows others to share the work with an acknowledgment of the work's authorship and initial publication in this
series.
2. Authors are able to enter into separate, additional contractual arrangements for the non-exclusive distribution of the series's published
version of the work (e.g., post it to an institutional repository or publish it in a book), with an acknowledgment of its initial
publication in this series.
3. Authors are permitted and encouraged to post their work online (e.g., in institutional repositories or on their website) prior to and
during the submission process, as it can lead to productive exchanges, as well as earlier and greater citation of published work (See
Open access policy for details).