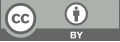
Applying multi-layer perceptron and ResNet for handwritten digits recognition
- 1 Zhongyuan University of Technology
* Author to whom correspondence should be addressed.
Abstract
The task of handwritten digit recognition is to recognizing the handwritten digits from pictures. Applying machine learning based models to automatically perform handwritten digit recognition task can significantly improve efficiency. This paper applies two machine learning based models, including multi-layer perceptron and residual neural network, for such a task. Firstly, this paper introduces the basic concept of the simple multi-layer perceptron model and then presents the structure of the residual neural network model. Subsequently, such two models are trained on the MNIST corpus, one of the classical dataset for the handwritten digit recognition task. The data pre-processing, like the splitting of training and test set, is described. Also, the processes of testing and training of the two models are presented. According to the experiments on the test set of MNIST, it is observed that the residual neural network can achieve better performance where the accuracy score is 99.240%, while the accuracy score of the multi-layer perceptron model is 97.260%.
Keywords
multi-layer perceptron, ResNet, handwritten digits recognition
[1]. Rahman M S, Khomh F, Hamidi A, Cheng J, Antoniol G, and Washizaki H 2023 Machine learning application development: practitioners’ insights Software Quality Journal p 1-55
[2]. Huang J C, Ko K M, Shu M H and Hsu B M 2020 Application and comparison of several machine learning algorithms and their integration models in regression problems Neural Computing and Applications vol 32 p 5461-69
[3]. Ali S, Shaukat Z, Azeem M, Sakhawat Z, Mahmood T and ur Rehman K 2019 An efficient and improved scheme for handwritten digit recognition based on convolutional neural network SN Applied Sciences vol 1 p 1-9
[4]. Lakshmi K L, Muthulakshmi P, Nithya A A, Jeyavathana R B, Usharani R, Das N S and Devi G N 2023 Recognition of emotions in speech using deep CNN and ResNet Soft Computing p 1-7
[5]. Jogin M, Madhulika M S, Divya G D, Meghana R K and Apoorva S 2018 Feature extraction using convolution neural networks (CNN) and deep learning In IEEE Int. Conf. on RTEICT p 2319-23
[6]. Ibrahim A O, Shamsuddin S M, Abraham A and Qasem S N 2019 Adaptive memetic method of multi-objective genetic evolutionary algorithm for backpropagation neural network Neural Computing and Applications vol 31 p 4945-62
[7]. Ghosh M M and Maghari A Y 2017 A comparative study on handwriting digit recognition using neural networks In Int. Conf. on Promising Electronic Technologies p 77-81
[8]. Jafar A and Lee M 2021 High-speed hyperparameter optimization for deep ResNet models in image recognition Cluster Computing p 1-9
[9]. Rössig A and Petkovic M 2021 Advances in verification of ReLU neural networks Journal of Global Optimization vol 81 p 109-52
[10]. Nayef B H, Abdullah S N H S, Sulaiman R and Alyasseri Z A A 2022 Optimized leaky ReLU for handwritten Arabic character recognition using convolution neural networks Multimedia Tools and Applications p 1-30
[11]. Sarkar S, Agrawal S, Baker T, Maddikunta P K and Gadekallu T R 2022 Catalysis of neural activation functions: adaptive feed-forward training for big data applications Applied Intelligence vol 52.12 p 13364-83
Cite this article
He,Z. (2023). Applying multi-layer perceptron and ResNet for handwritten digits recognition. Applied and Computational Engineering,18,16-22.
Data availability
The datasets used and/or analyzed during the current study will be available from the authors upon reasonable request.
Disclaimer/Publisher's Note
The statements, opinions and data contained in all publications are solely those of the individual author(s) and contributor(s) and not of EWA Publishing and/or the editor(s). EWA Publishing and/or the editor(s) disclaim responsibility for any injury to people or property resulting from any ideas, methods, instructions or products referred to in the content.
About volume
Volume title: Proceedings of the 5th International Conference on Computing and Data Science
© 2024 by the author(s). Licensee EWA Publishing, Oxford, UK. This article is an open access article distributed under the terms and
conditions of the Creative Commons Attribution (CC BY) license. Authors who
publish this series agree to the following terms:
1. Authors retain copyright and grant the series right of first publication with the work simultaneously licensed under a Creative Commons
Attribution License that allows others to share the work with an acknowledgment of the work's authorship and initial publication in this
series.
2. Authors are able to enter into separate, additional contractual arrangements for the non-exclusive distribution of the series's published
version of the work (e.g., post it to an institutional repository or publish it in a book), with an acknowledgment of its initial
publication in this series.
3. Authors are permitted and encouraged to post their work online (e.g., in institutional repositories or on their website) prior to and
during the submission process, as it can lead to productive exchanges, as well as earlier and greater citation of published work (See
Open access policy for details).