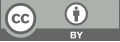
Stock price prediction based on the long short-term memory network
- 1 Green River College
* Author to whom correspondence should be addressed.
Abstract
Stock analysis is a challenging task that involves modelling complex and nonlinear dynamics of stock prices and volumes. Long Short-Term Memory (LSTM) is a type of recurrent neural network that can capture long-term dependencies and temporal patterns in time series data. In this paper, a stock analysis method based on LSTM is proposed that can predict future stock prices and transactions using historical data. Yfinance is used to obtain stock data of four technology companies (i.e. Apple, Google, Microsoft, and Amazon) and apply LSTM to extract features and forecast trends. Various techniques are also used such as moving average, correlation analysis, and risk assessment to evaluate the performance and risk of different stocks. When compare the method in this paper with other neural network models such as RNN and GRU, the result show that LSTM achieves better accuracy and stability in stock prediction. This paper demonstrates the effectiveness and applicability of LSTM method through experiments on real-world data sets.
Keywords
machine learning, stock price prediction, LSTM
[1]. Yu Y Si X Hu C et al. 2019 A review of recurrent neural networks: LSTM cells and network architectures Neural computation 31(7): 1235-1270
[2]. Staudemeyer R C Morris E R 2019 Understanding LSTM--a tutorial into long short-term memory recurrent neural networks arXiv preprint arXiv:1909.09586
[3]. Smagulova K James A P 2019 A survey on LSTM memristive neural network architectures and applications The European Physical Journal Special Topics 228(10): 2313-2324
[4]. Yin W Kann K Yu M 2017 et al. Comparative study of CNN and RNN for natural language processing arXiv preprint arXiv:1702.01923
[5]. Bordino I Kourtellis N Laptev N et al. 2014 Stock trade volume prediction with yahoo finance user browsing behavior 2014 IEEE 30th International Conference on Data Engineering pp 1168-1173
[6]. Lawrence A Ryans J Sun E et al. 2018 Earnings announcement promotions: A Yahoo Finance field experiment Journal of Accounting and Economics 66(2-3) 399-414
[7]. Xu S Y Berkely C U 2014 Stock price forecasting using information from Yahoo finance and Google trend UC Brekley
[8]. Ketkar N Ketkar N 2017 Introduction to keras Deep learning with python: a hands-on introduction 97-111
[9]. Qiu Y Yang Y Lin Z et al. 2020 Improved denoising autoencoder for maritime image denoising and semantic segmentation of USV China Communications 17(3): 46-57
[10]. Kayalibay B Jensen G van der Smagt P 2017 CNN-based segmentation of medical imaging data arXiv preprint arXiv:1701.03056
Cite this article
Zhang,S. (2023). Stock price prediction based on the long short-term memory network. Applied and Computational Engineering,18,28-32.
Data availability
The datasets used and/or analyzed during the current study will be available from the authors upon reasonable request.
Disclaimer/Publisher's Note
The statements, opinions and data contained in all publications are solely those of the individual author(s) and contributor(s) and not of EWA Publishing and/or the editor(s). EWA Publishing and/or the editor(s) disclaim responsibility for any injury to people or property resulting from any ideas, methods, instructions or products referred to in the content.
About volume
Volume title: Proceedings of the 5th International Conference on Computing and Data Science
© 2024 by the author(s). Licensee EWA Publishing, Oxford, UK. This article is an open access article distributed under the terms and
conditions of the Creative Commons Attribution (CC BY) license. Authors who
publish this series agree to the following terms:
1. Authors retain copyright and grant the series right of first publication with the work simultaneously licensed under a Creative Commons
Attribution License that allows others to share the work with an acknowledgment of the work's authorship and initial publication in this
series.
2. Authors are able to enter into separate, additional contractual arrangements for the non-exclusive distribution of the series's published
version of the work (e.g., post it to an institutional repository or publish it in a book), with an acknowledgment of its initial
publication in this series.
3. Authors are permitted and encouraged to post their work online (e.g., in institutional repositories or on their website) prior to and
during the submission process, as it can lead to productive exchanges, as well as earlier and greater citation of published work (See
Open access policy for details).