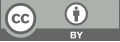
Learning rate adjustment and optimization of RepVGG network based on warmup strategy
- 1 Beijing University of Technology
* Author to whom correspondence should be addressed.
Abstract
Artificial neural networks have developed rapidly in recent years and play an important role in the academic field. In this paper, the RepVGG artificial neural network model is adjusted by the learning rate algorithm, so as to realize the optimization of the model including but not limited to accuracy. The main optimization strategy is to add the warmup strategy based on the learning rate algorithm of the original model so that the model can obtain good prior information on the data early in the training process, so as to converge quickly in the later training. Through a series of tests and simulations, the RepVGG-A0 model improves the Top1 accuracy by about 2.6% to 68.56% and the Top5 accuracy by about 0.38% to 94.32% on imagesetter dataset within 25 training epochs. The precision and recall are improved to 68.43% and 68.63%, respectively.
Keywords
RepVGG, Learning Rate, Warmup Strategy, Optimization
[1]. Great Again,” 2021 IEEE/CVF Conference on Computer Vision and Pattern Recognition (CVPR), Jun. 2021.
[2]. W. Li, X. Luo, Z. Meng, and J. Chen, “Facial expression recognition combined with improved RepVGG⁃A0 network and relabeling,” Oct. 2022.
[3]. J. Sirignano and K. Spiliopoulos, “Stochastic Gradient Descent in Continuous Time,” SSRN Electronic Journal, 2017.
[4]. G. Ioannou, T. Tagaris, and A. Stafylopatis, “AdaLip: An Adaptive Learning Rate Method per Layer for Stochastic Optimization,” Neural Processing Letters, Jan. 2023.
[5]. K. Zeng, J. Liu, Z. Jiang, and D. Xu, “A Scaling Transition Method from SGDM to SGD with 2ExpLR Strategy,” Applied Sciences, vol. 12, no. 23, p. 12023, Nov. 2022.
[6]. P. Izmailov, D. Podoprikhin, T. Garipov, D. Vetrov, and A. Wilson, “Averaging Weights Leads to Wider Optima and Better Generalization.”
[7]. P. Goyal et al., “Accurate, Large Minibatch SGD: Training ImageNet in 1 Hour.” 2020.
[8]. K. Zeng, J. Liu, Z. Jiang, and D. Xu, “A Scaling Transition Method from SGDM to SGD with 2ExpLR Strategy,” Applied Sciences, vol. 12, no. 23, p. 12023, Nov. 2022.K. He, X. Zhang, S. Ren, and J. Sun, “Deep Residual Learning for Image Recognition,” Dec. 10, 2015.
[9]. D. Shulman, “Optimization Methods in Deep Learning: A Comprehensive Overview,” Feb. 2023.
[10]. J. Howard and S. Gugger, “Fastai: A Layered API for Deep Learning,” Information, vol. 11, no. 2, p. 108, Feb. 2020, doi: https://doi.org/10.3390/info11020108.
[11]. K. He, X. Zhang, S. Ren, and J. Sun, “Deep Residual Learning for Image Recognition,” Dec. 10, 2015. C. Zhang, M. Yao, W. Chen, S. Zhang, D. Chen, and Y. Wu, “Gradient Descent Optimization in Deep Learning Model Training Based on Multistage and Method Combination Strategy,” Security and Communication Networks, vol. 2021, pp. 1–15, Jul. 2021.
[12]. X. Ding, X. Zhang, N. Ma, J. Han, G. Ding, and J. Sun, “RepVGG: Making VGG-style ConvNets
Cite this article
Lin,Y. (2023). Learning rate adjustment and optimization of RepVGG network based on warmup strategy. Applied and Computational Engineering,19,28-36.
Data availability
The datasets used and/or analyzed during the current study will be available from the authors upon reasonable request.
Disclaimer/Publisher's Note
The statements, opinions and data contained in all publications are solely those of the individual author(s) and contributor(s) and not of EWA Publishing and/or the editor(s). EWA Publishing and/or the editor(s) disclaim responsibility for any injury to people or property resulting from any ideas, methods, instructions or products referred to in the content.
About volume
Volume title: Proceedings of the 5th International Conference on Computing and Data Science
© 2024 by the author(s). Licensee EWA Publishing, Oxford, UK. This article is an open access article distributed under the terms and
conditions of the Creative Commons Attribution (CC BY) license. Authors who
publish this series agree to the following terms:
1. Authors retain copyright and grant the series right of first publication with the work simultaneously licensed under a Creative Commons
Attribution License that allows others to share the work with an acknowledgment of the work's authorship and initial publication in this
series.
2. Authors are able to enter into separate, additional contractual arrangements for the non-exclusive distribution of the series's published
version of the work (e.g., post it to an institutional repository or publish it in a book), with an acknowledgment of its initial
publication in this series.
3. Authors are permitted and encouraged to post their work online (e.g., in institutional repositories or on their website) prior to and
during the submission process, as it can lead to productive exchanges, as well as earlier and greater citation of published work (See
Open access policy for details).