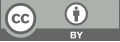
Brain tumor diagnoses based on VGG-16 and MobileNet
- 1 Tongji University
* Author to whom correspondence should be addressed.
Abstract
Brain tumor, recognized as one of the most formidable and aggressive diseases globally, continues to pose significant challenges for medical practitioners in clinical diagnosis and treatment. Addressing the burden on doctors and addressing resource limitations in certain hospitals necessitates the development of efficient and dependable alternative approaches. Convolutional Neural Networks (CNNs), renowned for their prowess in image recognition, hold immense potential in addressing this pressing issue. Leveraging transfer learning, the capabilities of established models such as VGG-16 and MobileNet can be harnessed to construct superior models within a comparatively abbreviated timeframe. This research paper aims to construct and evaluate VGG-16 and MobileNet-based models, employing transfer learning, to explore the applicability of these two classical models in the context of brain tumor diagnosis. The ultimate goal is to assist doctors and hospitals in alleviating the challenges associated with brain tumor diagnoses. The results demonstrated the effectiveness of brain tumor recognition based on CNNs.
Keywords
brain tumor, CNN, transfer learning, VGG-16, MobileNet
[1]. Kermany DS et al 2018 Identifying medical diagnoses and treatable diseases by image-based deep learning Cell 172(5) pp 1122–1131.
[2]. Kaggle 2020 Brain Tumor Classification (MRI) https://www.kaggle.com/datasets/sartajbhuvaji/brain-tumor-classification-mri.
[3]. Simonyan K Zisserman A 2014 Very deep convolutional networks for large-scale image recognition arXiv preprint arXiv:1409.1556.
[4]. Howard A G Zhu M Chen B et al 2017 Mobilenets: Efficient convolutional neural networks for mobile vision applications arXiv preprint arXiv:1704.04861.
[5]. Zhou X Li Y Liang W 2020 CNN-RNN based intelligent recommendation for online medical pre-diagnosis support IEEE/ACM Transactions on Computational Biology and Bioinformatics18(3) pp 912-921.
[6]. Yu Q et al 2019 Semantic segmentation of intracranial hemorrhages in head CT scans 2019 IEEE 10th International Conference on Software Engineering and Service Science (ICSESS).
[7]. Jiang X Yang S Wang F et al 2021 OrbitNet: A new CNN model for automatic fault diagnostics of turbomachines Applied Soft Computing 110: 107702.
[8]. Sun Y Xue B Zhang M et al 2019 Completely automated CNN architecture design based on blocks IEEE transactions on neural networks and learning systems 31(4) pp 1242-1254.
[9]. Abadi M 2016 TensorFlow: learning functions at scale Proceedings of the 21st ACM SIGPLAN International Conference on Functional Programming 2016 pp 1-1.
[10]. Pang B Nijkamp E Wu Y N 2020 Deep learning with tensorflow: A review Journal of Educational and Behavioral Statistics 45(2) pp 227-248.
Cite this article
Liu,Y. (2023). Brain tumor diagnoses based on VGG-16 and MobileNet. Applied and Computational Engineering,22,28-34.
Data availability
The datasets used and/or analyzed during the current study will be available from the authors upon reasonable request.
Disclaimer/Publisher's Note
The statements, opinions and data contained in all publications are solely those of the individual author(s) and contributor(s) and not of EWA Publishing and/or the editor(s). EWA Publishing and/or the editor(s) disclaim responsibility for any injury to people or property resulting from any ideas, methods, instructions or products referred to in the content.
About volume
Volume title: Proceedings of the 5th International Conference on Computing and Data Science
© 2024 by the author(s). Licensee EWA Publishing, Oxford, UK. This article is an open access article distributed under the terms and
conditions of the Creative Commons Attribution (CC BY) license. Authors who
publish this series agree to the following terms:
1. Authors retain copyright and grant the series right of first publication with the work simultaneously licensed under a Creative Commons
Attribution License that allows others to share the work with an acknowledgment of the work's authorship and initial publication in this
series.
2. Authors are able to enter into separate, additional contractual arrangements for the non-exclusive distribution of the series's published
version of the work (e.g., post it to an institutional repository or publish it in a book), with an acknowledgment of its initial
publication in this series.
3. Authors are permitted and encouraged to post their work online (e.g., in institutional repositories or on their website) prior to and
during the submission process, as it can lead to productive exchanges, as well as earlier and greater citation of published work (See
Open access policy for details).