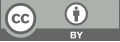
An overview of algorithms for discovering new categories of problems based on unlabeled data
- 1 The Dalian University of Technology
* Author to whom correspondence should be addressed.
Abstract
In traditional machine learning, supervised and semi-supervised learning is designed to be used in a closed-world setting where the training data is fixed and does not change over time. Unfortunately, these methods still require a large number of labels for the categories to be categorized, which is expensive and impractical. A new category discovery algorithm is designed so that it can discover new categories while classifying and recognizing labeled images. In this case, the machine can automatically identify new categories without manual marking of image feature categories, which can greatly reduce the cost of image classification. Kai Han et al. named this problem a new category discovery problem and proposed that deep clustering can be used to solve it well. This paper focuses on the comparison of two commonly used robust baselines in the new category discovery and proposes that adding a post-processing model can better improve the accuracy of the model result. This paper applied the relaxed contrast learning method to the Ranking Statistics, and the accuracy of CIFAR-100 is improved by 6%.
Keywords
NCD, GCD, representation learning, RankStats, UNO
[1]. Cao, Kaidi, Maria Brbic, and Jure Leskovec. "Open-world semi-supervised learning." arXiv preprint arXiv:2102.03526 (2021).
[2]. Han, Kai, Andrea Vedaldi, and Andrew Zisserman. "Learning to discover novel visual categories via deep transfer clustering." Proceedings of the IEEE/CVF International Conference on Computer Vision. 2019.
[3]. Han, Kai, et al. "Automatically discovering and learning new visual categories with ranking statistics." arXiv preprint arXiv:2002.05714 (2020).
[4]. Fini, Enrico, et al. "A unified objective for novel class discovery." Proceedings of the IEEE/CVF International Conference on Computer Vision. 2021.
[5]. Vaze, Sagar, et al. "Generalized category discovery." Proceedings of the IEEE/CVF Conference on Computer Vision and Pattern Recognition. 2022.
[6]. Yuval Netzer, Tao Wang, Adam Coates, Alessandro Bissacco, Bo Wu, and Andrew Y. Ng. Reading digits in natural images with unsupervised feature learning. In NeurIPS Workshop on Deep Learning and Unsupervised Feature Learning, 2011.
[7]. Antti Tarvainen and Harri Valpola. Mean teachers are better role models: Weight-averaged consistency targets improve semi-supervised deep learning results. In NeurIPS, 2017.
[8]. Sinno Jialin Pan and Qiang Yang. A survey on transfer learning. IEEE Transactions on Knowledge and Data Engineering, 2010.
[9]. Jia Deng, Wei Dong, Richard Socher, Li-Jia Li, Kai Li, and Li Fei-Fei. Imagenet: A large-scale hierarchical image database. In CVPR, 2009.
[10]. He, Kaiming, et al. "Deep residual learning for image recognition." Proceedings of the IEEE conference on computer vision and pattern recognition. 2016.
[11]. Kim, Sungyeon, et al. "Embedding transfer with label relaxation for improved metric learning." Proceedings of the IEEE/CVF conference on computer vision and pattern recognition. 2021.
[12]. Alex Krizhevsky. Learning multiple layers of features from tiny images. Technical report, 2009.
Cite this article
Tong,C. (2023). An overview of algorithms for discovering new categories of problems based on unlabeled data. Applied and Computational Engineering,20,49-54.
Data availability
The datasets used and/or analyzed during the current study will be available from the authors upon reasonable request.
Disclaimer/Publisher's Note
The statements, opinions and data contained in all publications are solely those of the individual author(s) and contributor(s) and not of EWA Publishing and/or the editor(s). EWA Publishing and/or the editor(s) disclaim responsibility for any injury to people or property resulting from any ideas, methods, instructions or products referred to in the content.
About volume
Volume title: Proceedings of the 5th International Conference on Computing and Data Science
© 2024 by the author(s). Licensee EWA Publishing, Oxford, UK. This article is an open access article distributed under the terms and
conditions of the Creative Commons Attribution (CC BY) license. Authors who
publish this series agree to the following terms:
1. Authors retain copyright and grant the series right of first publication with the work simultaneously licensed under a Creative Commons
Attribution License that allows others to share the work with an acknowledgment of the work's authorship and initial publication in this
series.
2. Authors are able to enter into separate, additional contractual arrangements for the non-exclusive distribution of the series's published
version of the work (e.g., post it to an institutional repository or publish it in a book), with an acknowledgment of its initial
publication in this series.
3. Authors are permitted and encouraged to post their work online (e.g., in institutional repositories or on their website) prior to and
during the submission process, as it can lead to productive exchanges, as well as earlier and greater citation of published work (See
Open access policy for details).