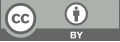
A machine learning based ‘wearable depression, anxiety and somatisation monitoring IOT system (WDASMS)’ for the prevention of suicides
- 1 Department of Computer Science and Engineering, Periyar Maniammai Institute of Science & Technology, Thanjavur, Tamilnadu, India.
- 2 Department of Computer Science and Engineering, Periyar Maniammai Institute of Science & Technology, Thanjavur, Tamilnadu, India.
- 3 Department of Computer Science and Engineering, Periyar Maniammai Institute of Science & Technology, Thanjavur, Tamilnadu, India.
- 4 Department of Computer Science and Engineering, Periyar Maniammai Institute of Science & Technology, Thanjavur, Tamilnadu, India.
* Author to whom correspondence should be addressed.
Abstract
Tamil Nadu reportedly is the alternate loftiest rates of pupil self-murder in India. According to report from the National Crime Records Bureau (NCRB) for 2020, about 46 people failed each day by self-murder in the state; further than two of these victims each day were scholars. The government of India’s domestic seminaries for meritorious pastoral children have reportedly witnessed 49 self-murders in just five times and half of the children who killed themselves on the premises were Dalits and Adivasis, Educationalists point at the pressure mounted on scholars for marks and competitive examinations as one of the main reasons. Utmost seminaries have councillors but going to the council has a smirch about it still. Domestic seminaries where these cases are passing more need to be covered more. Children dying by self-murder due to academic pressure are unheard of in other countries. Utmost of the A huge number of enquiries revealed depression and anxiety to be implicit risk factors for self-murder. Heart health is good among students between the ages of 15 and 25. A few physiological parameters are altered by anxiety and depression. Hence, current pulsation is the symptom for psychiatric diseases. Clinical trials have demonstrated that mental reasons, most frequently anxiety, depression, and somatoform illnesses, are present in cases with pulsation. Psychiatric problems like anxiety and somatization are linked to palpitation. A study found that individuals with high levels of both depression and anxiety are 54.77 times more likely to commit suicide, which is a significant increase above individuals with either high levels of anxiety or depression (2.46). (26.32).. Naturally students studying +2 and preparing for high marks are in under depression and it's dragged for two times. When the examinations are nearing and the results are nearing, the combined depression and anxiety factor increases the threat for committing self-murders for84.5. Hence, a system with literacy and decision making medium is needed to constantly monitor the students to take prompt conduct. A wearable IoT device transmits with scholars Id, the pulsation position constantly to the fellow knot. Logistic Retrogression machine literacy algorithm is used for decision making grounded on the probability of circumstance. The authorities are informed in their widgets and prompt conduct can be taken. This system will be more applicable to the boarding seminaries, where the children are far down from their parents and the number of self-murders are high particularly among +2 grade. This system can be used for colourful communities, where the working culture gives depression and anxiety.
Keywords
self murder, palpitation, depression, anxiety, IOT, machine learning
[1]. Mullick T, Radovic A, Shaaban S, Doryab A. Predicting Depression in Adolescents Using Mobile and Wearable Sensors: Multimodal Machine Learning-Based Exploratory Study. JMIR Form Res. 2022 Jun 24;6(6):e35807. doi: 10.2196/35807. PMID: 35749157; PMCID: PMC9270714
[2]. Minkowski L, Mai KV, Gurve D. Feature Extraction to Identify Depression and Anxiety Based on EEG. Annu Int Conf IEEE Eng Med Biol Soc. 2021 Nov;2021:6322-6325. doi: 10.1109/EMBC46164.2021.9630821.PMID: 34892559
[3]. A. Ahmed, R. Sultana, M. T. R. Ullas, M. Begom, M. M. I. Rahi and M. A. Alam, "A Machine Learning Approach to detect Depression and Anxiety using Supervised Learning," 2020 IEEE Asia-Pacific Conference on Computer Science and Data Engineering (CSDE), Gold Coast, Australia, 2020, pp. 1-6, doi:10.1109/CSDE50874.2020.9411642.
[4]. S. Fukuda, Y. Matsuda, Y. Tani, Y. Arakawa and K. Yasumoto, "Predicting Depression and Anxiety Mood by Wrist-Worn Sleep Sensor," 2020 IEEE International Conference on Pervasive Computing and Communications Workshops (PerCom Workshops), Austin, TX, USA, 2020
[5]. D. M. Shukla, K. Sharma and S. Gupta, "Identifying Depression in a Person Using Speech Signals by Extracting Energy and Statistical Features," 2020 IEEE International Students' Conference on Electrical,Electronics and Computer Science (SCEECS), Bhopal, India, 2020, pp. 1-4, doi:10.1109/SCEECS48394.2020.60.
[6]. S. Jaiswal, S. Song and M. Valstar, "Automatic prediction of Depression and Anxiety from behaviour and personality attributes," 2019 8th International Conference on Affective Computing and Intelligent Interaction (ACII), Cambridge, UK, 2019, pp. 1-7, doi: 10.1109/ACII.2019.8925456.
[7]. S. J. K. Jagadeesh Kumar, P. Parthasarathi , Mofreh A. Hogo, Mehedi Masud, Jehad F. Al-Amri and Mohamed Abouhawwash, “Breast Cancer Detection Using Breastnet-18 Augmentation with Fine Tuned Vgg-16”, Intelligent Automation & Soft Computing, Vol. 36, No.2, pp. 2363–2378, 2023.
[8]. Aledavood, T., Torous, J., Triana Hoyos, A.M. et al. Smartphone-Based Tracking of Sleep in Depression, Anxiety, and Psychotic Disorders. Curr Psychiatry Rep 21, 49 (2019).
[9]. A. Tiwari, R. Cassani, S. Narayanan and T. H. Falk, "A Comparative Study of Stress and Anxiety Estimation in Ecological Settings Using a Smart-shirt and a Smart-bracelet," 2019 41st Annual International Conference of the IEEE Engineering in Medicine and Biology Society (EMBC), Berlin, Germany, 2019, pp. 2213-2216, doi: 10.1109/EMBC.2019.8857890.
[10]. P. Sundaravadivel, P. Salvatore and P. Indic, "M-SID: An IoT-based Edge-intelligent Framework for Suicidal Ideation Detection," 2020 IEEE 6th World Forum on Internet of Things (WF-IoT), New Orleans, LA, USA, 2020, pp. 1-6, doi: 10.1109/WF-IoT48130.2020.9221279.
Cite this article
Narayanan,A.E.;Vijayadharshini,D.;Ruthrabala,P.;Ajay,A.S. (2023). A machine learning based ‘wearable depression, anxiety and somatisation monitoring IOT system (WDASMS)’ for the prevention of suicides. Applied and Computational Engineering,20,19-25.
Data availability
The datasets used and/or analyzed during the current study will be available from the authors upon reasonable request.
Disclaimer/Publisher's Note
The statements, opinions and data contained in all publications are solely those of the individual author(s) and contributor(s) and not of EWA Publishing and/or the editor(s). EWA Publishing and/or the editor(s) disclaim responsibility for any injury to people or property resulting from any ideas, methods, instructions or products referred to in the content.
About volume
Volume title: Proceedings of the 5th International Conference on Computing and Data Science
© 2024 by the author(s). Licensee EWA Publishing, Oxford, UK. This article is an open access article distributed under the terms and
conditions of the Creative Commons Attribution (CC BY) license. Authors who
publish this series agree to the following terms:
1. Authors retain copyright and grant the series right of first publication with the work simultaneously licensed under a Creative Commons
Attribution License that allows others to share the work with an acknowledgment of the work's authorship and initial publication in this
series.
2. Authors are able to enter into separate, additional contractual arrangements for the non-exclusive distribution of the series's published
version of the work (e.g., post it to an institutional repository or publish it in a book), with an acknowledgment of its initial
publication in this series.
3. Authors are permitted and encouraged to post their work online (e.g., in institutional repositories or on their website) prior to and
during the submission process, as it can lead to productive exchanges, as well as earlier and greater citation of published work (See
Open access policy for details).