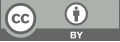
Traditional Banking Evolution in China Amidst the Fintech Revolution
- 1 Faculty of Humanities and Social Sciences, University of Newcastle upon Tyne, NE1 7RU, UK
* Author to whom correspondence should be addressed.
Abstract
This paper aims to investigate how the evolution of fintech could impact the traditional banking industry in China by reviewing the existing research. It provides a brief introduction to Chinese banks, outlining their structures, and functions against the background of the development in the Chinese financial market. The paper also discusses how fintech could potentially interact with Chinese banks and the possible changes in bank financing systems, clearing systems, and credit rating systems in light of the fintech revolution. The final section of the report provides a summary of the accomplishments of fintech in the banking industry. It also explains the reasons behind the fintech revolution in the banking sector and suggests practical solutions for traditional Chinese banks to integrate fintech into their regular operations. This integration can help improve the efficiency of financing, clearing, and credit rating systems by utilizing fintech techniques already in use such as P2P lending, blockchain, and artificial intelligence (AI).
Keywords
banking, fintech, P2P lending, blockchain, artificial intelligence (AI)
[1]. Bayraktar, N., & Wang, Y. (2008). Banking sector openness and economic growth. Margin: The Journal of Applied Economic Research, 2(2), 145-175.
[2]. Fu, J. and Mishra, M. (2022) ‘Fintech in the time of COVID−19: Technological adoption during crises’, Journal of Financial Intermediation, 50, p. 100945. Available at: https://doi.org/10.1016/j.jfi.2021.100945.
[3]. Chen, T.-H. (2020) ‘Do you know your customer? Bank risk assessment based on machine learning’, Applied Soft Computing, 86, p. 105779. Available at: https://doi.org/10.1016/j.asoc.2019.105779.
[4]. Lyonnet, V., & Stern, L. H. (2022). Venture capital (mis) allocation in the age of AI. Fisher College of Business Working Paper, (2022-03), 002.
[5]. Cornelli, G., Frost, J., Gambacorta, L., Rau, P. R., Wardrop, R., & Ziegler, T. (2020). Fintech and big tech credit: a new database.
[6]. Chuen, D. L. K., & Teo, E. G. (2015). Emergence of FinTech and the LASIC principles. The Journal of Financial Perspectives: Fintech, 3(3), 24-37.
[7]. From FinTech, F. S. I. (2017). Supervisory and Regulatory Issues that Merit Authorities’ Attention. FSB. Available at: https://www. fsb. org/wp-content/uploads, 270617.
[8]. Liu, J., Li, X. and Wang, S. (2020) ‘What have we learnt from 10 years of fintech research? a scientometric analysis’, Technological Forecasting and Social Change, 155, p. 120022. Available at: https://doi.org/10.1016/j.techfore.2020.120022.
[9]. Jagtiani, J., & Lemieux, C. (2017). Fintech lending: Financial inclusion, risk pricing, and alternative information. Risk Pricing, and Alternative Information (December 26, 2017).
[10]. Turing, A. (2004) ‘Computing Machinery and Intelligence (1950)’, in Turing, A., The Essential Turing. Oxford University Press. Available at: https://doi.org/10.1093/oso/9780198250791.003.0017.
[11]. Kelly, B., & Xiu, D. (2023). Financial machine learning. Foundations and Trends® in Finance, 13(3-4), 205-363.
[12]. Fethi, M.D. and Pasiouras, F. (2009) ‘Assessing Bank Performance with Operational Research and Artificial Intelligence Techniques: A Survey’, SSRN Electronic Journal [Preprint]. Available at: https://doi.org/10.2139/ssrn.1350544.
[13]. Xiang, D., Zhang, Y. and Worthington, A.C. (2021) ‘Determinants of the Use of Fintech Finance Among Chinese Small and Medium-Sized Enterprises’, IEEE Transactions on Engineering Management, 68(6), pp. 1590–1604. Available at: https://doi.org/10.1109/TEM.2020.2989136.
[14]. Tsai, W.-T. et al. (2020) ‘Blockchain systems for trade clearing’, The Journal of Risk Finance, 21(5), pp. 469–492. Available at: https://doi.org/10.1108/JRF-02-2017-0022.
[15]. Wang, X. et al. (2020) ‘Making the right business decision: Forecasting the binary NPD strategy in Chinese automotive industry with machine learning methods’, Technological Forecasting and Social Change, 155, p. 120032. Available at: https://doi.org/10.1016/j.techfore.2020.120032.
[16]. Gan, L., Wang, H. and Yang, Z. (2020) ‘Machine learning solutions to challenges in finance: An application to the pricing of financial products’, Technological Forecasting and Social Change, 153, p. 119928. Available at: https://doi.org/10.1016/j.techfore.2020.119928.
[17]. Tang, L., Cai, F. and Ouyang, Y. (2019) ‘Applying a nonparametric random forest algorithm to assess the credit risk of the energy industry in China’, Technological Forecasting and Social Change, 144, pp. 563–572. Available at: https://doi.org/10.1016/j.techfore.2018.03.007.
[18]. Gogas, P., Papadimitriou, T. and Agrapetidou, A. (2014) ‘Forecasting bank credit ratings’, The Journal of Risk Finance, 15(2), pp. 195–209. Available at: https://doi.org/10.1108/JRF-11-2013-0076.
[19]. Öğüt, H. et al. (2012) ‘Prediction of bank financial strength ratings: The case of Turkey’, Economic Modelling, 29(3), pp. 632–640. Available at: https://doi.org/10.1016/j.econmod.2012.01.010.
[20]. Kumar, K. and Bhattacharya, S. (2006) ‘Artificial neural network vs linear discriminant analysis in credit ratings forecast: A comparative study of prediction performances’, Review of Accounting and Finance, 5(3), pp. 216–227. Available at: https://doi.org/10.1108/14757700610686426.
[21]. Li, J.-P. et al. (2020) ‘Machine learning and credit ratings prediction in the age of fourth industrial revolution’, Technological Forecasting and Social Change, 161, p. 120309. Available at: https://doi.org/10.1016/j.techfore.2020.120309.
Cite this article
Li,S. (2024). Traditional Banking Evolution in China Amidst the Fintech Revolution. Advances in Economics, Management and Political Sciences,75,228-233.
Data availability
The datasets used and/or analyzed during the current study will be available from the authors upon reasonable request.
Disclaimer/Publisher's Note
The statements, opinions and data contained in all publications are solely those of the individual author(s) and contributor(s) and not of EWA Publishing and/or the editor(s). EWA Publishing and/or the editor(s) disclaim responsibility for any injury to people or property resulting from any ideas, methods, instructions or products referred to in the content.
About volume
Volume title: Proceedings of the 3rd International Conference on Business and Policy Studies
© 2024 by the author(s). Licensee EWA Publishing, Oxford, UK. This article is an open access article distributed under the terms and
conditions of the Creative Commons Attribution (CC BY) license. Authors who
publish this series agree to the following terms:
1. Authors retain copyright and grant the series right of first publication with the work simultaneously licensed under a Creative Commons
Attribution License that allows others to share the work with an acknowledgment of the work's authorship and initial publication in this
series.
2. Authors are able to enter into separate, additional contractual arrangements for the non-exclusive distribution of the series's published
version of the work (e.g., post it to an institutional repository or publish it in a book), with an acknowledgment of its initial
publication in this series.
3. Authors are permitted and encouraged to post their work online (e.g., in institutional repositories or on their website) prior to and
during the submission process, as it can lead to productive exchanges, as well as earlier and greater citation of published work (See
Open access policy for details).