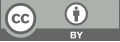
A simplified U-net model for Covid-19 CT image segmentation
- 1 Beijing University of Technology
* Author to whom correspondence should be addressed.
Abstract
The ongoing COVID-19 pandemic has highlighted the importance of accurate and efficient medical image analysis to aid in the diagnosis and treatment of patients. In particular, the segmentation of COVID-19 medical images has become a critical task to identify regions of interest, such as the infected lung areas, and to track disease progression. Traditional image segmentation methods have been widely used in medical image analysis. However, these methods are often challenged by the complex and diverse nature of COVID-19 images, as well as the limited availability of data. In this paper, we propose a simplified version of the U-Net that eliminates redundant crop operations. This simplification reduces computational complexity and memory usage, and enables the model to learn from larger input images, resulting in better performance. We evaluate the performance of our simplified U-Net model on a public COVID-19 dataset and demonstrate that our model achieves state-of-the-art results while using fewer computational resources.
Keywords
Covid-19, image segmentation, U-net, deep learning, CT image
[1]. Yang L, Liu S, Liu J, et al. COVID-19: immunopathogenesis and Immunotherapeutics. Signal Transduct. Target. Ther., 2020.
[2]. Zhou T, Canu S, Ruan S. Automatic COVID-19 CT segmentation using U-Net integrated spatial and channel attention mechanism. Int. J. Imaging Syst. Technol., 2021.
[3]. Shi F, Wang J, Shi J, et al. Review of Artificial Intelligence Techniques in Imaging Data Acquisition, Segmentation, and Diagnosis for COVID-19. IEEE Rev. Biomed. Eng., 2021.
[4]. Rendon-Gonzalez E, Ponomaryov V. Automatic Lung nodule segmentation and classification in CT images based on SVM. 2016 9th Int. Kharkiv Symp. Phys. Eng. Microw. Millim. Submillimeter Waves MSMW, 2016.
[5]. Liu C, Zhao R, Pang M. A fully automatic segmentation algorithm for CT lung images based on random forest. Med. Phys., 2020.
[6]. Rajinikanth V, Dey N, Raj ANJ, et al. Harmony-Search and Otsu based System for Coronavirus Disease (COVID-19) Detection using Lung CT Scan Images. 2020.
[7]. Shanks CB, Hingle MD, Parks CA, et al. The COVID-19 Pandemic: A Watershed Moment to Strengthen Food Security Across the US Food System. Am. J. Public Health, 2020.
[8]. Faragallah OS, El-Hoseny HM, El-Sayed HS. Efficient COVID-19 super pixel segmentation algorithm using MCFO-based SLIC. J. Ambient Intell. Humaniz. Comput., 2022.
[9]. Xu X, Xu S, Jin L, et al. Characteristic analysis of Otsu threshold and its applications. Pattern Recognit. Lett., 2011.
[10]. Beucher S. The Watershed Transformation Applied to Image Segmentation. Scanning Microsc., 1992.
[11]. SLIC Superpixels Compared to State-of-the-art Superpixel Methods. IEEE Trans. Pattern Anal. Mach. Intell., 2012.
[12]. Yang F, Sun Q, Jin H, et al. Superpixel Segmentation With Fully Convolutional Networks. 2020.
[13]. Ronneberger O, Fischer P, Brox T. U-Net: Convolutional Networks for Biomedical Image Segmentation. Med. Image Comput. Comput.-Assist. Interv. – MICCAI 2015, 2015.
[14]. Yamashita R, Nishio M, Do RKG, et al. Convolutional neural networks: an overview and application in radiology. Insights Imaging, 2018.
[15]. Naseri H, Mehrdad V. Novel CNN with investigation on accuracy by modifying stride, padding, kernel size and filter numbers. Multimed. Tools Appl., 2023.
[16]. Kandel I, Castelli M. The effect of batch size on the generalizability of the convolutional neural networks on a histopathology dataset. ICT Express, 2020.
[17]. Rahman MA, Wang Y. Optimizing Intersection-Over-Union in Deep Neural Networks for Image Segmentation. Adv. Vis. Comput., 2016.
[18]. Luc P, Neverova N, Couprie C, et al. Predicting Deeper Into the Future of Semantic Segmentation. 2017.
[19]. Deep‐learning‐based, computer‐aided classifier developed with a small dataset of clinical images surpasses board‐certified dermatologists in skin tumour diagnosis | British Journal of Dermatology | Oxford Academic.
[20]. Ng H-W, Nguyen VD, Vonikakis V, et al. Deep Learning for Emotion Recognition on Small Datasets using Transfer Learning. Proc. 2015 ACM Int. Conf. Multimodal Interact., 2015.
[21]. Hagos MT, Kant S. Transfer Learning based Detection of Diabetic Retinopathy from Small Dataset. 2019.
[22]. Preprocessing-Free Gear Fault Diagnosis Using Small Datasets With Deep Convolutional Neural Network-Based Transfer Learning | IEEE Journals & Magazine | IEEE Xplore.
Cite this article
Zhan,H. (2023). A simplified U-net model for Covid-19 CT image segmentation. Applied and Computational Engineering,19,75-83.
Data availability
The datasets used and/or analyzed during the current study will be available from the authors upon reasonable request.
Disclaimer/Publisher's Note
The statements, opinions and data contained in all publications are solely those of the individual author(s) and contributor(s) and not of EWA Publishing and/or the editor(s). EWA Publishing and/or the editor(s) disclaim responsibility for any injury to people or property resulting from any ideas, methods, instructions or products referred to in the content.
About volume
Volume title: Proceedings of the 5th International Conference on Computing and Data Science
© 2024 by the author(s). Licensee EWA Publishing, Oxford, UK. This article is an open access article distributed under the terms and
conditions of the Creative Commons Attribution (CC BY) license. Authors who
publish this series agree to the following terms:
1. Authors retain copyright and grant the series right of first publication with the work simultaneously licensed under a Creative Commons
Attribution License that allows others to share the work with an acknowledgment of the work's authorship and initial publication in this
series.
2. Authors are able to enter into separate, additional contractual arrangements for the non-exclusive distribution of the series's published
version of the work (e.g., post it to an institutional repository or publish it in a book), with an acknowledgment of its initial
publication in this series.
3. Authors are permitted and encouraged to post their work online (e.g., in institutional repositories or on their website) prior to and
during the submission process, as it can lead to productive exchanges, as well as earlier and greater citation of published work (See
Open access policy for details).